SIoT Selection, Clustering, and Routing for Federated Learning with Privacy-Preservation
IEEE International Conference on Communications (ICC)(2022)
摘要
With the advances in Social Internet of Things (SIoT) and Federated learning (FL), smart devices are now able to cooperatively and locally perform learning tasks to protect sensitive data by Differential Privacy (DP). On the other hand, Hierarchical FL (HFL) clusters SIoTs into multiple local training groups to reduce communication overheads by local aggregation. In this paper, we explore SIoT Training Group Construction (STGC) for HFL to minimize the total SIoT computation, communication and hiring costs, and the privacy cost for exploiting DP. We prove that STGC is NP-hard and inapproximable within any factor unless P = NP. Then, we design an algorithm with the ideas of Coverage Efficiency Indicator, Data Balance-aware Dual Adjustment, and Privacy-Aware Rerouting to choose and cluster SIoTs and to determine the aggregator for local training and SIoT routing in each cluster. Simulation results manifest that the proposed algorithm outperforms state-of-the-arts regarding the total cost, model accuracy, and convergence time.
更多查看译文
关键词
SIoT selection,Federated learning,Privacy-preservation,Social Internet of Things,sensitive data,Differential Privacy,Hierarchical FL clusters SIoTs,HFL,multiple local training groups,communication overheads,local aggregation,SIoT Training Group Construction,STGC,total SIoT computation,privacy cost,NP-hard,Data Balance-aware Dual Adjustment,Privacy-Aware Rerouting,cluster SIoTs,SIoT routing
AI 理解论文
溯源树
样例
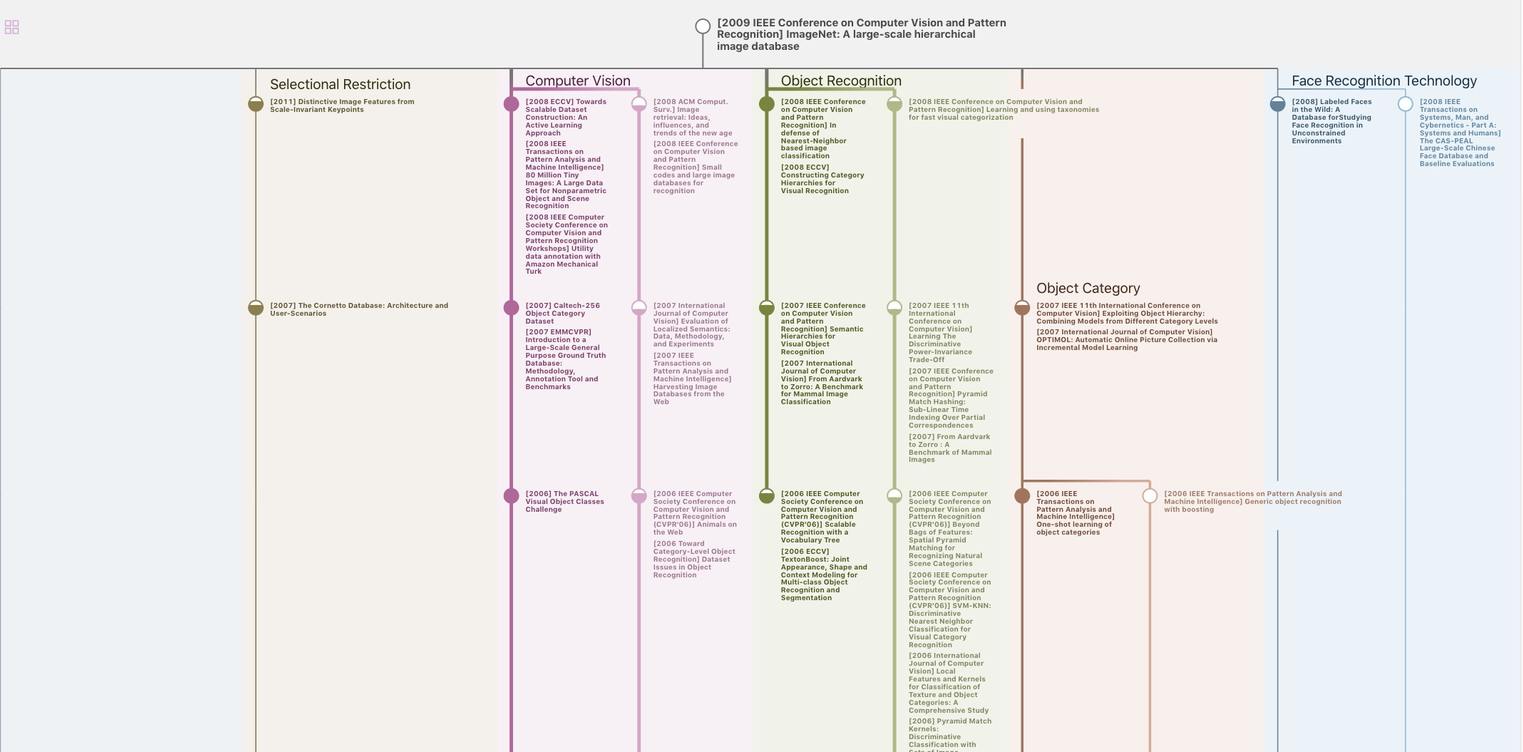
生成溯源树,研究论文发展脉络
Chat Paper
正在生成论文摘要