Dynamically Adjust Word Representations Using Unaligned Multimodal Information
International Multimedia Conference(2022)
摘要
ABSTRACTMultimodal Sentiment Analysis is a promising research area for modeling multiple heterogeneous modalities. Two major challenges that exist in this area are a) multimodal data is unaligned in nature due to the different sampling rates of each modality, and b) long-range dependencies between elements across modalities. These challenges increase the difficulty of conducting efficient multimodal fusion. In this work, we propose a novel end-to-end network named Cross Hyper-modality Fusion Network (CHFN). The CHFN is an interpretable Transformer-based neural model that provides an efficient framework for fusing unaligned multimodal sequences. The heart of our model is to dynamically adjust word representations in different non-verbal contexts using unaligned multimodal sequences. It is concerned with the influence of non-verbal behavioral information at the scale of the entire utterances and then integrates this influence into verbal expression. We conducted experiments on both publicly available multimodal sentiment analysis datasets CMU-MOSI and CMU-MOSEI. The experiment results demonstrate that our model surpasses state-of-the-art models. In addition, we visualize the learned interactions between language modality and non-verbal behavior information and explore the underlying dynamics of multimodal language data.
更多查看译文
关键词
dynamically adjust word representations
AI 理解论文
溯源树
样例
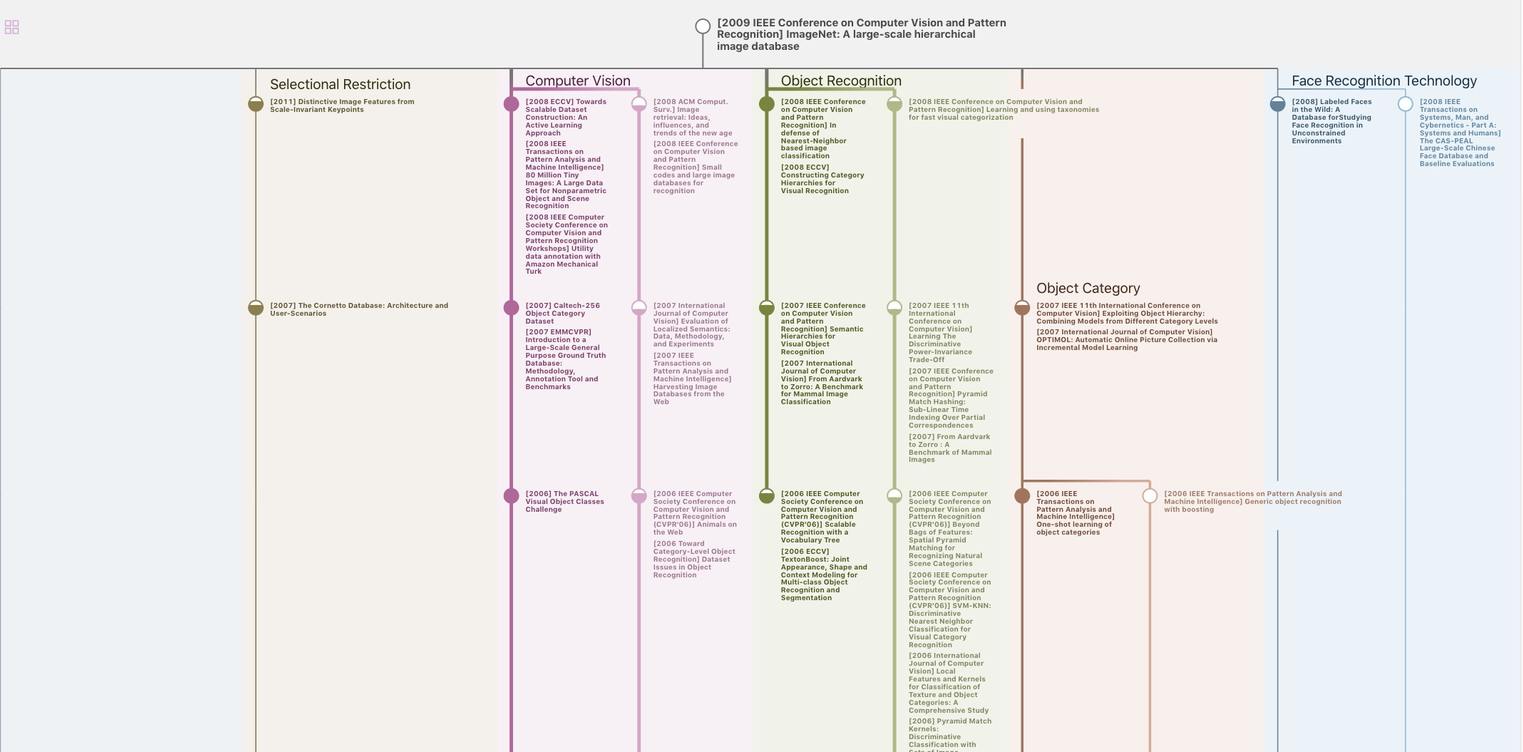
生成溯源树,研究论文发展脉络
Chat Paper
正在生成论文摘要