Poster Abstract: Representation Learning from Multimodal Sensor Data with Maximally Correlated Autoencoders
2022 21st ACM/IEEE International Conference on Information Processing in Sensor Networks (IPSN)(2022)
摘要
With the development of sensing technology, multiple sensors are widely used in Internet of Things (IoT) devices. A key challenge is to learn feature representations from multimodal sensor data to combine the information of different sensors. Although progress has been made by previous works, the correlation between different sensors is still not well exploited, which may limit the performance of representation learning. To address this problem, we propose a deep learning approach to learn representations from multimodal sensor data with maximally correlated autoencoders (MCA). It can efficiently capture high dependence between different modalities at different feature levels. The learned representations are further used for the recognition task. Experimental results on the real-world RGB- D dataset demonstrate the high effectiveness of MCA.
更多查看译文
关键词
representation learning,multimodal sensor data,maximally correlated autoencoders,feature representations,deep learning approach,Internet-of-Things devices,IoT devices,MCA effectiveness,real-world RGB- D dataset
AI 理解论文
溯源树
样例
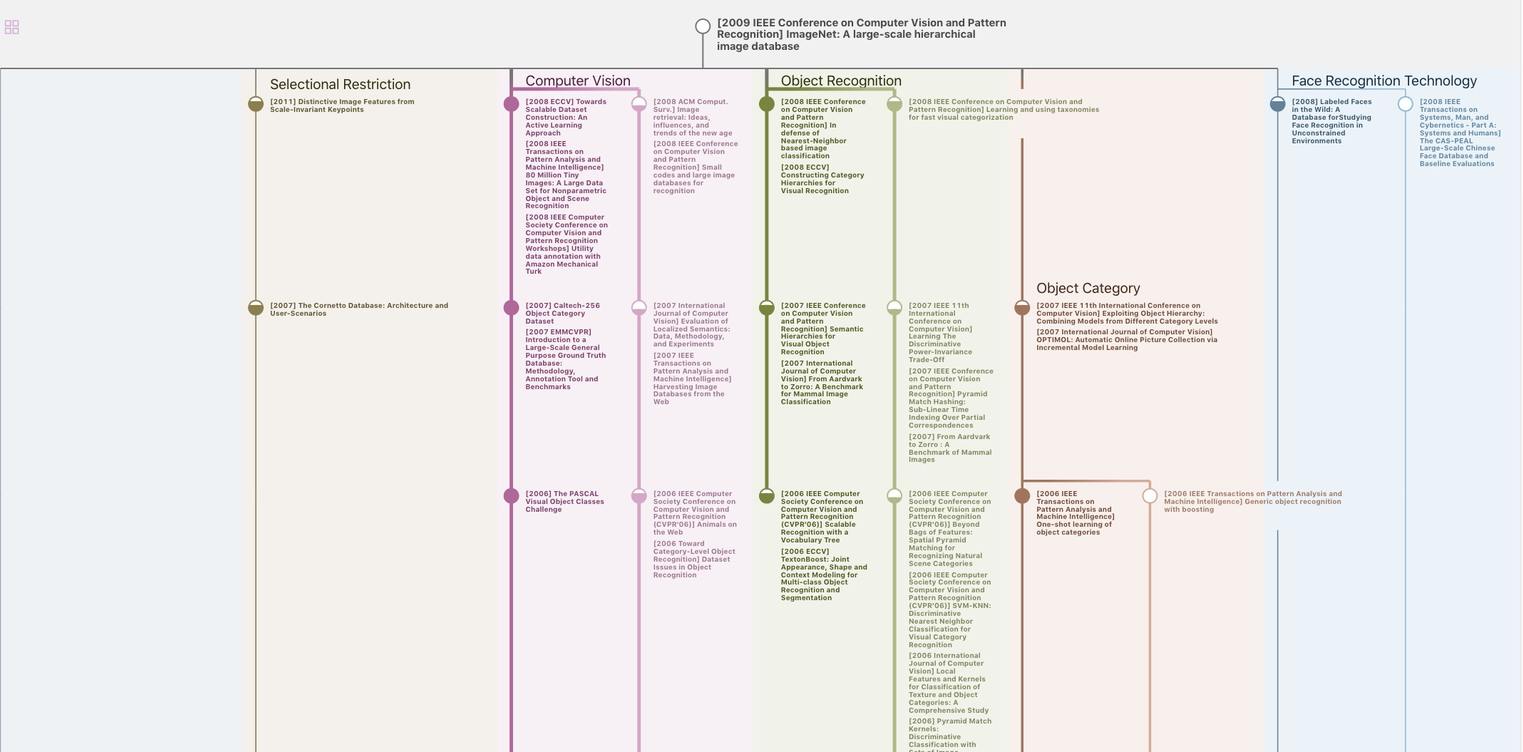
生成溯源树,研究论文发展脉络
Chat Paper
正在生成论文摘要