Triton join: Efficiently Scaling to a Large loin State on GPUs with Fast Interconnects
PROCEEDINGS OF THE 2022 INTERNATIONAL CONFERENCE ON MANAGEMENT OF DATA (SIGMOD '22)(2022)
摘要
Database management systems are facing growing data volumes. Previous research suggests that GPUs are well-equipped to quickly process joins and similar stateful operators, as GPUs feature high-bandwidth on-board memory. However, GPUs cannot scale joins to large data volumes due to two limiting factors: (1) large state does not lit into the on-board memory, and (2) spilling state to main memory is constrained by the interconnect bandwidth. Thus, CPUs are often the better choice for scalable data processing. In this paper, we propose a new join algorithm that scales to large data volumes by taking advantage of fast interconnect& Fast interconnects such as NVLink 2.0 are a new technology that connect the GPU to main memory at a high bandwidth, and thus enable us to design our join to efficiently spill its state. Our evaluation shows that our Triton join outperforms a no-partitioning hash join by more than 100x on the same GPU, and a radix-partitioned join on the CPU by up to 2.5x. As a result, GPU-enabled DBMSs are able to scale beyond the CPU memory capacity.
更多查看译文
关键词
Modern hardware, GPU, data transfer bottleneck, out-of-core, TLB
AI 理解论文
溯源树
样例
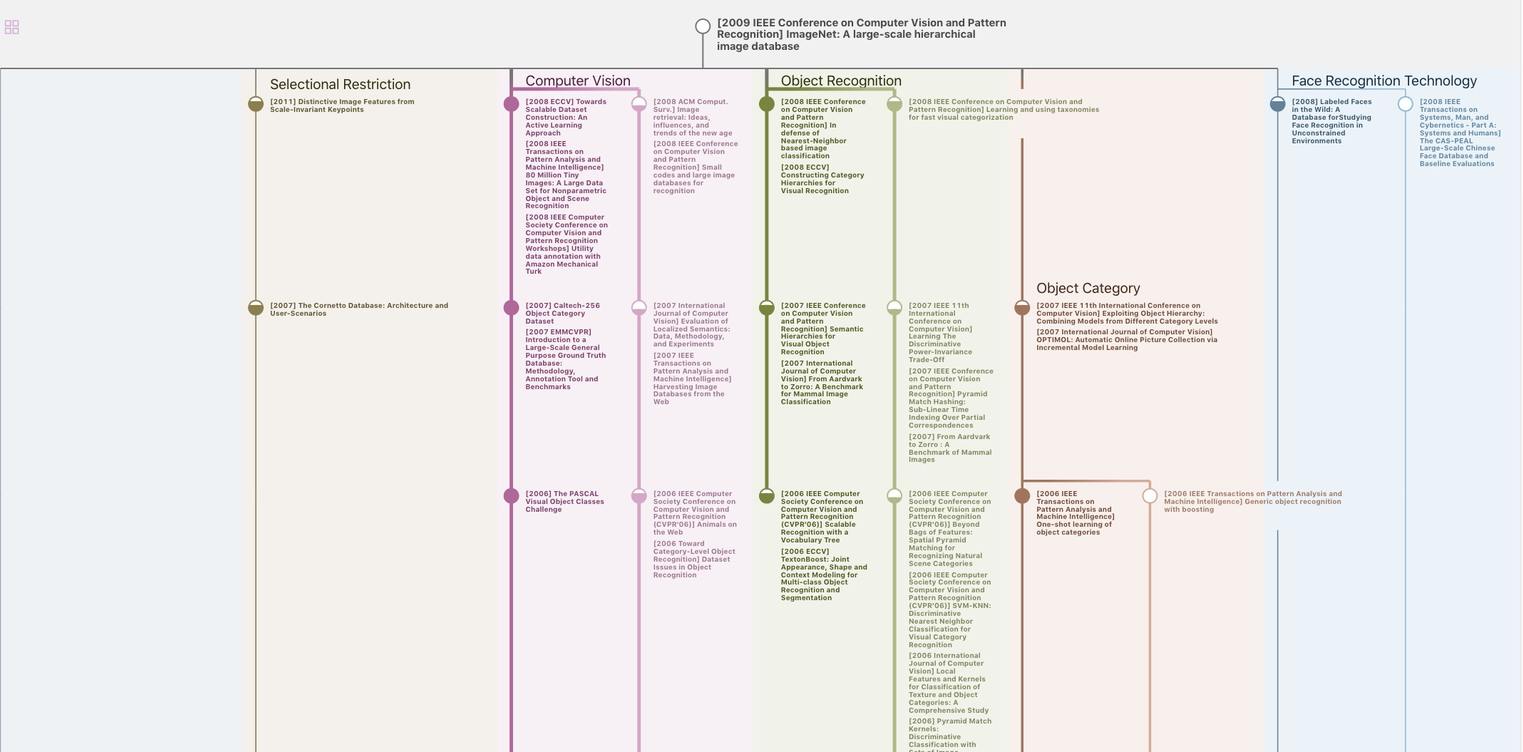
生成溯源树,研究论文发展脉络
Chat Paper
正在生成论文摘要