Automatic Parameter Selection for Non-Redundant Clustering
SIAM International Conference on Data Mining (SDM)(2023)
摘要
High-dimensional datasets often contain multiple meaningful clusterings in
different subspaces. For example, objects can be clustered either by color,
weight, or size, revealing different interpretations of the given dataset. A
variety of approaches are able to identify such non-redundant clusterings.
However, most of these methods require the user to specify the expected number
of subspaces and clusters for each subspace. Stating these values is a
non-trivial problem and usually requires detailed knowledge of the input
dataset. In this paper, we propose a framework that utilizes the Minimum
Description Length Principle (MDL) to detect the number of subspaces and
clusters per subspace automatically. We describe an efficient procedure that
greedily searches the parameter space by splitting and merging subspaces and
clusters within subspaces. Additionally, an encoding strategy is introduced
that allows us to detect outliers in each subspace. Extensive experiments show
that our approach is highly competitive to state-of-the-art methods.
更多查看译文
关键词
clustering,selection,non-redundant
AI 理解论文
溯源树
样例
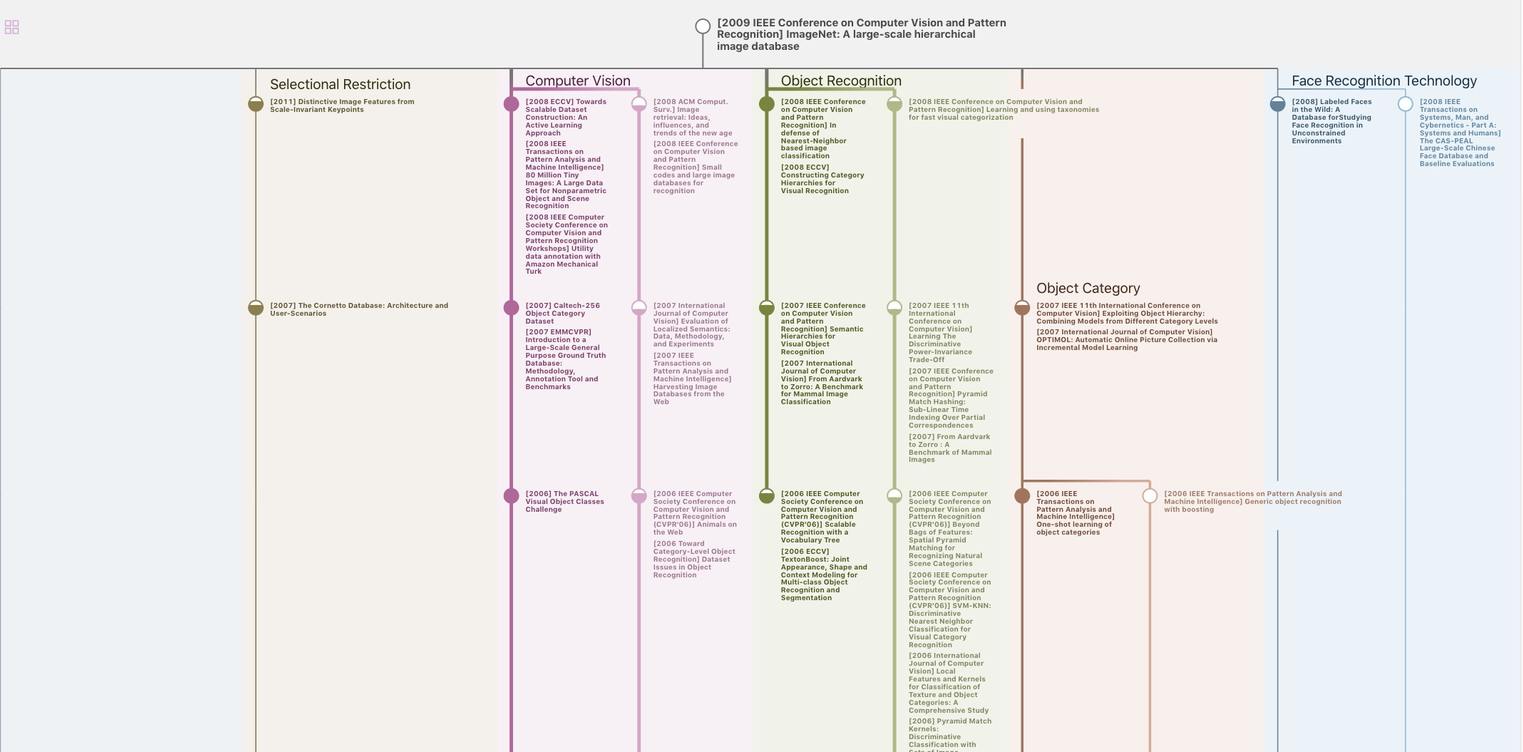
生成溯源树,研究论文发展脉络
Chat Paper
正在生成论文摘要