No-reference Omnidirectional Image Quality Assessment Based on Joint Network
International Multimedia Conference(2022)
摘要
ABSTRACTIn panoramic multimedia applications, the perception quality of the omnidirectional content often comes from the observer's perception of the viewports and the overall impression after browsing. Starting from this hypothesis, this paper proposes a deep-learning based joint network to model the no-reference quality assessment of omnidirectional images. On the one hand, motivated by different scenarios that lead to different human understandings, a convolutional neural network (CNN) is devised to simultaneously encode the local quality features and the latent perception rules of different viewports, which are more likely to be noticed by the viewers. On the other hand, a recurrent neural network (RNN) is designed to capture the interdependence between viewports from their sequence representation, and then predict the impact of each viewport on the observer's overall perception. Experiments on two popular omnidirectional image quality databases demonstrate that the proposed method outperforms the state-of-the-art omnidirectional image quality metrics.
更多查看译文
关键词
image quality assessment,joint network,no-reference
AI 理解论文
溯源树
样例
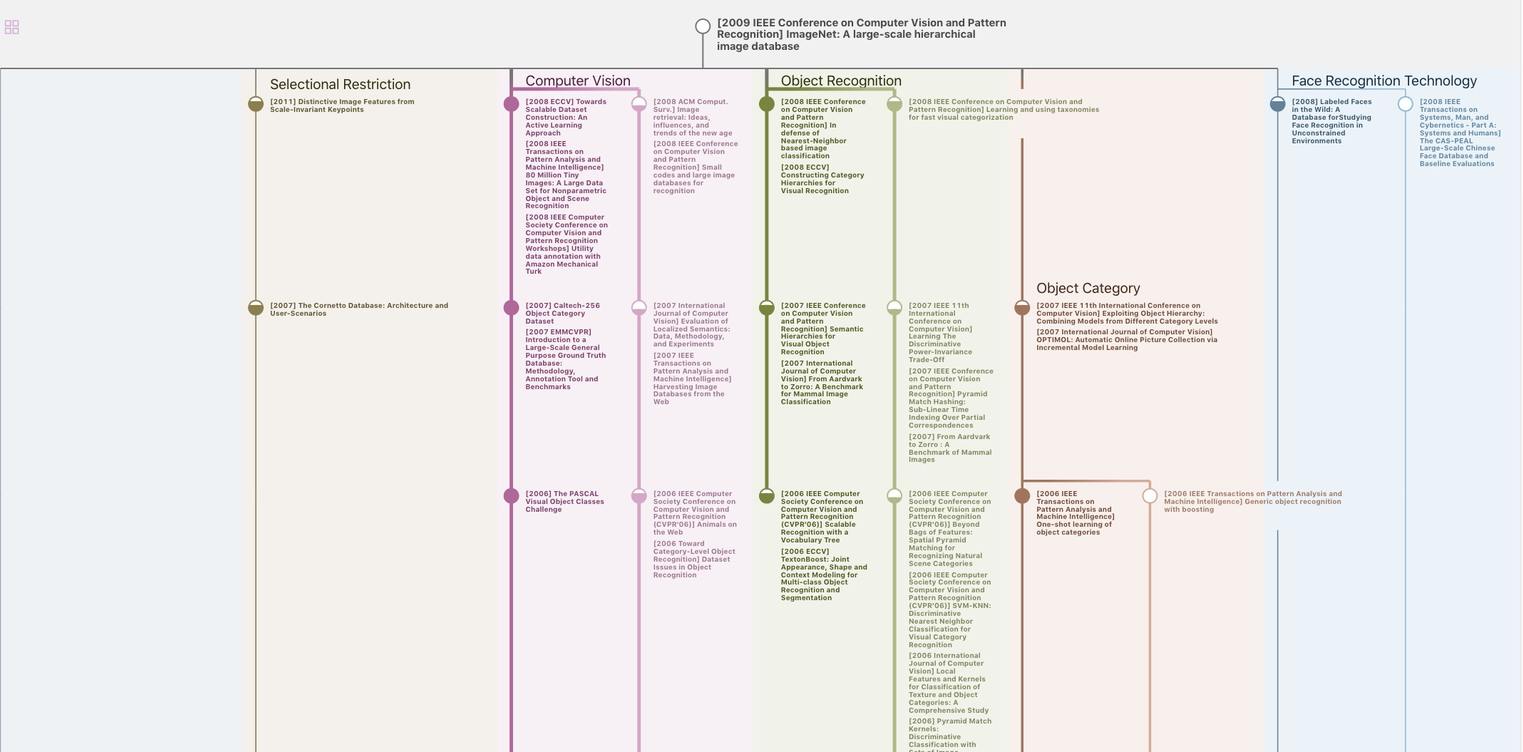
生成溯源树,研究论文发展脉络
Chat Paper
正在生成论文摘要