Mirror Descent Policy Optimization
International Conference on Learning Representations (ICLR)(2020)
摘要
Mirror descent (MD), a well-known first-order method in constrained convex
optimization, has recently been shown as an important tool to analyze
trust-region algorithms in reinforcement learning (RL). However, there remains
a considerable gap between such theoretically analyzed algorithms and the ones
used in practice. Inspired by this, we propose an efficient RL algorithm,
called mirror descent policy optimization (MDPO). MDPO iteratively
updates the policy by approximately solving a trust-region problem, whose
objective function consists of two terms: a linearization of the standard RL
objective and a proximity term that restricts two consecutive policies to be
close to each other. Each update performs this approximation by taking multiple
gradient steps on this objective function. We derive on-policy and off-policy variants of MDPO, while emphasizing important design choices
motivated by the existing theory of MD in RL. We highlight the connections
between on-policy MDPO and two popular trust-region RL algorithms: TRPO and
PPO, and show that explicitly enforcing the trust-region constraint is in fact
not a necessity for high performance gains in TRPO. We then show how the
popular soft actor-critic (SAC) algorithm can be derived by slight
modifications of off-policy MDPO. Overall, MDPO is derived from the MD
principles, offers a unified approach to viewing a number of popular RL
algorithms, and performs better than or on-par with TRPO, PPO, and SAC in a
number of continuous control tasks. Code is available at
.
更多查看译文
关键词
optimization,mirror,policy
AI 理解论文
溯源树
样例
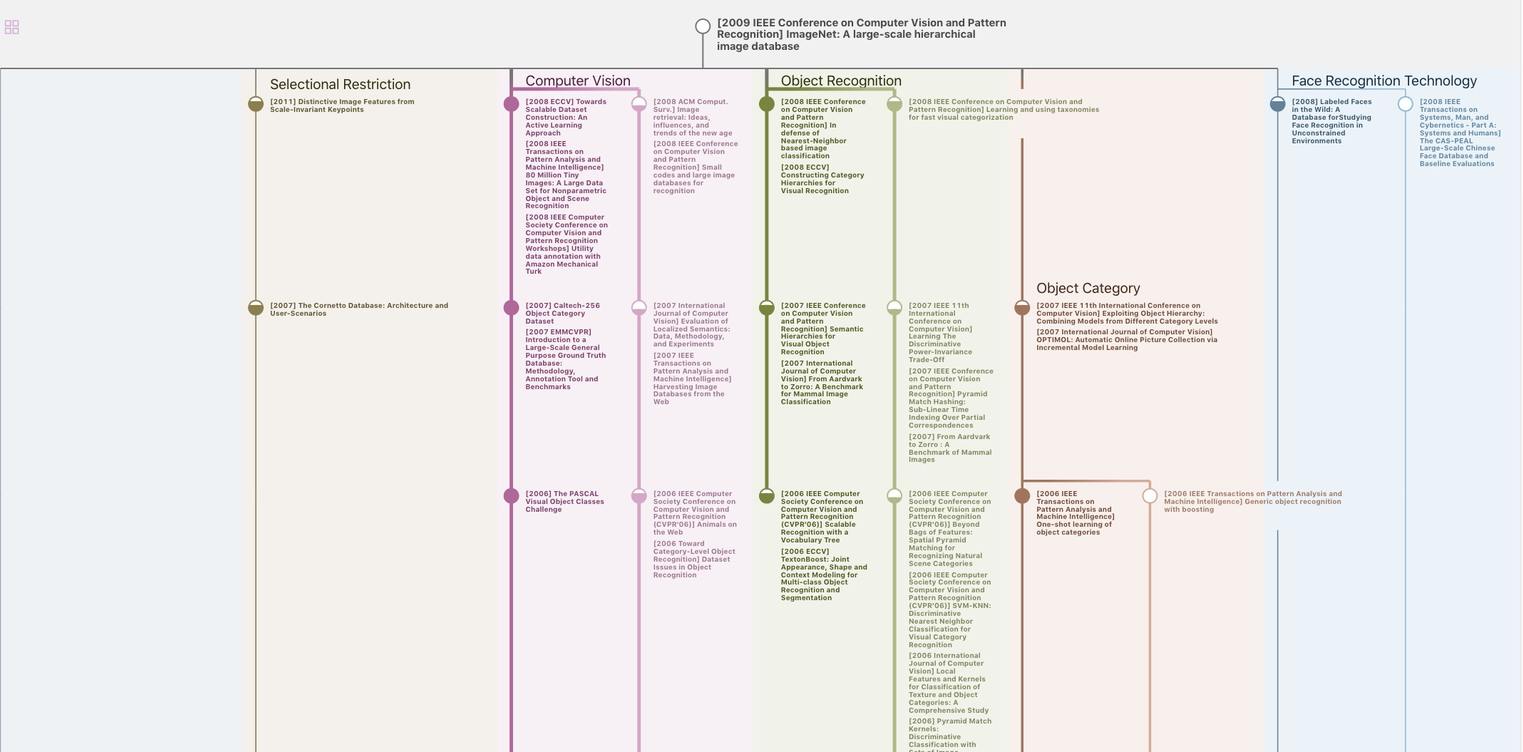
生成溯源树,研究论文发展脉络
Chat Paper
正在生成论文摘要