Time-Frequency and Geometric Analysis of Task-Dependent Learning in Raw Waveform Based Acoustic Models
IEEE International Conference on Acoustics, Speech, and Signal Processing (ICASSP)(2022)
摘要
End-to-end raw-waveform modelling with learnable feature extraction front-ends has shown promising results in various speech/audio tasks. Despite its varied success, there have not been many attempts to understand how spectral/temporal feature integration from raw inputs helps recognize task-dependent information. Towards this aim, this work presents data-dependent and data-independent methods for understanding the modelling behavior of acoustic models. The first method employs time-frequency analysis to visualize input-specific response spectra as a function of short-time front-end block processing. The second method employs geometric properties of layer-wise weights to quantify the impact of architectural choices on signal propagation and trainability of the model. We demonstrate potential of the proposed methods with help of case studies on speech classification, speaker identification, and spoofing classification tasks.
更多查看译文
关键词
Spectral visualization,mutual coherence,acoustic modelling,raw-waveform models
AI 理解论文
溯源树
样例
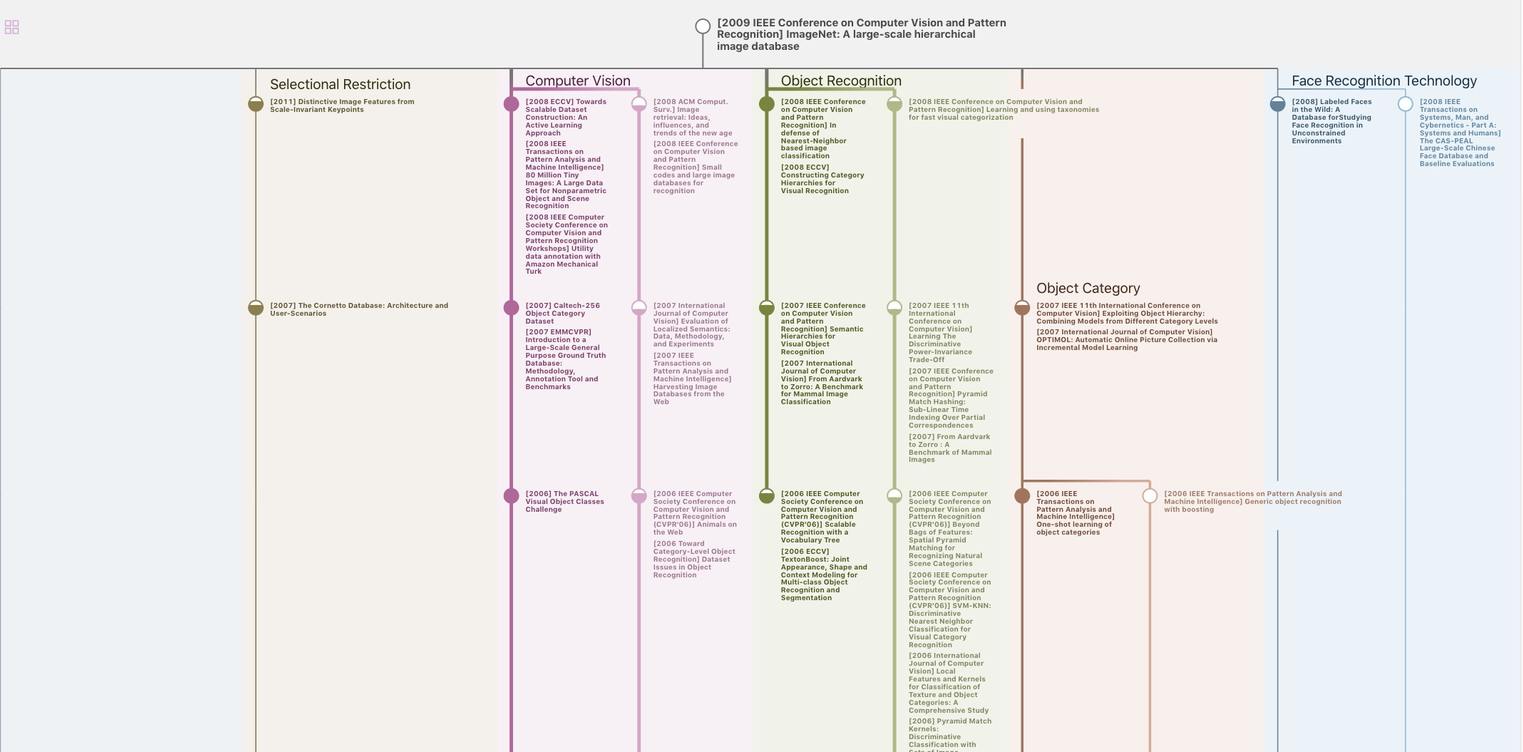
生成溯源树,研究论文发展脉络
Chat Paper
正在生成论文摘要