AuxFormer: Robust Approach to Audiovisual Emotion Recognition
IEEE International Conference on Acoustics, Speech, and Signal Processing (ICASSP)(2022)
摘要
A challenging task in audiovisual emotion recognition is to implement neural network architectures that can leverage and fuse multimodal information while temporally aligning modalities, handling missing modalities, and capturing information from all modalities without losing information during training. These requirements are important to achieve model robustness and to increase accuracy on the emotion recognition task. A recent approach to perform multimodal fusion is to use the transformer architecture to properly fuse and align the modalities. This study proposes the AuxFormer framework, which addresses in a principled way the aforementioned challenges. AuxFormer combines the transformer framework with auxiliary networks. It uses shared losses to infuse information from single-modality networks that are separately embedded. The extra layer of audiovisual information added to our main network retains information that would otherwise be lost during training. The results show that the AuxFormer architecture achieves macro and micro F1-Scores of 71.3% and 71.7%, respectively, on the CREMA-D corpus. For the MSP-IMPROV corpus, AuxFormer achieves a macro and micro F1-Scores of 70.4% and 76.5%, respectively. The results for both corpora are significantly better than strong baselines, indicating that our framework benefits from auxiliary networks. We also show that under non-ideal conditions (e.g., missing modalities) our architecture is able to sustain strong performance under audio-only and video-only scenarios, benefiting from a optimized training strategy.
更多查看译文
关键词
Audiovisual emotion recognition,shared losses,multimodal fusion,transformers,auxiliary networks
AI 理解论文
溯源树
样例
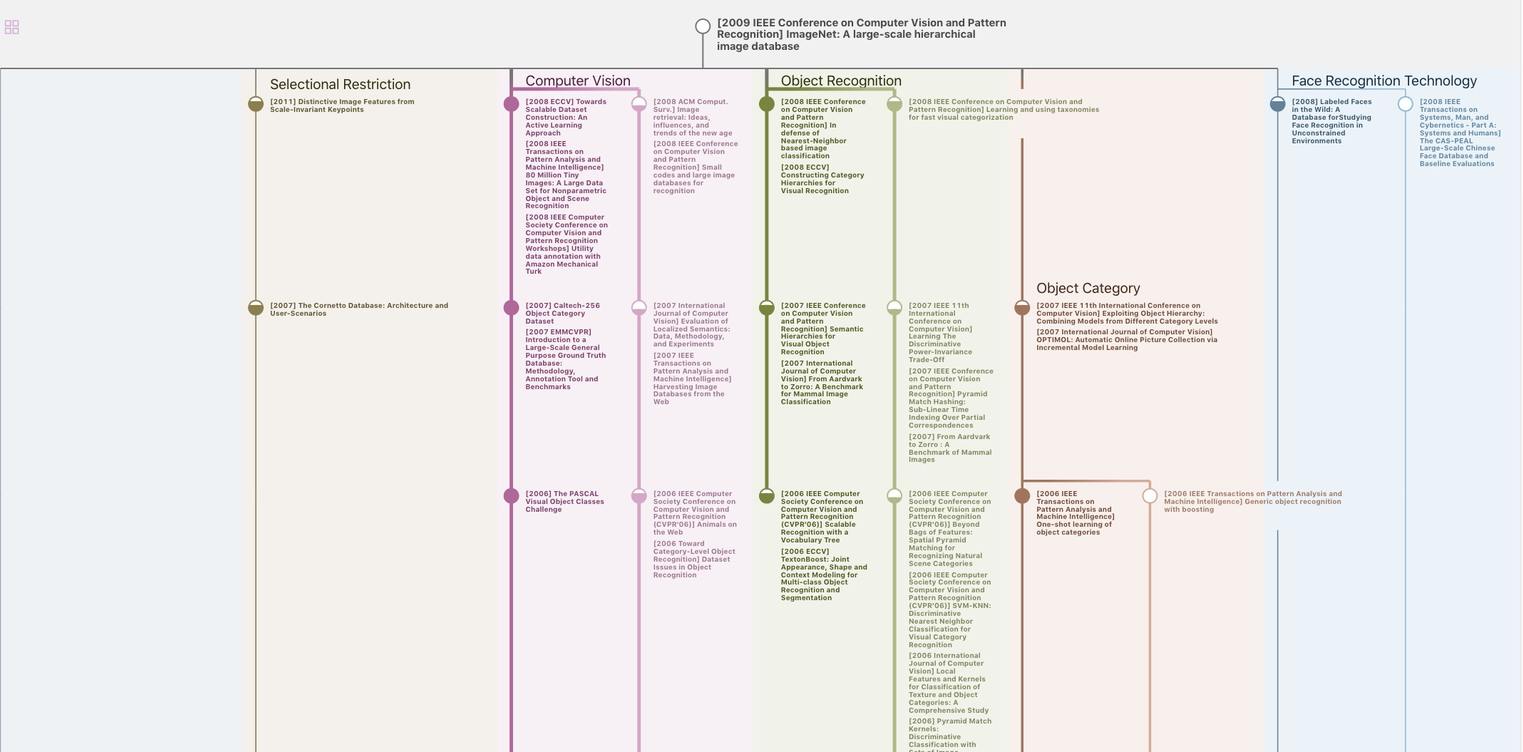
生成溯源树,研究论文发展脉络
Chat Paper
正在生成论文摘要