Anomaly Detection in Time Series with Robust Variational Quasi-Recurrent Autoencoders
2022 IEEE 38TH INTERNATIONAL CONFERENCE ON DATA ENGINEERING (ICDE 2022)(2022)
摘要
We propose variational quasi-recurrent autoencoders (VQRAEs) to enable robust and efficient anomaly detection in time series in unsupervised settings. The proposed VQRAEs employs a judiciously designed objective function based on robust divergences, including alpha, beta, and gamma-divergence, making it possible to separate anomalies from normal data without the reliance on anomaly labels, thus achieving robustness and fully unsupervised training. To better capture temporal dependencies in time series data, VQRAEs are built upon quasi-recurrent neural networks, which employ convolution and gating mechanisms to avoid the inefficient recursive computations used by classic recurrent neural networks. Further, VQRAEs can be extended to bi-directional BiVQRAEs that utilize bi-directional information to further improve the accuracy. The above design choices make VQRAEs not only robust and thus accurate, but also efficient at detecting anomalies in streaming settings. Experiments on five realworld time series offer insight into the design properties of VQRAEs and demonstrate that VQRAEs are capable of outperforming stateof-the-art methods.
更多查看译文
关键词
robust detection,efficient anomaly detection,unsupervised settings,judiciously designed objective function,robust divergences,anomaly labels,fully unsupervised training,time series data,quasirecurrent neural networks,classic recurrent neural networks,bi-directional Bi VQRAEs,real-world time series offer insight,robust variational quasirecurrent autoencoders
AI 理解论文
溯源树
样例
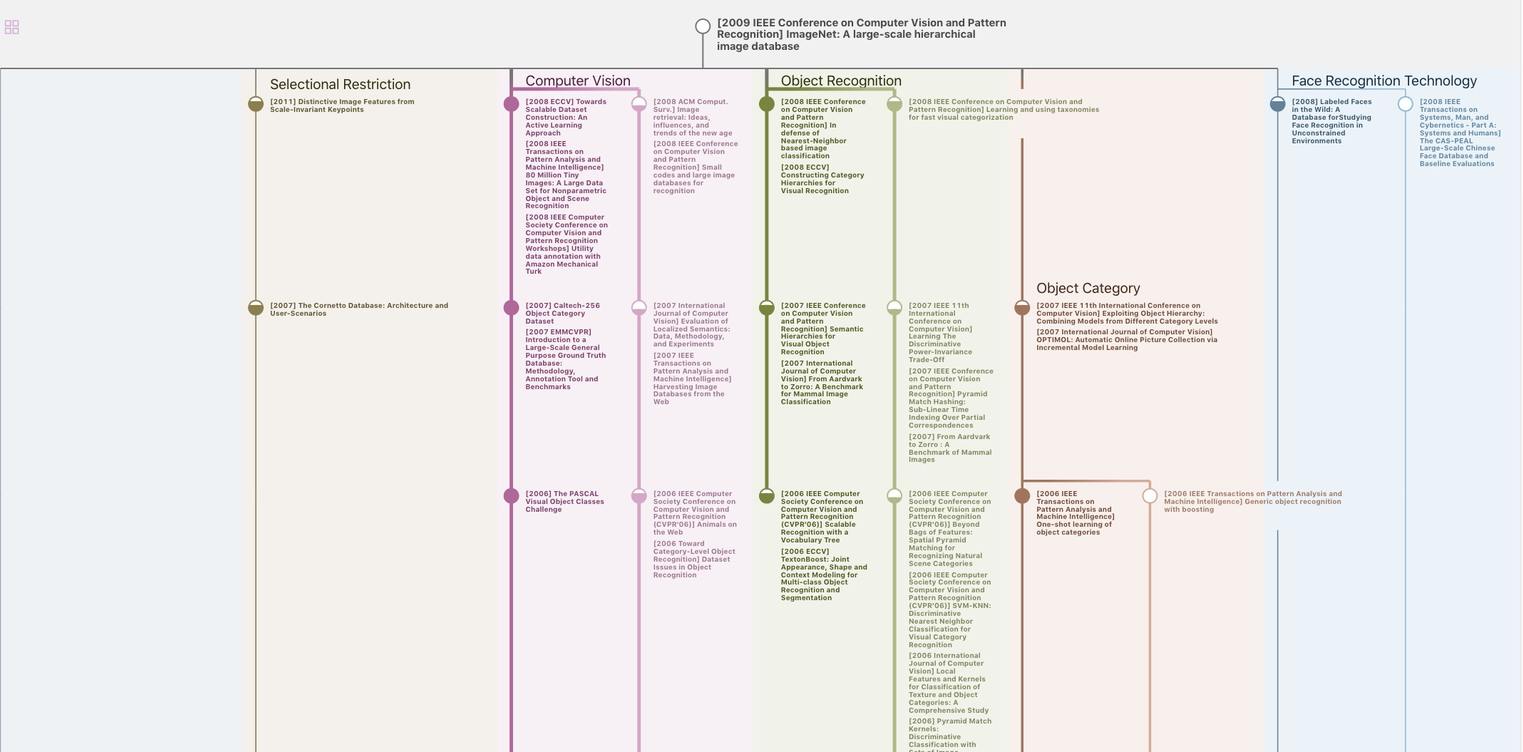
生成溯源树,研究论文发展脉络
Chat Paper
正在生成论文摘要