Multi-Scale Reinforcement Learning Strategy for Object Detection
IEEE International Conference on Acoustics, Speech, and Signal Processing (ICASSP)(2022)
摘要
Feature Pyramid Network (FPN) has become a common detection paradigm by improving multi-scale features with strong semantics. However, most FPN-based methods typically treat each feature map equally and sum the loss without distinction, which might lead to suboptimal overall performance. In this paper, we propose a Multi-scale Reinforcement Learning Strategy (MRLS) for balanced multi-scale training First, we design Dynamic Feature Fusion (DFF) to dynamically magnify the impact of more important feature maps in FPN. Second, we introduce Compensatory Scale Training (CST) to enhance the supervision of the undertraining scale. We regard the whole detector as a reinforcement learning system while the state bases on multi-scale loss. And we develop the corresponding action, reward, and policy. Compared with adding more rich model architectures, MRLS would not add any extra modules and computational burdens on the baselines. Experiments on MS COCO and PASCAL VOC benchmark demonstrate that our method significantly improves the performance of commonly used object detectors.
更多查看译文
关键词
Object detection,Reinforcement learning,Multi-scale,Balanced training
AI 理解论文
溯源树
样例
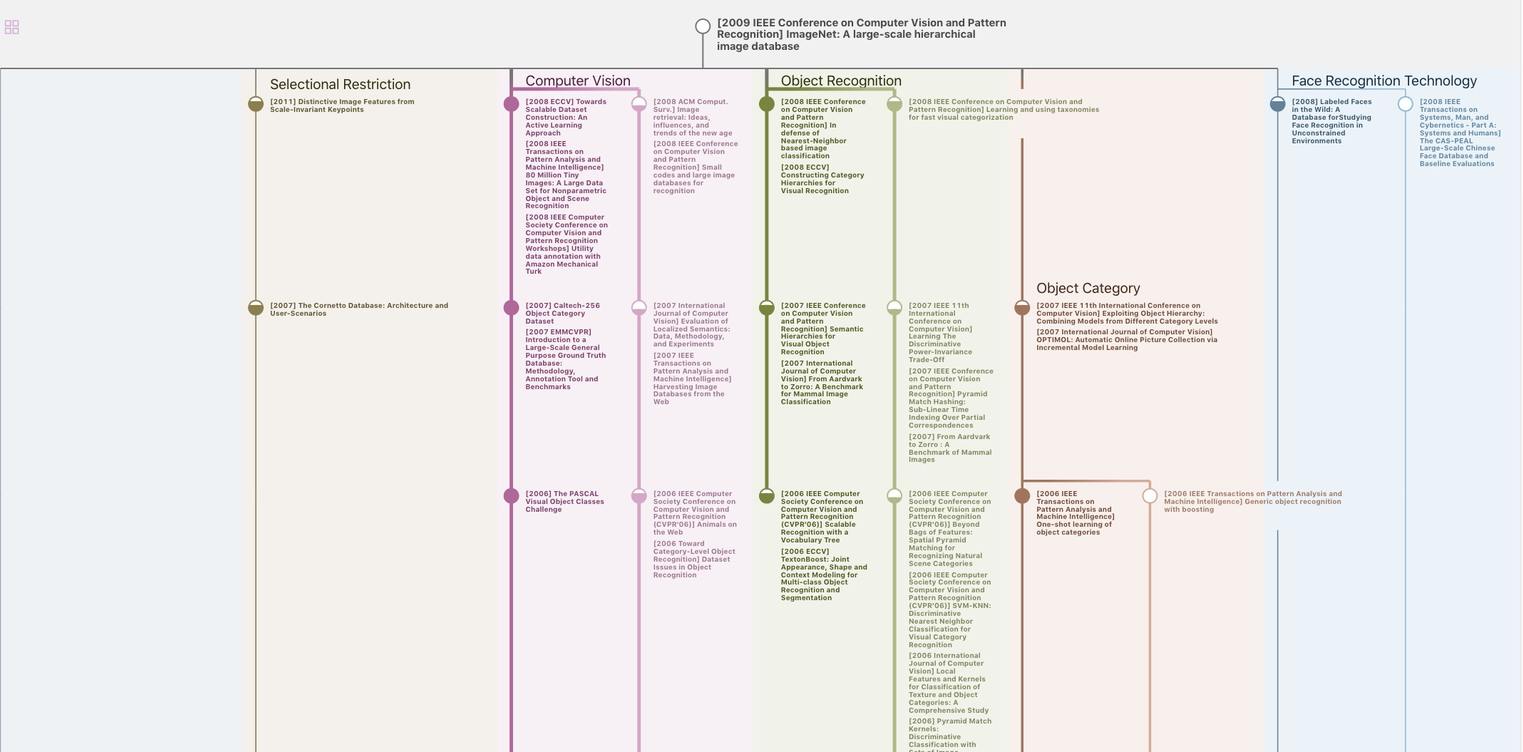
生成溯源树,研究论文发展脉络
Chat Paper
正在生成论文摘要