Self-reconstructive evidential clustering for high-dimensional data
2022 IEEE 38TH INTERNATIONAL CONFERENCE ON DATA ENGINEERING (ICDE 2022)(2022)
摘要
Although many algorithms have been presented to tackle the curse of dimensionality in high-dimensional clustering, most of these algorithms require prior knowledge of the number of clusters. Besides, these existing algorithms create only a hard or fuzzy partition for high-dimensional objects, which are often located in highly overlapping areas. The adoption of hard/fuzzy partition ignores the ambiguity in the assignment of objects and may lead to performance degradation. To address these issues, we propose a novel self-reconstructive evidential clustering (SREC) algorithm. After learning the correlations between objects from a self-reconstruction process, SREC provides a human-readable chart. Through this chart, users can select several objects existing in the dataset as the cluster centers, instead of just detecting the number of clusters. Under the framework of evidence theory, SREC derives a more flexible credal partition that improves the fault tolerance of clustering. Ablation study demonstrates the benefits of the self-reconstruction and evidence theory. Comparison experiments on real-world datasets show that SREC consumes competitive running time and performs better than other state-of-the-art algorithms. We also apply SREC in a realworld application scenario to illustrate the rationality of selecting cluster centers by human intervention.
更多查看译文
关键词
high-dimensional data,high-dimensional clustering,SREC,human-readable chart,cluster centers,evidence theory,credal partition,self-reconstructive evidential clustering algorithm
AI 理解论文
溯源树
样例
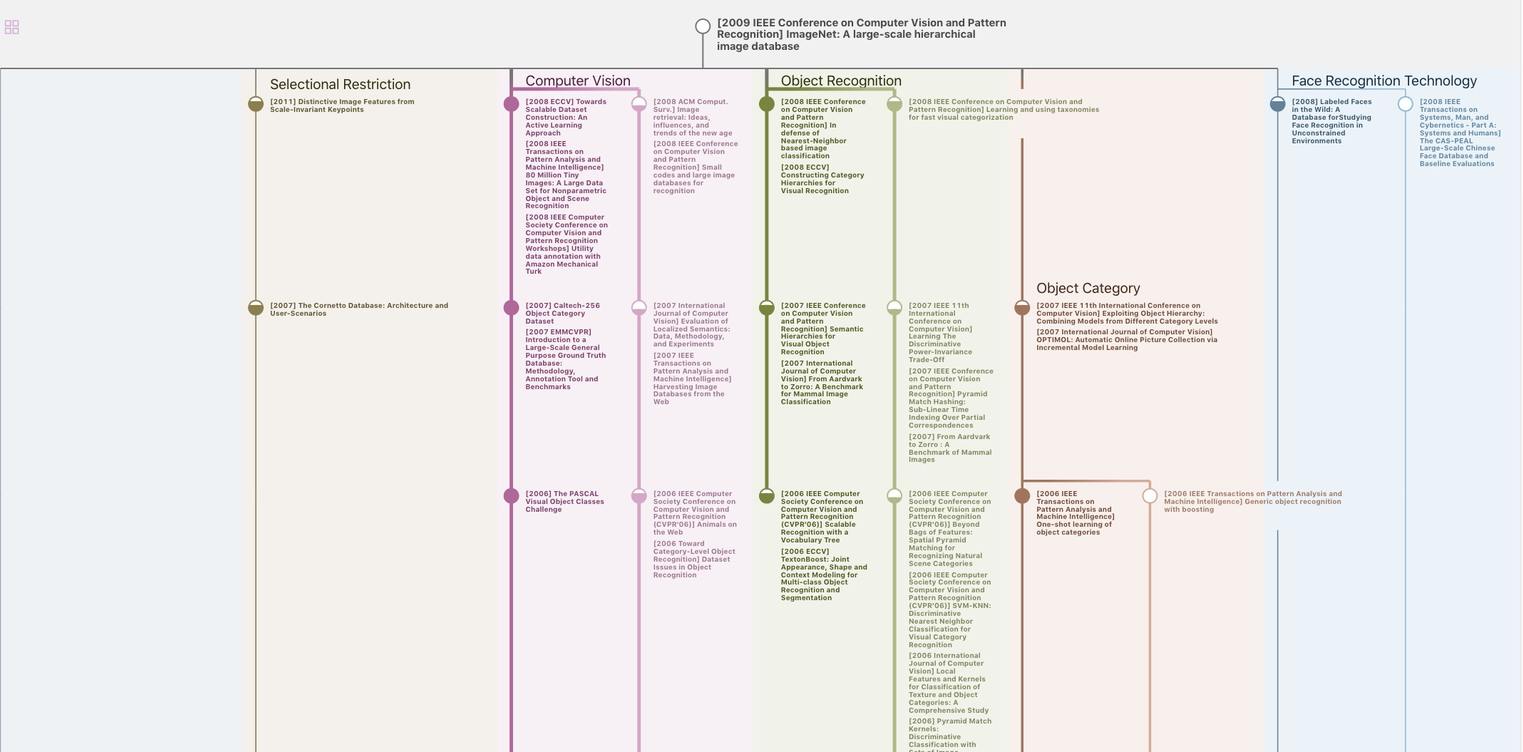
生成溯源树,研究论文发展脉络
Chat Paper
正在生成论文摘要