Dynamic Scene Graph Generation via Temporal Prior Inference
International Multimedia Conference(2022)
摘要
ABSTRACTReal-world videos are composed of complex actions with inherent temporal continuity (eg "person-touching-bottle" is usually followed by "person-holding-bottle"). In this work, we propose a novel method to mine such temporal continuity for dynamic scene graph generation (DSGG), namely Temporal Prior Inference (TPI). As opposed to current DSGG methods, which individually capture the temporal dependence of each video by refining representations, we make the first attempt to explore the temporal continuity by extracting the entire co-occurrence patterns of action categories from a variety of videos in Action Genome (AG) dataset. Then, these inherent patterns are organized as Temporal Prior Knowledge (TPK) which serves as prior knowledge for models' learning and inference. Furthermore, given the prior knowledge, human-object relationships in current frames can be effectively inferred from adjacent frames via the robust Temporal Prior Inference algorithm with tiny computation cost. Specifically, to efficiently guide the generating of temporal-consistent dynamic scene graphs, we incorporate the temporal prior inference into a DSGG framework by introducing frame enhancement, continuity loss, and fast inference. The proposed model-agnostic strategies significantly boost the performances of existing state-of-the-art models on the Action Genome dataset, achieving 69.7 and 72.6 for [email protected] and [email protected] on PredCLS. In addition, the inference speed can be significantly reduced by 41% with an acceptable drop on [email protected] (69.7 to 66.8) by utilizing fast inference.
更多查看译文
关键词
dynamic scene graph generation
AI 理解论文
溯源树
样例
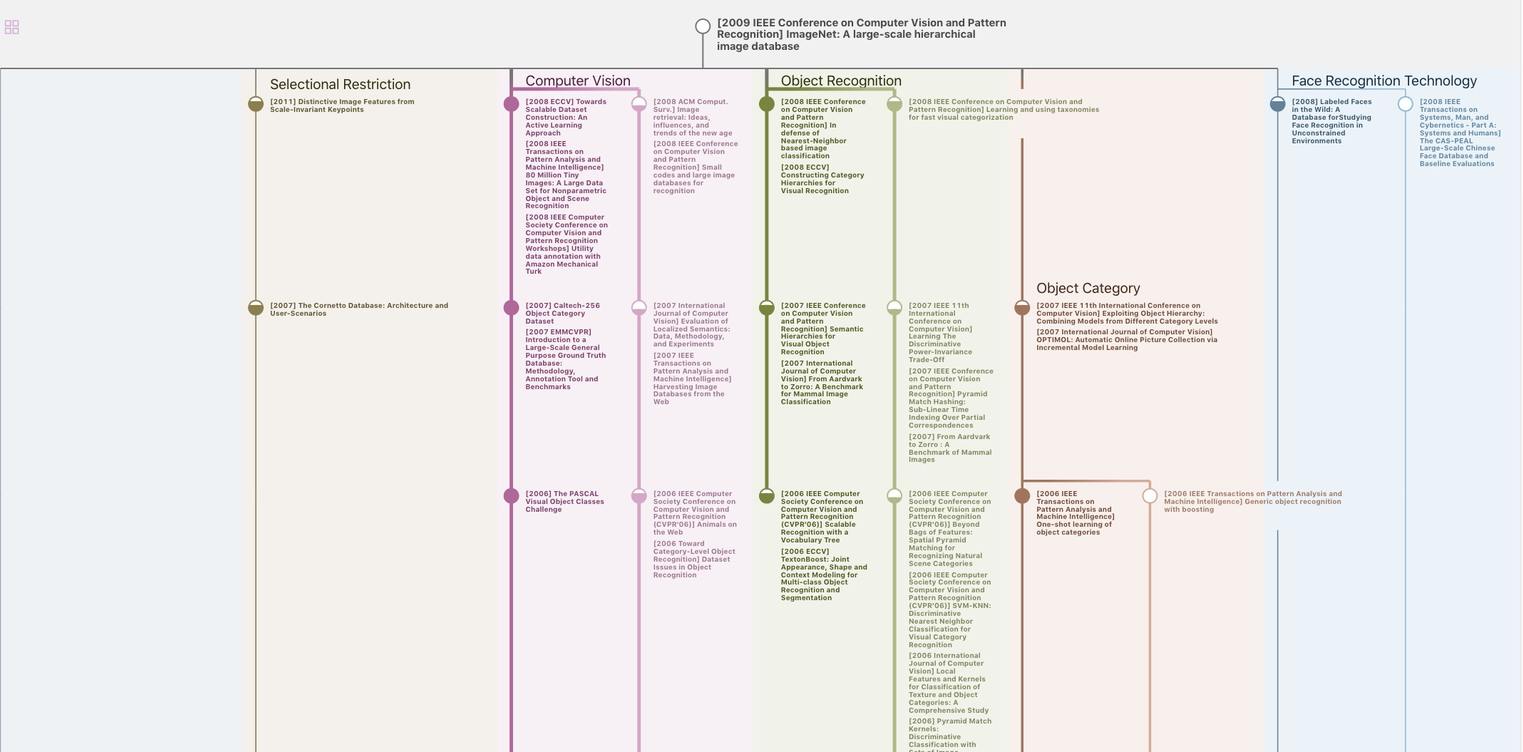
生成溯源树,研究论文发展脉络
Chat Paper
正在生成论文摘要