Progressive Teacher-Student Training Framework for Music Tagging
IEEE International Conference on Acoustics, Speech, and Signal Processing (ICASSP)(2022)
摘要
Music tagging is the task of predicting multiple tags of a music excerpt, and plays an important role in modern music recommendation systems. To obtain superior performance, recent approaches of music tagging focus on developing sophisticated models or exploiting additional multi-modal information. However, none of them deal with the problem of label noise during the training process despite of its ubiquitous presence. In this paper, we propose a progressive two-stage teacher-student training framework to prevent the music tagging model from overfitting label noise. Experimental results suggest that the proposed method surpasses conventional label-noise-robust methods and exhibits scalability across different tagging models. Moreover, detailed analyses demonstrate that the two teachers in the framework gradually improve student model's generalization performance and effectively avoid the impairment from label noise.
更多查看译文
关键词
Music tagging,label noise,teacher student training,deep learning
AI 理解论文
溯源树
样例
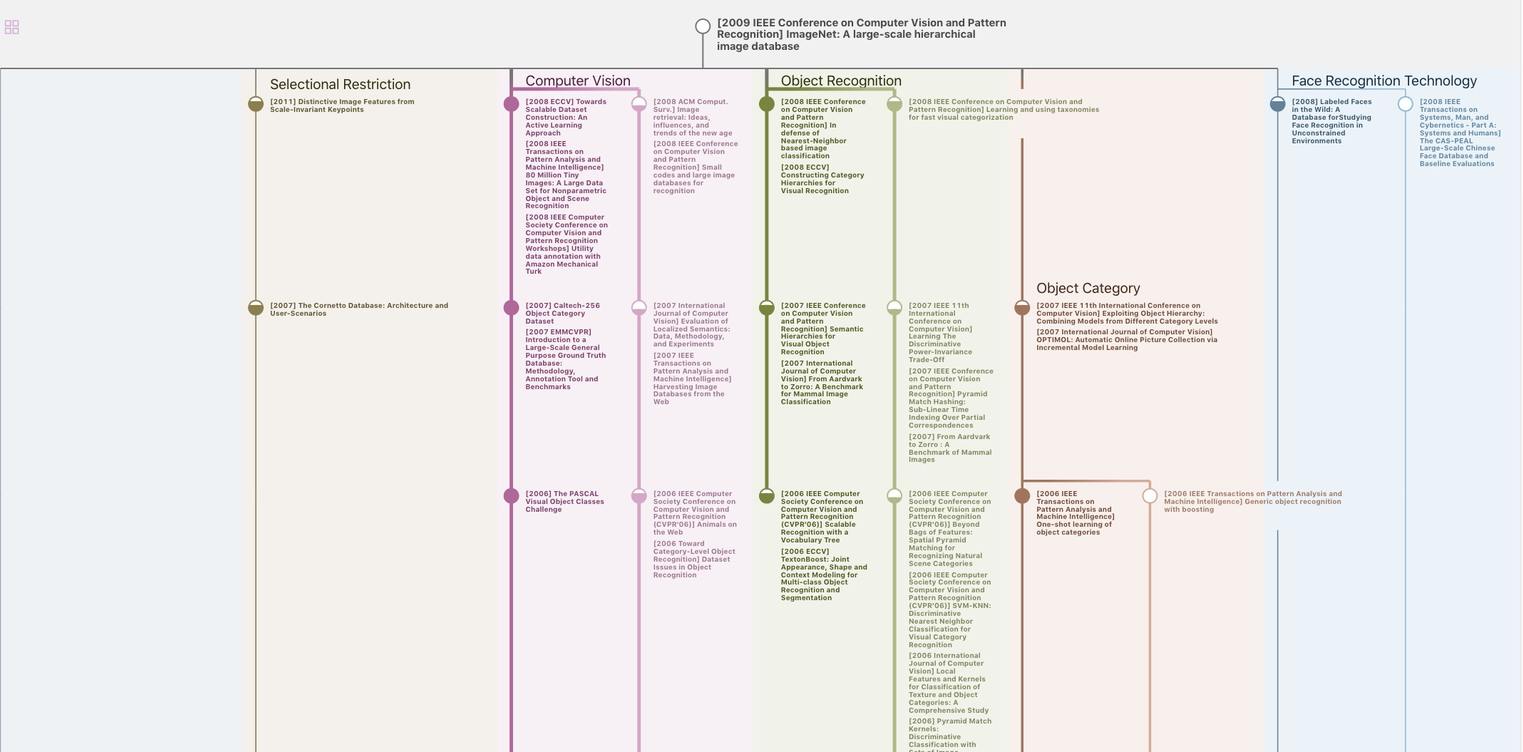
生成溯源树,研究论文发展脉络
Chat Paper
正在生成论文摘要