Generalized Face Anti-Spoofing via Cross-Adversarial Disentanglement with Mixing Augmentation
IEEE International Conference on Acoustics, Speech, and Signal Processing (ICASSP)(2022)
摘要
Conventional face anti-spoofing methods might be poorly generalized to unseen data distributions. Thus, we improve the generalization of spoof detection from the multi-domain feature disentanglement. Specially, a two-branch convolutional network is proposed to separate spoof-specific features and domain-specific features from face images explicitly. The spoof-specific features are further used for live vs. spoof classification. To minimize correlation among these two features, we present a cross-adversarial training scheme, which requires each branch to act as adversarial supervision for the other branch. To further exploit the subdomains from source data, a mixing augmentation approach is proposed based on mixing domain-specific feature statistics from different instances. It ensures more abundant domain discrepancy and facilitates the disentanglement process. The proposed approach shows promising generalization capacity in several public face anti-spoofing datasets.
更多查看译文
关键词
Face anti-spoofing,feature disentanglement,adversarial learning,data augmentation
AI 理解论文
溯源树
样例
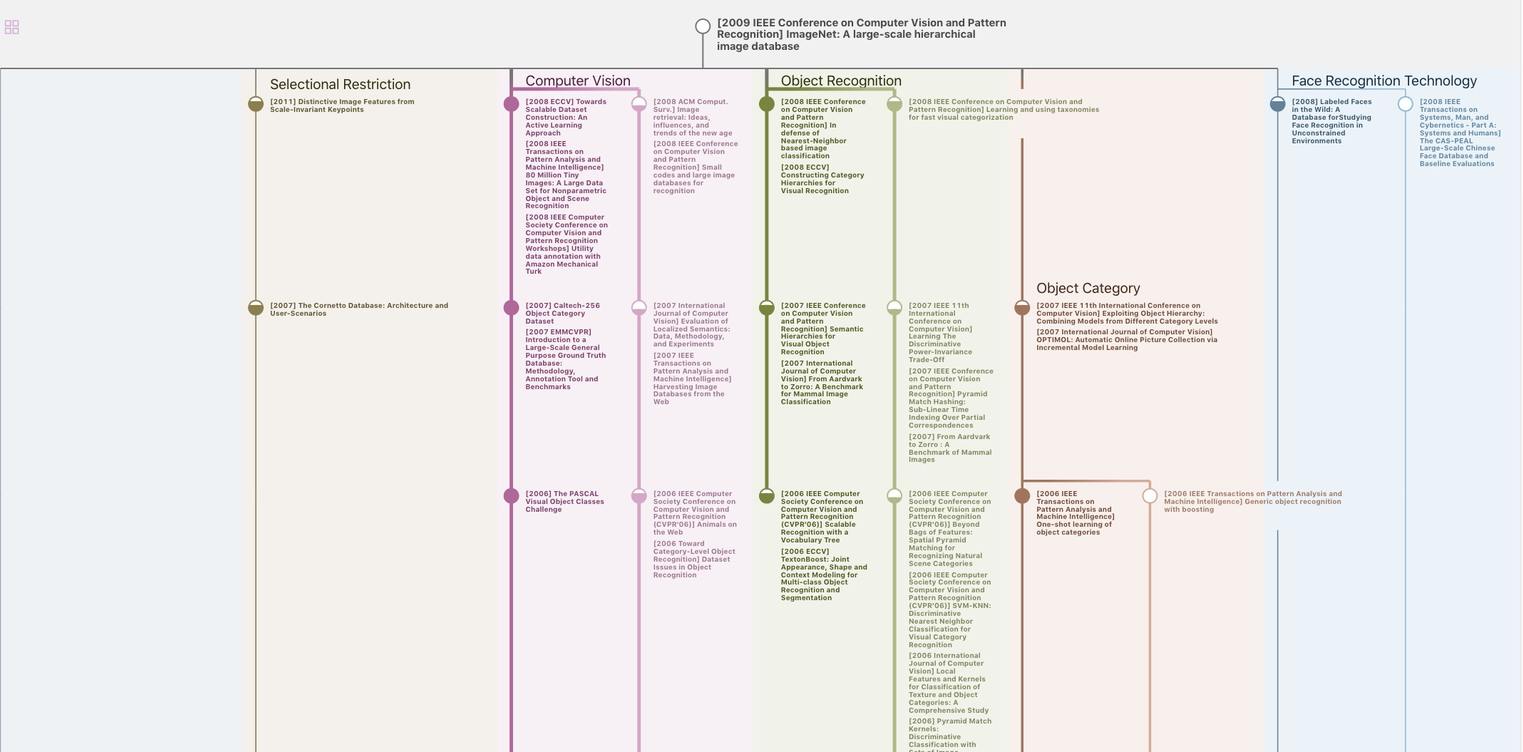
生成溯源树,研究论文发展脉络
Chat Paper
正在生成论文摘要