Joint Evidential K-Nearest Neighbor Classification
2022 IEEE 38TH INTERNATIONAL CONFERENCE ON DATA ENGINEERING (ICDE 2022)(2022)
摘要
The performance of K-nearest neighbor (K-NN) classification depends significantly on the searched neighborhoods of test samples, namely, the neighborhood size K and the used distance metric. For the two issues, many methods either to acquire the adaptive K or to learn a variant metric have been presented and yielded appropriate performance. However, most of the existing methods ignore the fact that these two factors can be jointly learned. Besides, nearly all the metric learning methods aim to shrink intra-class distance while expanding inter-class distance. In this way, embedding the learned metric directly into the K-NN does not efficiently improve its accuracy. To address these issues, we propose a joint K-NN algorithm with the help of evidence theory, optimizing the joint learning of adaptive K and distance matrix based on the feedback from error function. Ablation study demonstrates the performance improvement from the joint learning, and comparison experiments on real-world datasets show that our approach consumes competitive running time and achieves better performance than other state-of-the-art algorithms.
更多查看译文
关键词
neighborhood size,distance metric,metric learning methods,intra-class distance,inter-class distance,joint K-NN algorithm,joint learning,distance matrix,joint evidential K-nearest neighbor classification,variant metric,evidence theory,ablation study
AI 理解论文
溯源树
样例
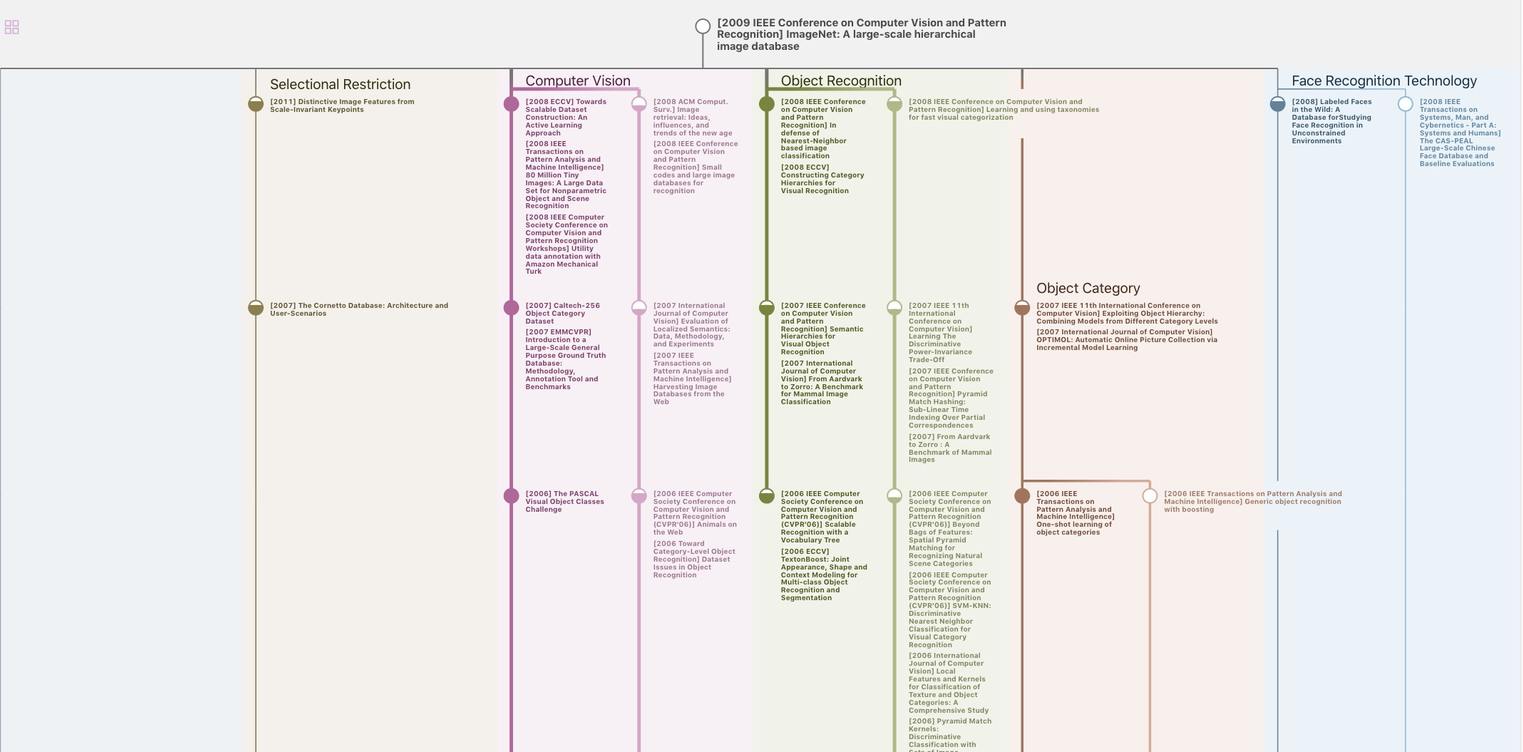
生成溯源树,研究论文发展脉络
Chat Paper
正在生成论文摘要