Learning an Inference-accelerated Network from a Pre-trained Model with Frequency-enhanced Feature Distillation
International Multimedia Conference(2022)
摘要
ABSTRACTConvolution neural networks (CNNs) have achieved great success in various computer vision tasks, but they are still suffering from the heavy computation costs, which are mainly resulted from the substantial redundancy of the feature maps. In order to reduce these redundancy, we proposed a simple but effective frequency-enhanced feature distillation strategy to train an inference-accelerated network with a pre-trained model. Traditionally, one CNN can be regarded as a hierarchical structure, which can generate the low-level, middle-level and high-level feature maps from different convolution layers. In order to accelerate the inference time of CNNs, in this paper, we propose to resize the low-level and middle-level feature maps to smaller scales to reduce the spatial computation costs of CNNs. A frequency-enhanced feature distillation training strategy with a pre-trained model is then used to help the inference-accelerated network to maintain the core information after resizing the feature maps. To be specific, the original pre-trained network and the inference-accelerated network with resized feature maps are regarded as the teacher network and student network respectively. Considering that the low-frequency domain of the feature maps contribute the most parts to the final classification, we then transform the feature maps of different levels into a frequency-enhanced feature space, which highlights the low-frequency features for both the teacher and student networks. The frequency-enhanced features are used to transfer the knowledge from the teacher network to the student network. At the same time, knowledge for the final classification, i.e., the classification feature and predicted probabilities, are also used for distillation. Experiments on multiple databases based on various network structure types, e.g., ResNet, Res2Net, MobileNetV2, and ConvNeXt, have shown that with the proposed frequency-enhanced feature distillation training strategy, our method could get an inference-accelerated network with comparable performance and much less computation cost.
更多查看译文
关键词
learning,model,network,feature,inference-accelerated,pre-trained,frequency-enhanced
AI 理解论文
溯源树
样例
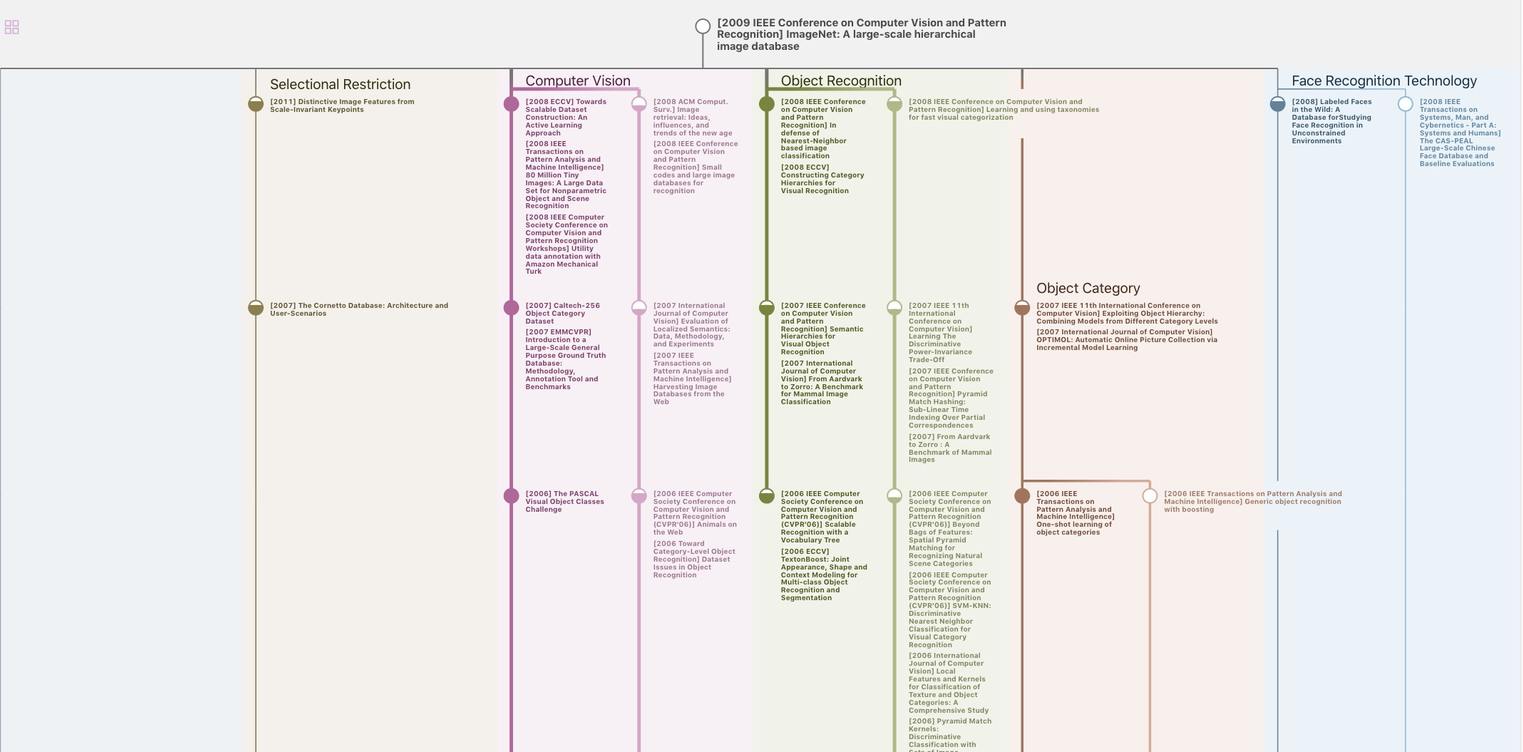
生成溯源树,研究论文发展脉络
Chat Paper
正在生成论文摘要