PDD-GAN: Prior-based GAN Network with Decoupling Ability for Single Image Dehazing
International Multimedia Conference(2022)
摘要
ABSTRACTSingle image dehazing is a challenging vision problem aiming to provide clear images for downstream computer vision applications (e.g., semantic segmentation, object detection, and super resolution). Most existing methods leverage the physical scattering model or convolutional neural networks (CNNs) for haze removal, which however ignore the complementary advantages between each other. Especially lacking marginal and visual prior instructions, CNN-based methods still have gaps in details and color recovery. To solve these, we propose a Prior-based with Decoupling ability Dehazing GAN Network (PDD-GAN), which is based on PeleetNet and attached with an attention module (CBAM). The prior-based decoupling approach consists of two parts: high and low frequency filtering and HSV contrastive loss. We process the image via a band-stop filter and add it as the fourth channel of data (RGBFHL) to decouple the hazy image at the structural level. Besides, a novel prior loss with contrastive regularization is proposed at the visual level. Sufficient experiments are carried out to demonstrate that PDD-GAN outperforms state-of-the-art methods by up to 0.86db in PSNR. In particular, extensive experiments indicate that RGBFHL increases by 0.99db compared with the original three-channel data (RGB) and the extra HSV prior loss escalates by 2.0db. Above all, our PDD-GAN indeed has the decoupling ability and improves the dehazing results.
更多查看译文
AI 理解论文
溯源树
样例
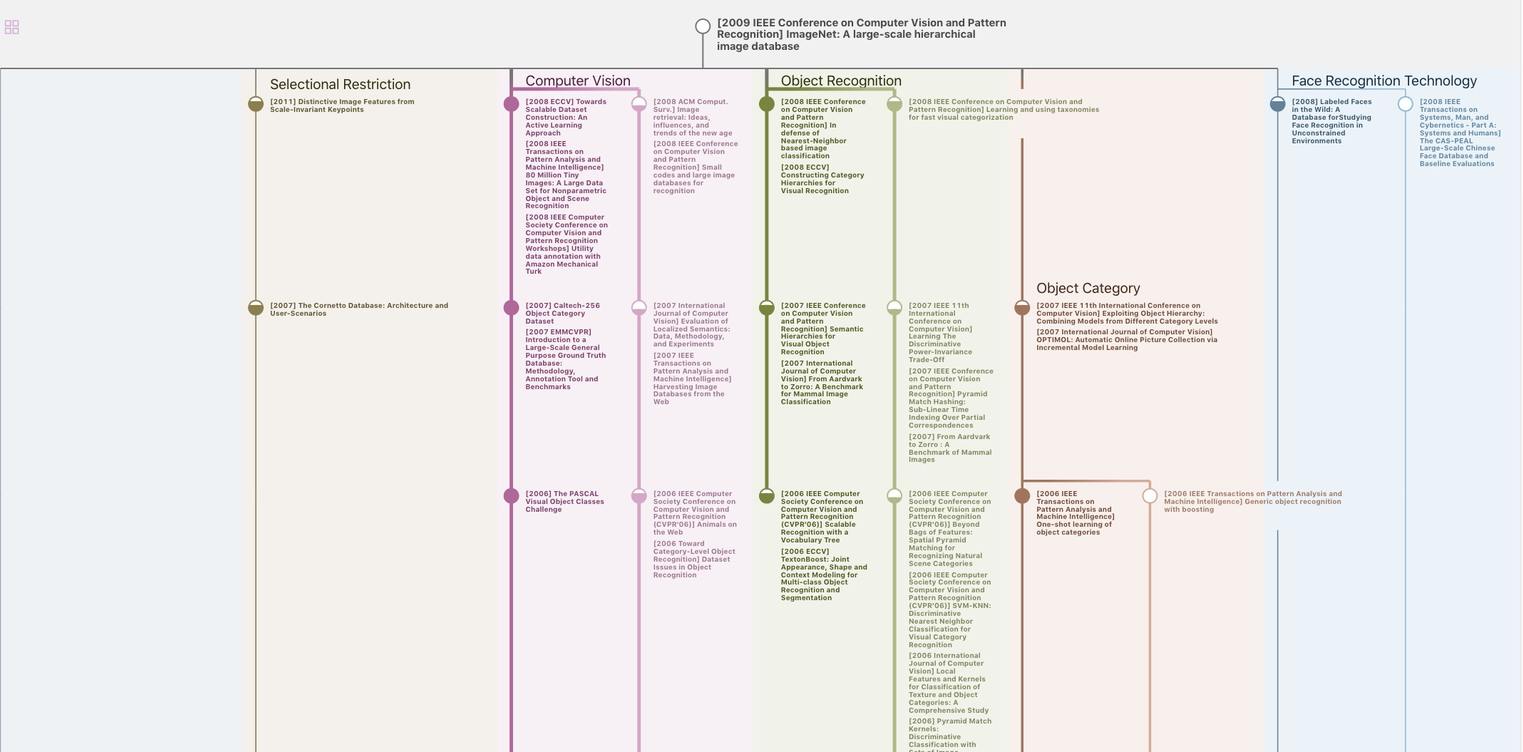
生成溯源树,研究论文发展脉络
Chat Paper
正在生成论文摘要