Client Discovery and Data Exchange in Edge-based Federated Learning via Named Data Networking
IEEE International Conference on Communications (ICC)(2022)
摘要
Federated learning (FL) is gaining momentum as a prominent solution to perform training procedures without the need to move sensitive end-user data to a centralized third party server. In FL, models are locally trained at distributed end-devices, acting as clients, and only model updates are transferred from the clients to the aggregator, which is in charge of global model aggregation. Although FL can ensure better privacy preservation than centralized machine learning (ML), it exhibits still some concerns. First, clients need to be properly discovered and selected to ensure that highly accurate models are built. Second, huge models may still require to be exchanged from the aggregator to all the selected clients, incurring a not negligible network footprint. To tackle such issues, in this paper, we propose a framework built upon in-network caching, multicast and name-based data delivery, natively provided by the Named Data Networking (NDN) paradigm, in order to support client discovery and aggregator-clients data exchange. Benefits of the proposal are showcased when compared to a conventional application-layer solution.
更多查看译文
关键词
Federated Learning,Edge Artificial Intelligence,Named Data Networking,Information Centric Networking,In-network caching
AI 理解论文
溯源树
样例
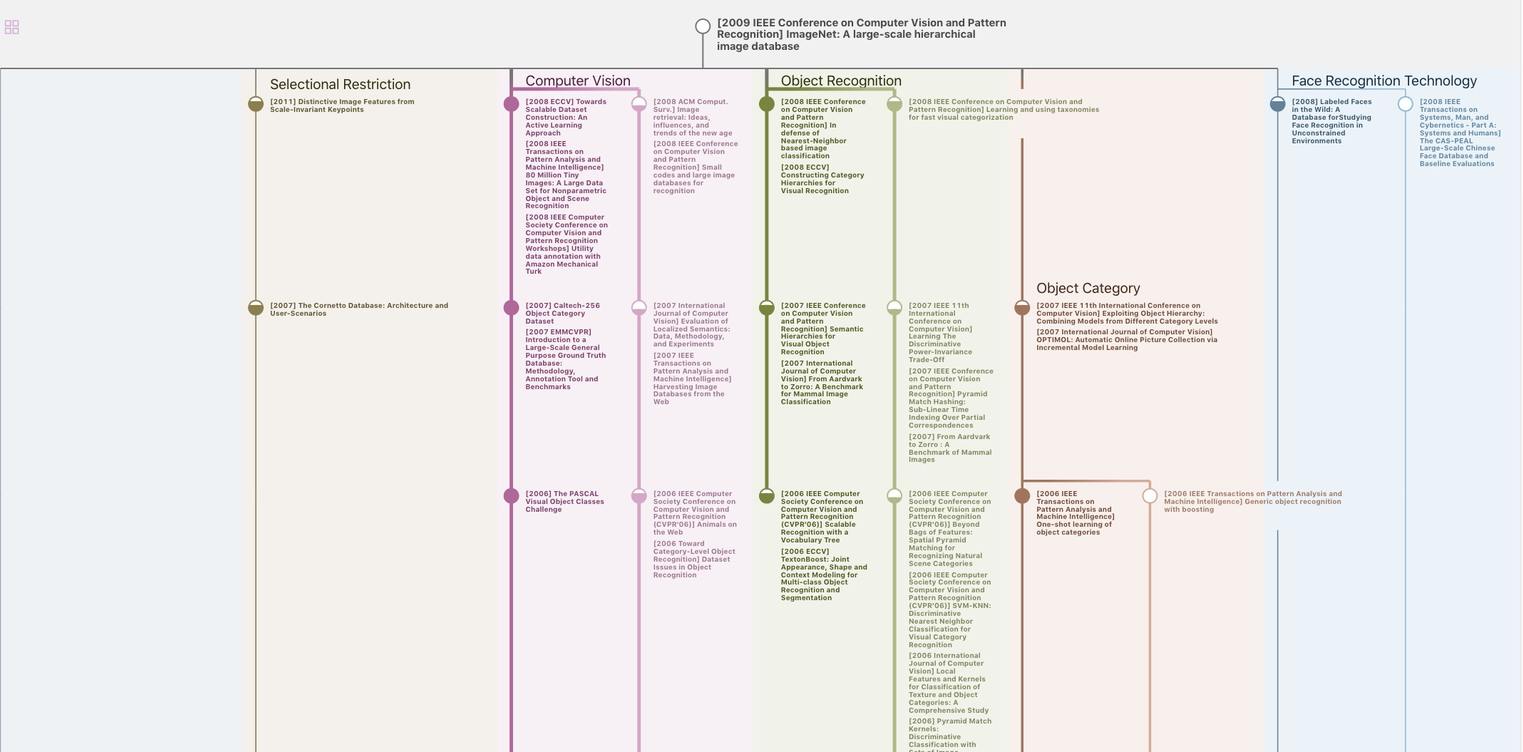
生成溯源树,研究论文发展脉络
Chat Paper
正在生成论文摘要