Interpretable Image Classification Using Sparse Oblique Decision Trees
IEEE International Conference on Acoustics, Speech, and Signal Processing (ICASSP)(2022)
摘要
Interpreting the image datasets is a difficult task, as each image contains a lot of irrelevant data. This paper presents a simple yet effective method to interpret the image datasets. We achieve this by using sparse oblique trees as a tool to select features from the dataset. These trees are not only accurate but also very interpretable. The hierarchical structure of the tree helps to visualize the underlying patterns in the dataset. By studying the weights of the nodes, we can determine what set of features differentiate between classes or groups of classes. We effectively demonstrate our results in multiple image datasets.
更多查看译文
关键词
interpretability,image classification,decision trees
AI 理解论文
溯源树
样例
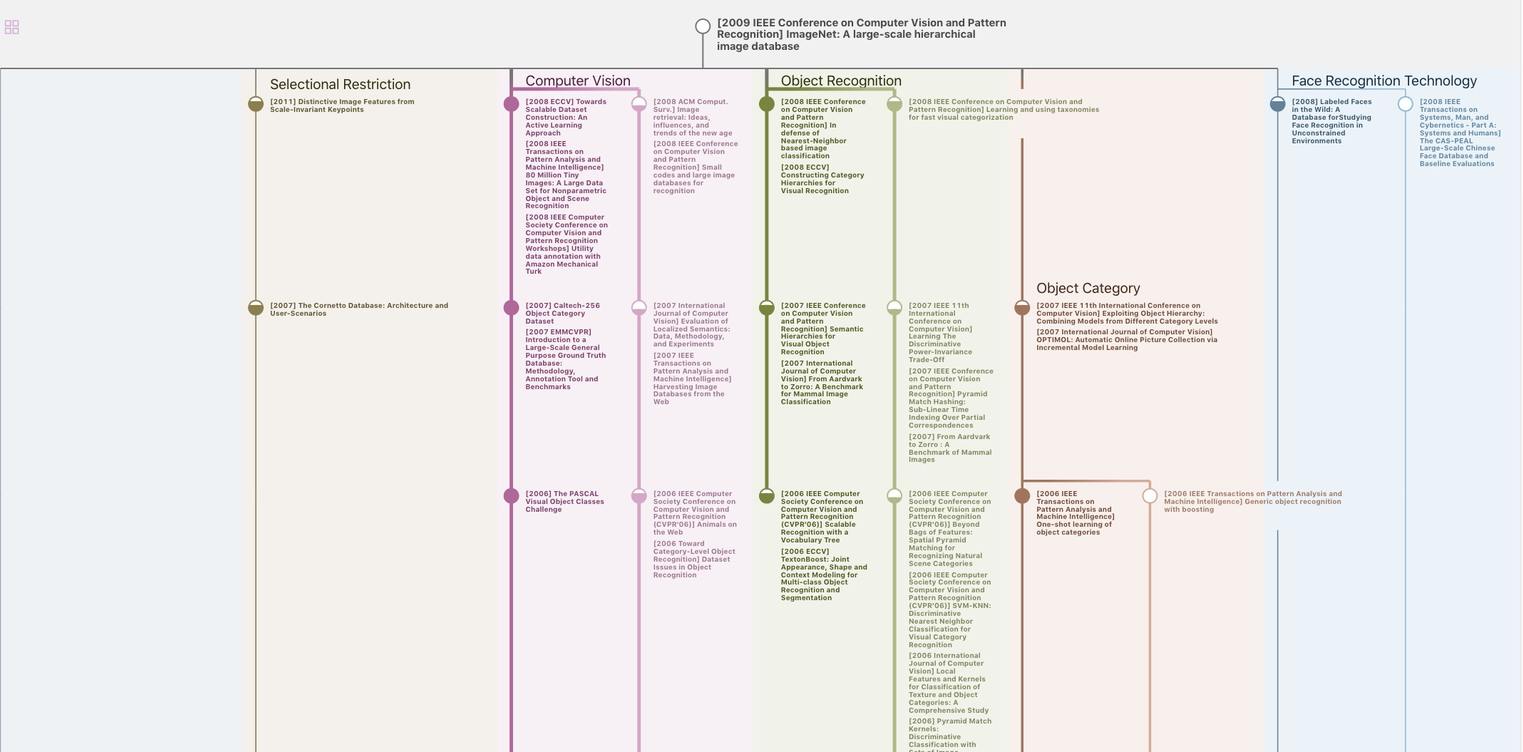
生成溯源树,研究论文发展脉络
Chat Paper
正在生成论文摘要