Connecting Targets via Latent Topics And Contrastive Learning: A Unified Framework For Robust Zero-Shot and Few-Shot Stance Detection
IEEE International Conference on Acoustics, Speech, and Signal Processing (ICASSP)(2022)
摘要
Zero-shot and few-shot stance detection (ZFSD) aims to automatically identify the users' stance toward a wide range of continuously emerging targets without or with limited labeled data. Previous works on in-target and cross-target stance detection typically focus on extremely limited targets, which is not applicable to the zero-shot and few-shot scenarios. Additionally, existing ZFSD models are not good at modeling the relationship between seen and unseen targets. In this paper, we propose a unified end-to-end framework with a discrete latent topic variable that implicitly establishes the connections between targets. Moreover, we apply supervised contrastive learning to enhance the generalization ability of the model. Comprehensive experiments on the ZFSD task verify the effectiveness and superiority of our proposed method.
更多查看译文
关键词
Stance Detection,Latent Topic Variable,Contrastive Learning,BERT
AI 理解论文
溯源树
样例
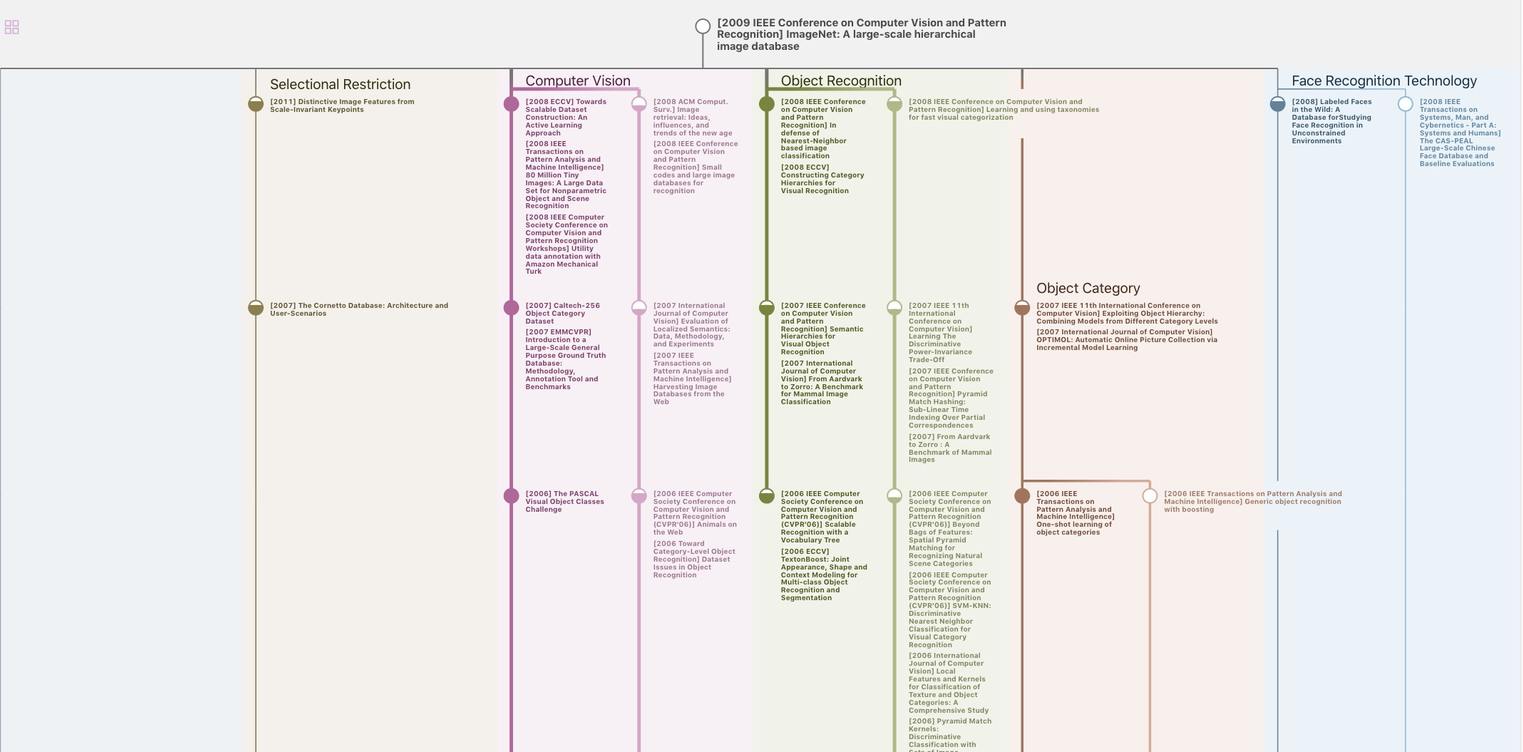
生成溯源树,研究论文发展脉络
Chat Paper
正在生成论文摘要