Ran$Net: An Anti-Ransomware Methodology based on Cache Monitoring and Deep Learning
ACM Great Lakes Symposium on VLSI (GLSVLSI)(2022)
摘要
Ransomware has become a serious threat in the cyberspace. Existing software pattern-based malware detectors are specific for certain ransomware and may not capture new variants. Recognizing a common essential behavior of ransomware - employing local cryptographic software for malicious encryption and therefore leaving footprints on the victim machine's caches, this work proposes an anti-ransomware methodology, Ran$Net, based on hardware activities. It consists of a passive cache monitor to log suspicious cache activities, and a follow-on non-profiled deep learning analysis strategy to retrieve the secret cryptographic key from the timing traces generated by the monitor. We implement the first of its kind tool to combat an open-source ransomware and successfully recover the secret key.
更多查看译文
AI 理解论文
溯源树
样例
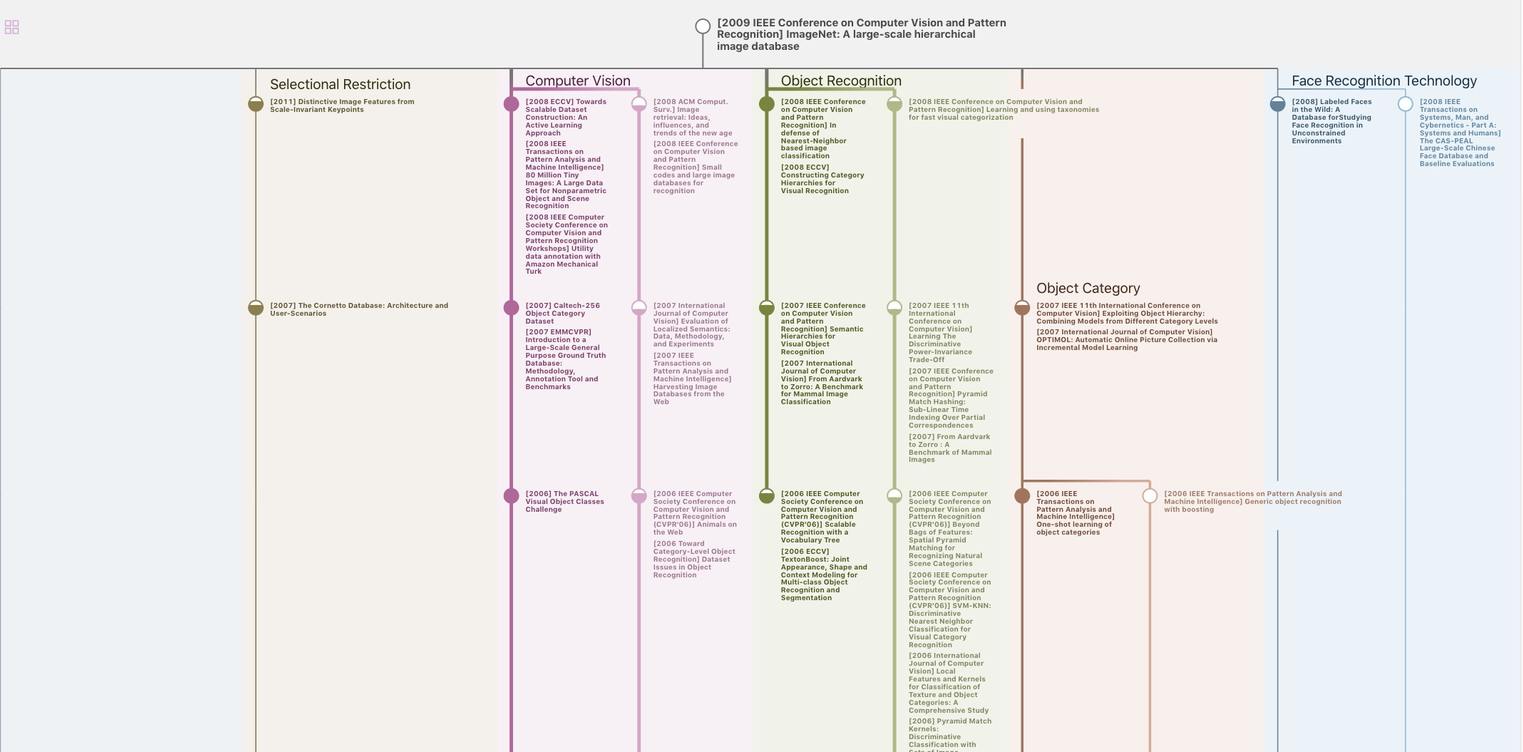
生成溯源树,研究论文发展脉络
Chat Paper
正在生成论文摘要