Sampling Set Selection for Graph Signals under Arbitrary Signal Priors
IEEE International Conference on Acoustics, Speech, and Signal Processing (ICASSP)(2022)
摘要
We propose a sampling set selection method for graph signals under arbitrary signal priors. Most approaches of graph signal sampling assume that signals are bandlimited. However, in practical situations, there exist many full-band graph signals like piecewise smooth/constant signals. Our sampling set selection method allows for arbitrary graph signal models as long as they are linear. This can be derived from a generalized sampling framework. In contrast to existing works, we focus on the direct sum condition between sampling and reconstruction subspaces where the direct sum condition plays a key role for the best possible recovery of sampled signals. We also design a fast sampling set selection algorithm based on the proposed method with the Neumann series approximation. In sampling and recovery experiments, we validate the effectiveness of the proposed method for several graph signal models.
更多查看译文
关键词
Generalized sampling,subspace prior,smoothness prior,stochastic prior,direct sum condition
AI 理解论文
溯源树
样例
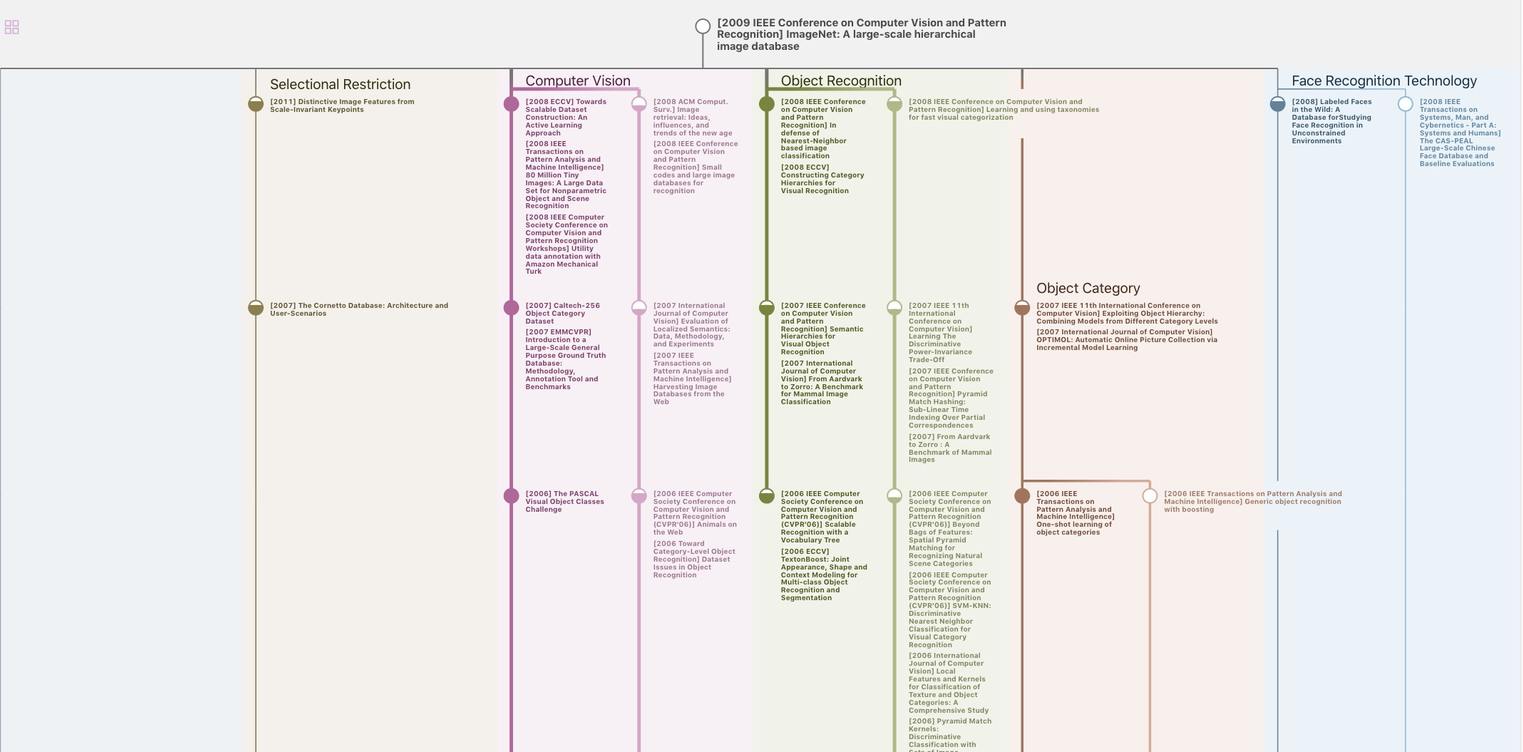
生成溯源树,研究论文发展脉络
Chat Paper
正在生成论文摘要