Pandemic-Aware Electric Load Forecasting: A Multitask Bidirectional LSTM/CNN Model
IEEE International Conference on Communications (ICC)(2022)
摘要
COVID-19 pandemic has brought major uncertainty in load forecasting. Enforcing and relaxing lockdown rules, infection numbers, and the changing habits of people are the main causes of this uncertainty. Electric load forecasting maintains the balance between electric supply and demand. It also assists electric utilities in pricing their services, planning, and managing their infrastructure. This paper proposes two pandemic-aware load forecasting models (i) a city-level model, applied on the cities of Ottawa and Toronto, predicting hourly load using weather and pandemic-related features including population mobility and the number of daily COVID-19 infections, and (ii) a second open-source model forecasting quarter-hourly residential-level loads using weather and population mobility features for the city of Pune in India. Both models utilize multitask learning to jointly learn and predict future electric loads. The quarter-hourly model uses Bi-directional Long Short-Term Memory (LSTM) to learn from COVID's specific features, and a Convolutional Neural Network (CNN) to learn from the historical load data before the pandemic. The multitask nature of the model allows for incorporating multiple datasets with different numbers of features. The residential-level multitask model allowed for learning from long-term data before COVID-19 using weather features, short-term load data, and the mobility data. Multitask learning has also enabled the use of two datasets with different numbers of features due to the lack of mobility data pre-COVID.
更多查看译文
关键词
Bidirectional LSTM,CNN,COVID-19,demand response,eMARC,mobility,multitask learning
AI 理解论文
溯源树
样例
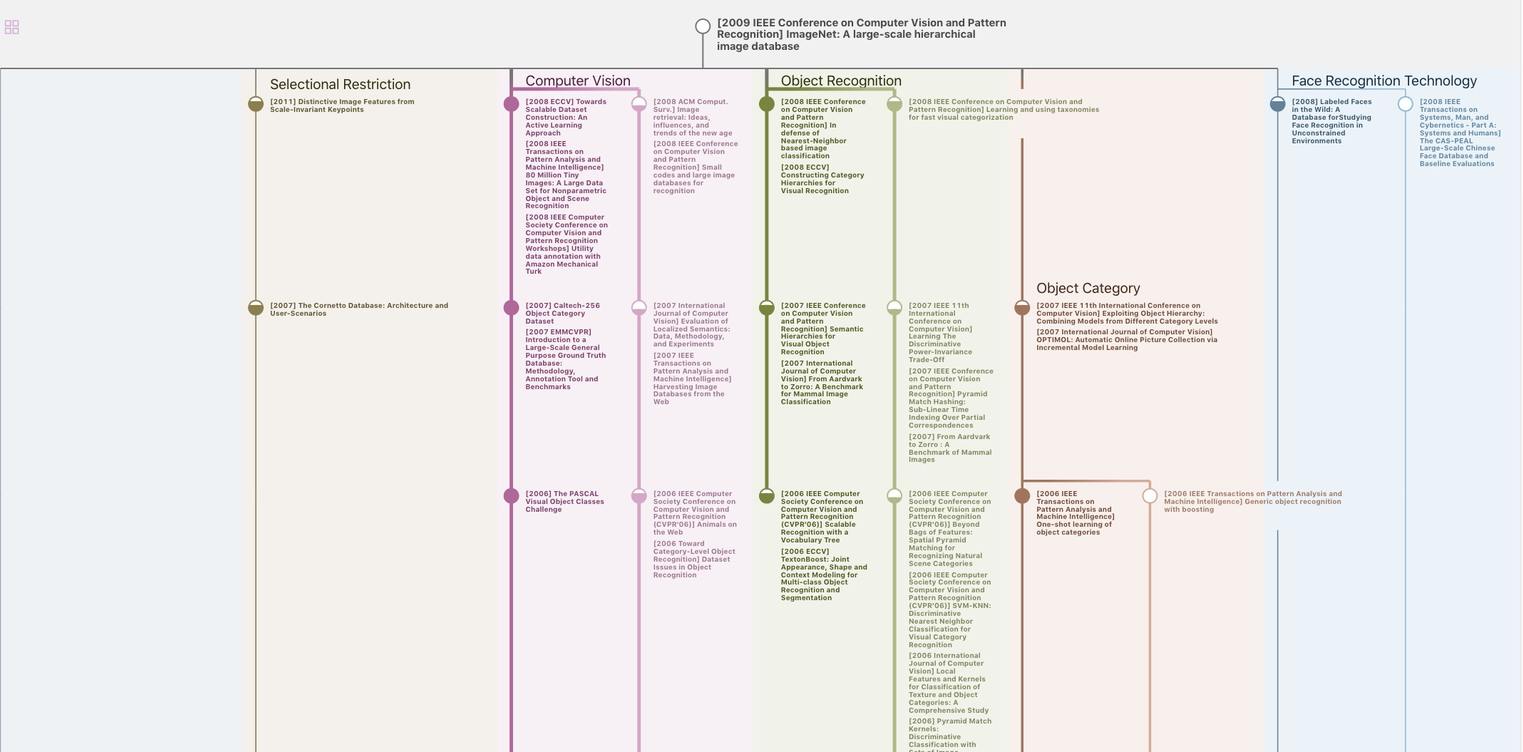
生成溯源树,研究论文发展脉络
Chat Paper
正在生成论文摘要