Cerebral Microbleeds Detection Using a 3D Feature Fused Region Proposal Network with Hard Sample Prototype Learning
MEDICAL IMAGE COMPUTING AND COMPUTER ASSISTED INTERVENTION, MICCAI 2022, PT I(2022)
摘要
Cerebral Microbleeds (CMBs) are chronic deposits of small blood products in the brain tissues, which have explicit relation to cerebrovascular diseases, including cognitive decline, intracerebral hemorrhage, and cerebral infarction. However, manual detection of the CMBs is a time-consuming and error-prone process because of the sparse and tiny properties of CMBs. Also, the detection of CMBs is commonly affected by the existence of many CMB mimics that cause a high false-positive rate (FPR), such as calcification, iron depositions, and pial vessels. This paper proposes an efficient single-stage deep learning framework for the automatic detection of CMBs. The structure consists of a 3D U-Net employed as a backbone and Region Proposal Network (RPN). To significantly reduce the FPs, we developed a new scheme, containing Feature Fusion Module (FFM) that greatly detects small candidates utilizing contextual information and Hard Sample Prototype Learning (HSPL) that mines CMB mimics and generates additional loss term called concentration loss using Convolutional Prototype Learning (CPL). The proposed network utilizes Susceptibility-Weighted Imaging (SWI) and phase images as 3D input to efficiently capture 3D information. The proposed model was trained and tested using data containing 114 subjects with 365 CMBs. The performance of vanilla RPN shows a sensitivity of 93.33% and an average number of false positives per subject (FPavg) of 14.73. In contrast, the proposed Feature Fused RPN that utilizes the HSPL outperforms the vanilla RPN and achieves a sensitivity of 94.66% and FP(avg )of 0.86.
更多查看译文
关键词
Cerebral Microbleeds, Deep learning, Region Proposal Network, Prototype learning, Cerebral small vessel disease
AI 理解论文
溯源树
样例
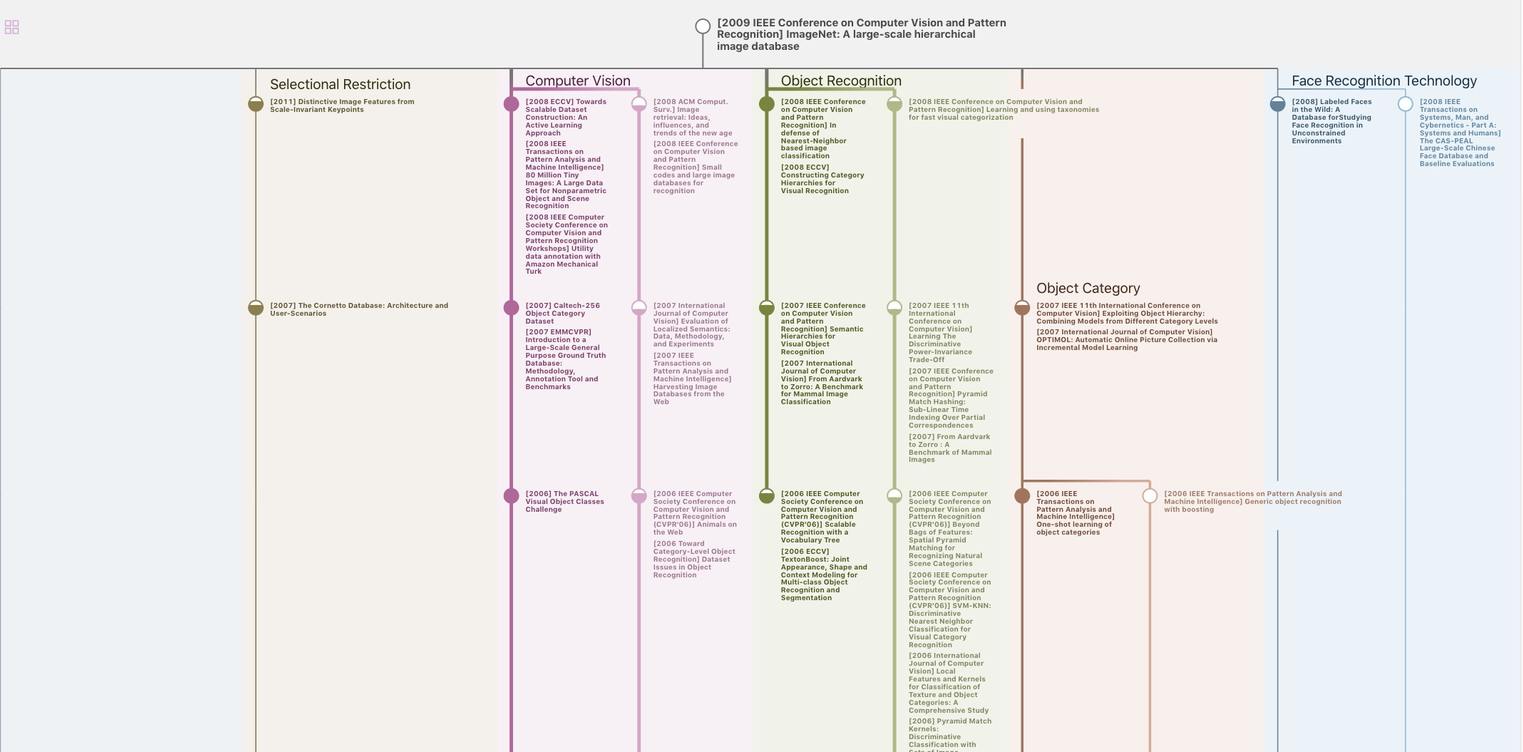
生成溯源树,研究论文发展脉络
Chat Paper
正在生成论文摘要