AI in 5G: The Case of Online Distributed Transfer Learning over Edge Networks
IEEE Conference on Computer Communications (INFOCOM)(2022)
摘要
Transfer learning does not train from scratch but leverages existing models to help train the new model of better accuracy. Unfortunately, realizing transfer learning in distributed cloud-edge networks faces critical challenges such as online training, uncertain network environments, time-coupled control decisions, and the balance between resource consumption and model accuracy. We formulate distributed transfer learning as a non-linear mixed-integer program of long-term cost optimization. We design polynomial-time online algorithms by exploiting the real-time trade-off between preserving previous decisions and applying new decisions, based on primal-dual one-shot solutions for each single time slot. While orchestrating model placement, data dispatching, and inference aggregation, our approach produces new models via combining the existing offline models and the online models being trained using weights adaptively updated based on inference upon data samples that dynamically arrive. Our approach provably incurs the number of inference mistakes no greater than a constant times that of the single best model in hindsight, and achieves a constant competitive ratio for the total cost. Evaluations have confirmed the superior performance of our approach compared to alternatives on real-world traces.
更多查看译文
关键词
edge networks,transfer,ai
AI 理解论文
溯源树
样例
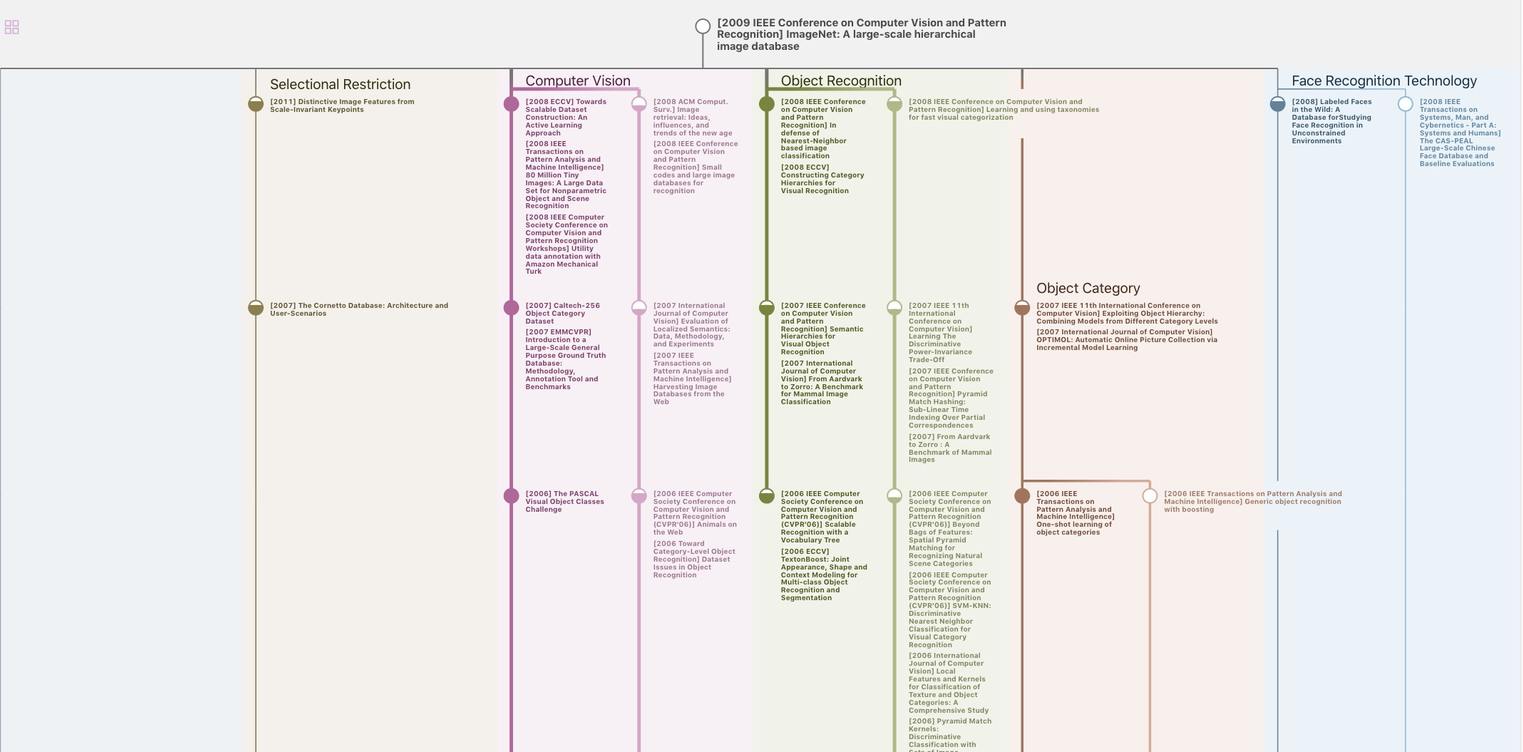
生成溯源树,研究论文发展脉络
Chat Paper
正在生成论文摘要