Pyramidal Transformer with Conv-Patchify for Person Re-identification
International Multimedia Conference(2022)
摘要
ABSTRACTThe robust and discriminative feature extraction is the key component in person re-identification (Re-ID). The major weakness of conventional convolution neural network (CNN) based methods is that they cannot extract long-range information from diverse parts, which can be alleviated by recently developed Transformers. Existing vision Transformers show their power on various vision tasks. However, they (i) cannot address translation problems and different viewpoints; (ii) cannot capture detailed features to discriminate people with a similar appearance. In this paper, we propose a powerful Re-ID baseline built on top of the pyramidal transformer with conv-patchify operation, termed PTCR, which inherits the advantages of both CNN and Transformer. The pyramidal structure captures multi-scale fine-grained features, while the conv-patchify enhances the robustness against translation. Moreover, we additionally design two novel modules to improve the robust feature learning. A Token Perception module augments the patch embeddings to enhance the robustness against perturbation and viewpoint changes, while the Auxiliary Embedding module integrates the auxiliary information (cam ID, pedestrian attributes, etc) to reduce feature bias caused by non-visual factors. Our method is validated through extensive experiments to show its superior performance with abundant ablation studies. Notably, without re-ranking, we achieve 98.0% Rank-1 on Market-1501 and 88.6% Rank-1 on MSMT17, significantly outperforming the counterparts. The code is available at: https://github.com/lihe404/PTCR
更多查看译文
关键词
transformer,person,conv-patchify,re-identification
AI 理解论文
溯源树
样例
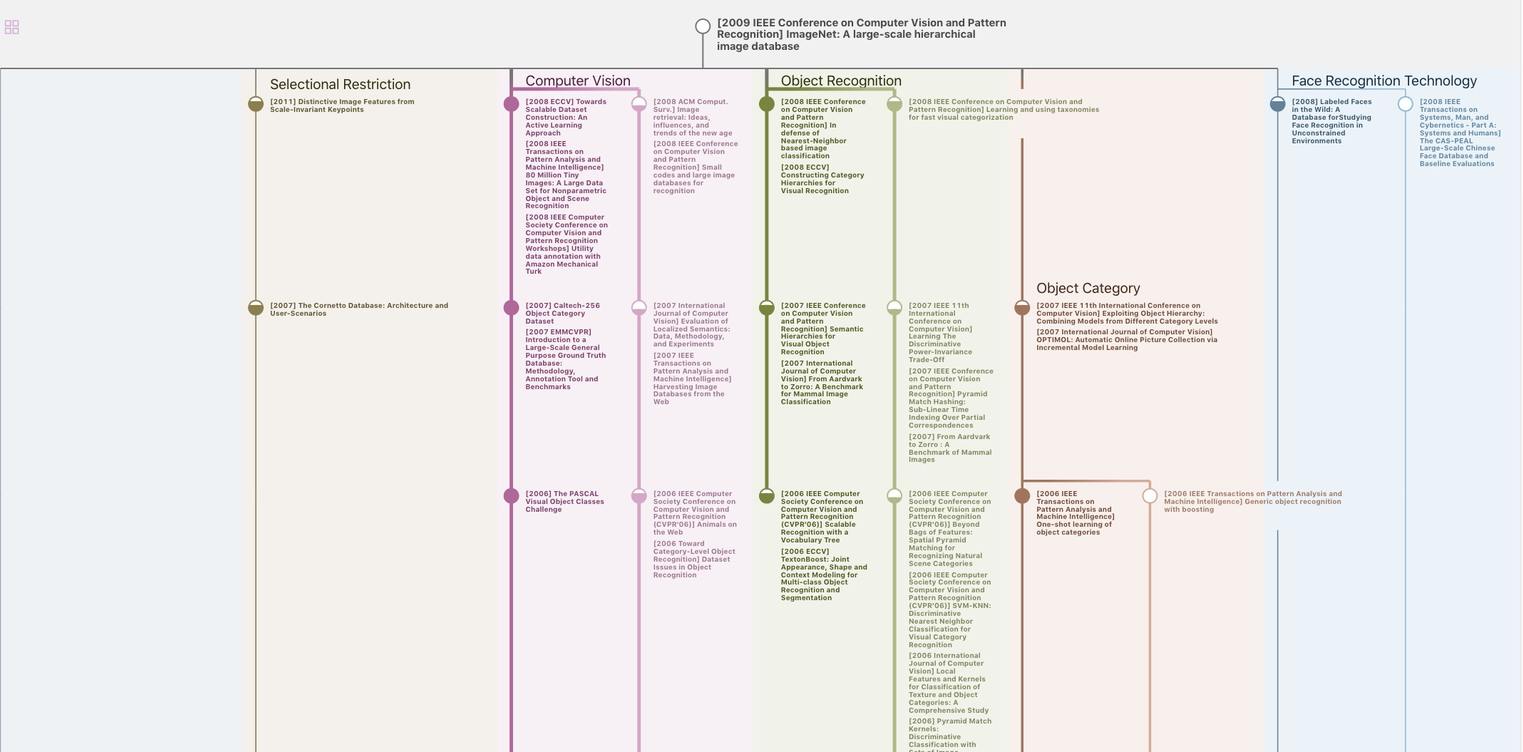
生成溯源树,研究论文发展脉络
Chat Paper
正在生成论文摘要