MC-SLT: Towards Low-Resource Signer-Adaptive Sign Language Translation
International Multimedia Conference(2022)
摘要
ABSTRACTOne of the challenging factors in real application of sign language translation (SLT) is inter-signer variation. With the assumption that the pre-trained translation model cannot cover all the signers, the adaptation capability for unseen signers is of great concern. In this paper, we take a completely different perspective for SLT, called signer-adaptive SLT, which mainly considers the transferable ability of SLT systems. To attack this challenging problem, we propose MC-SLT, a novel meta-learning framework that could exploit additional new-signer data via a support set, and output a signer-adaptive model via a few-gradient-step update. Considering the various degrees of style discrepancies of different words performed by multiple signers, we further devise diversity-aware meta-adaptive weights for the token-wise cross-entropy losses. Besides, to improve the training robustness, we adopt the self-guided curriculum learning scheme that first captures the global curricula from each signer to avoid falling into a bad local optimum early, and then learns the curricula of individualities to improve the model adaptability for learning signer-specific knowledge. We re-construct the existing standard datasets of SLT for the signer-adaptive setting and establish a new benchmark for subsequent research.
更多查看译文
关键词
signer-adaptive,language translation,mc-slt,low-resource,signer-adaptive
AI 理解论文
溯源树
样例
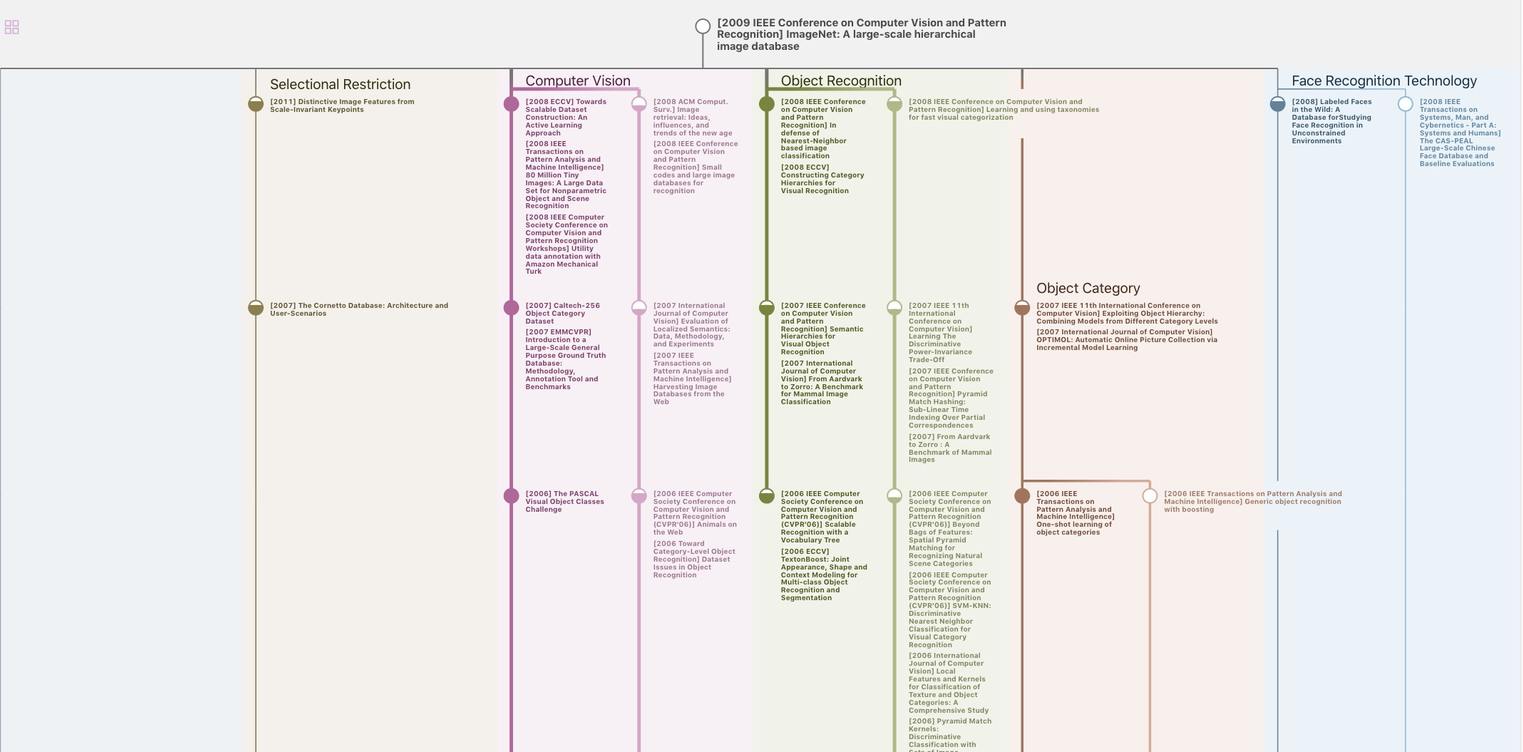
生成溯源树,研究论文发展脉络
Chat Paper
正在生成论文摘要