SAMBA: A System for Secure Federated Multi-Armed Bandits
2022 IEEE 38TH INTERNATIONAL CONFERENCE ON DATA ENGINEERING (ICDE 2022)(2022)
摘要
The federated learning paradigm allows several data owners to contribute to a machine learning task without exposing their potentially sensitive data. We focus on cumulative reward maximization in Multi-Armed Bandits (MAB), a classical reinforcement learning model for decision making under uncertainty. We demonstrate SAMBA, a generic framework for Secure federAted Multi-armed BAndits. The demonstration platform is a Web interface that simulates the distributed components of SAMBA, and which helps the data scientist to configure the end-to-end workflow of deploying a federated MAB algorithm. The user-friendly interface of SAMBA allows the users to examine the interaction between three key dimensions of federated MAB: cumulative reward, computation time, and security guarantees. We demonstrate SAMBA with two real-world datasets: Google Local Reviews and Steam Video Game.
更多查看译文
关键词
machine learning security,multi-armed bandits,federated learning,secure protocol
AI 理解论文
溯源树
样例
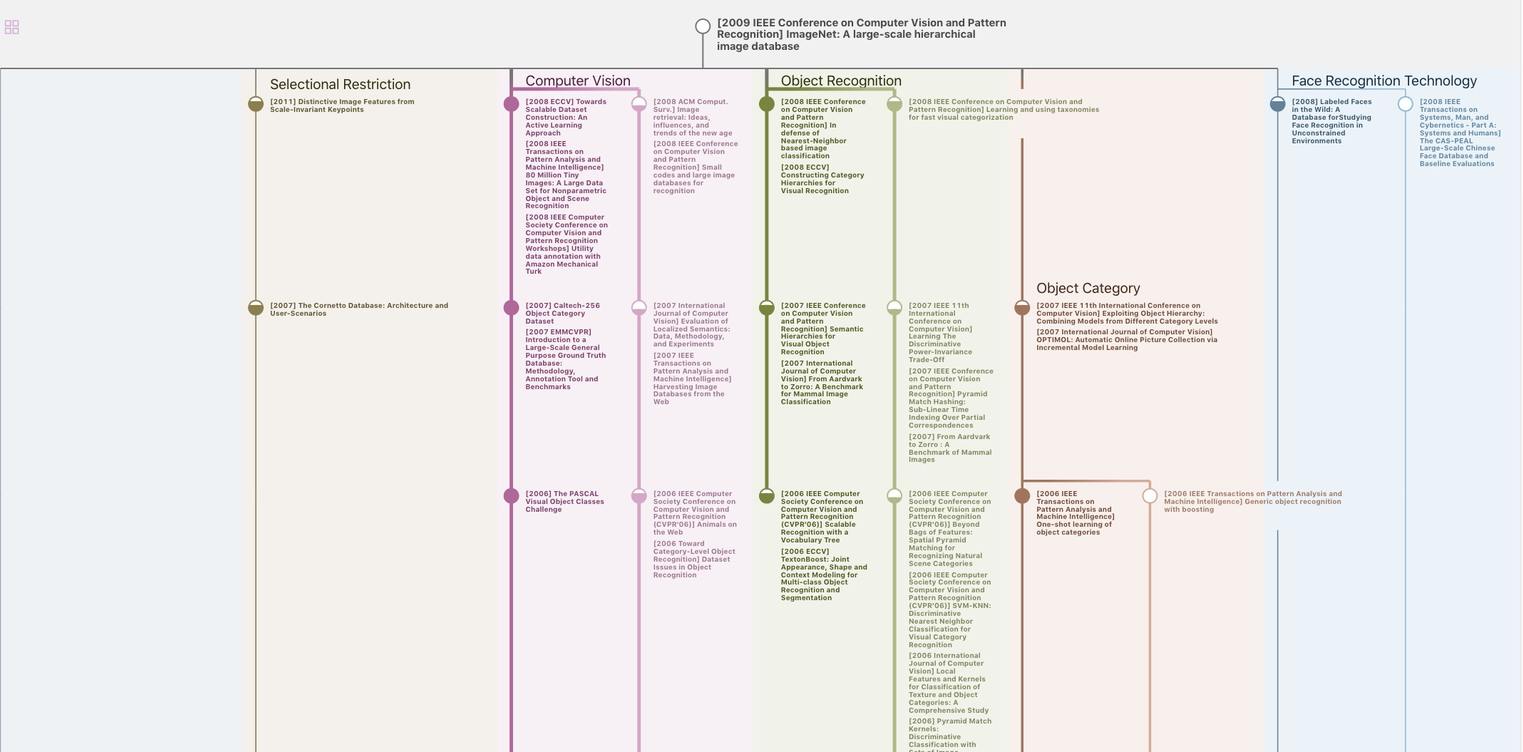
生成溯源树,研究论文发展脉络
Chat Paper
正在生成论文摘要