Active Patterns Perceived for Stochastic Video Prediction
International Multimedia Conference(2022)
摘要
ABSTRACTPredicting future scenes based on historical frames is challenging, especially when it comes to the complex uncertainty in nature. We observe that there is a divergence between spatial-temporal variations of active patterns and non-active patterns in a video, where these patterns constitute visual content and the former ones implicate more violent movement. This divergence enables active patterns the higher potential to act with more severe future uncertainty. Meanwhile, the existence of non-active patterns provides an opportunity for machines to examine some underlying rules with a mutual constraint between non-active patterns and active patterns. In order to solve this divergence, we provide a method called active patterns-perceived stochastic video prediction (ASVP) which allows active patterns to be perceived by neural networks during training. Our method starts with separating active patterns along with non-active ones from a video. Then, both scene-based prediction and active pattern-perceived prediction are conducted to respectively capture the variations within the whole scene and active patterns. Specially for active pattern-perceived prediction, a conditional generative adversarial network (CGAN) is exploited to model active patterns as conditions, with a variational autoencoder (VAE) for predicting the complex dynamics of active patterns. Additionally, a mutual constraint is designed to improve the learning procedure for the network to better understand underlying interacting rules among these patterns. Extensive experiments are conducted on both KTH human action and BAIR action-free robot pushing datasets with comparison to state-of-the-art works. Experimental results demonstrate the competitive performance of the proposed method as we expected. The released code and models are at https://github.com/tolearnmuch/ASVP.
更多查看译文
关键词
active patterns,prediction,video
AI 理解论文
溯源树
样例
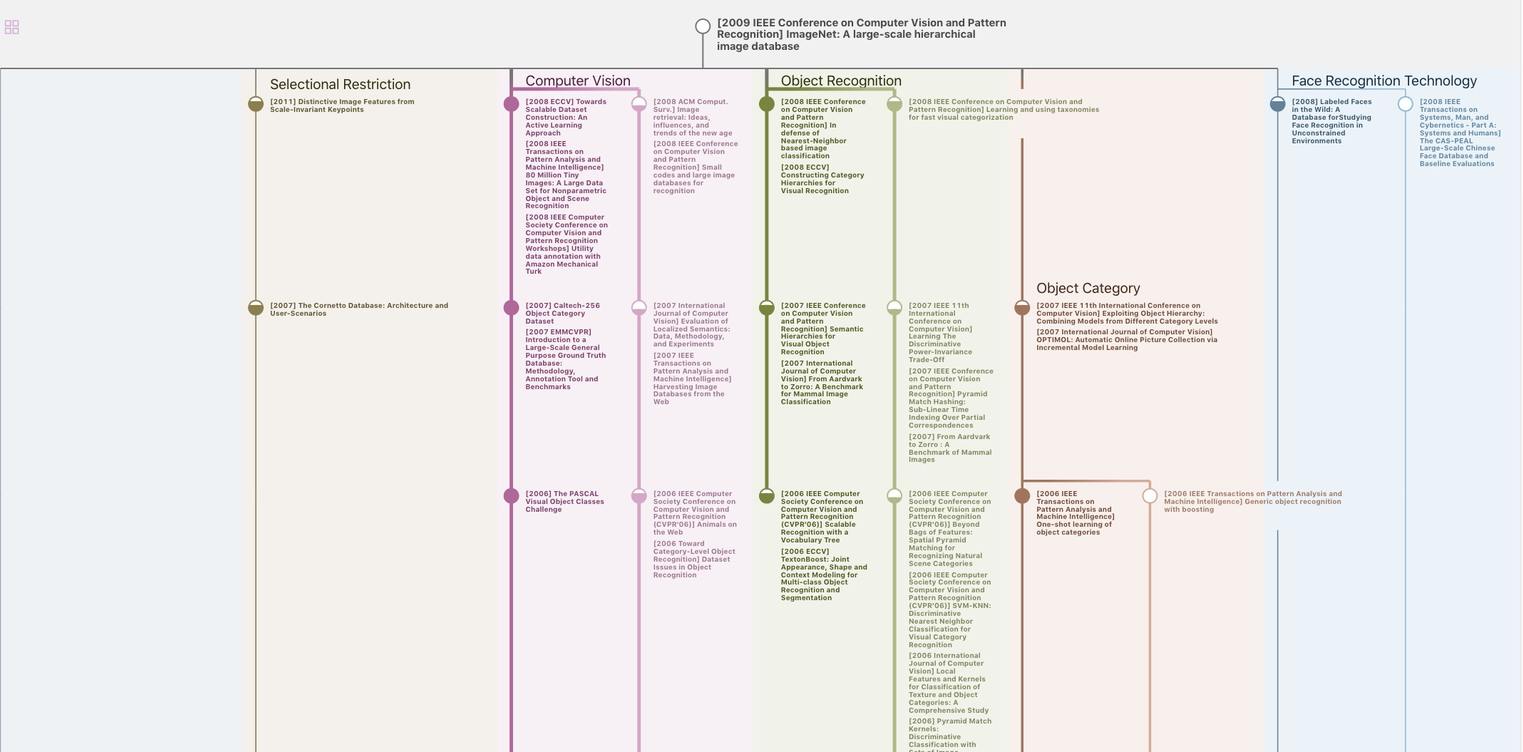
生成溯源树,研究论文发展脉络
Chat Paper
正在生成论文摘要