Streaming Session-Based Recommendation: When Graph Neural Networks meet the Neighborhood
ACM Conference On Recommender Systems(2022)
摘要
BSTRACTFrequent updates and model retraining are important in various application areas of recommender systems, e.g., news recommendation. Moreover, in such domains, we may not only face the problem of dealing with a constant stream of new data, but also with anonymous users, leading to the problem of streaming session-based recommendation (SSR). Such problem settings have attracted increased interest in recent years, and different deep learning architectures were proposed that support fast updates of the underlying prediction models when new data arrive. In a recent paper, a method based on Graph Neural Networks (GNN) was proposed as being superior than previous methods for the SSR problem. The baselines in the reported experiments included different machine learning models. However, several earlier studies have shown that often conceptually simpler methods, e.g., based on nearest neighbors, can be highly effective for session-based recommendation problems. In this work, we report a similar phenomenon for the streaming configuration. We first reproduce the results of the mentioned GNN method and then show that simpler methods are able to outperform this complex state-of-the-art neural method on two datasets. Overall, our work points to continued methodological issues in the academic community, e.g., in terms of the choice of baselines and reproducibility.1
更多查看译文
AI 理解论文
溯源树
样例
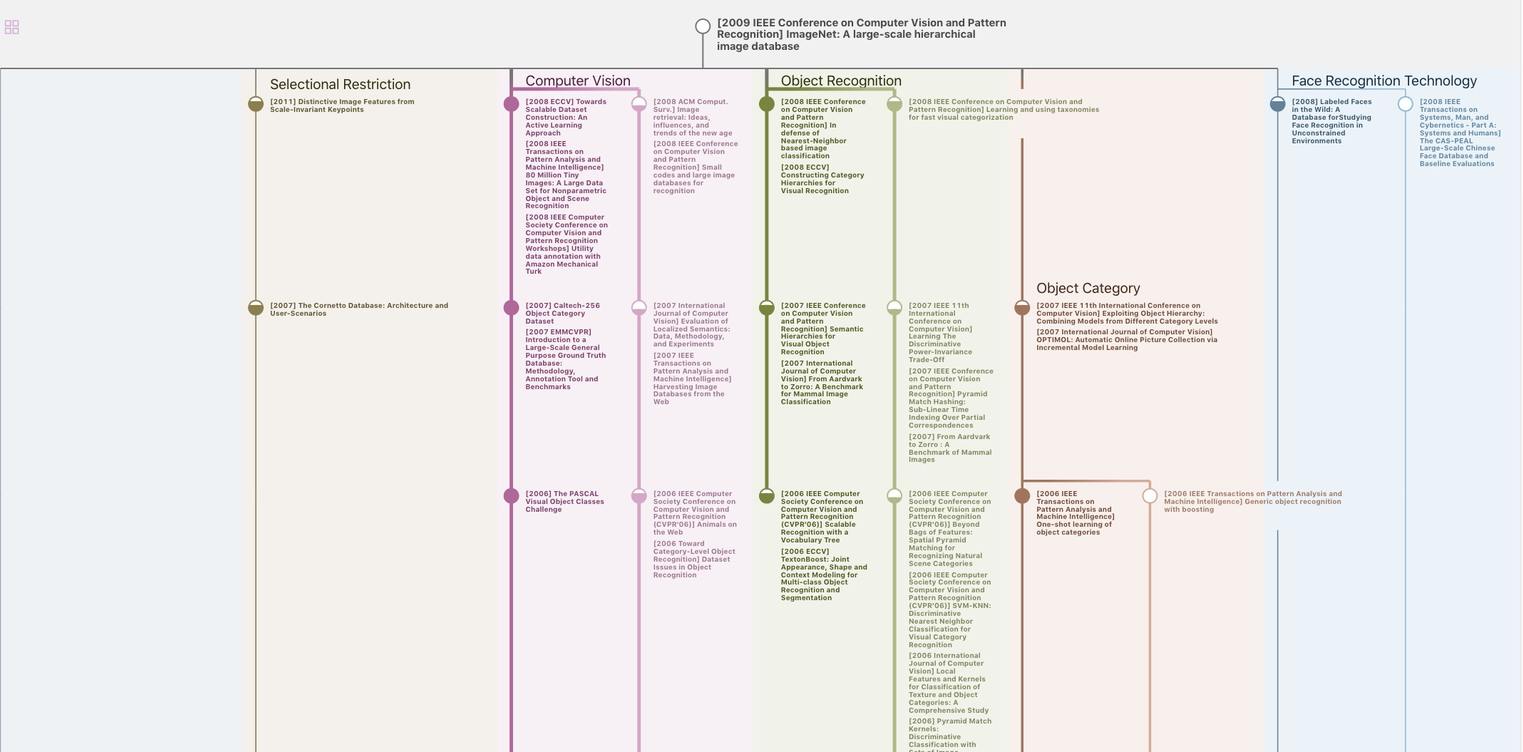
生成溯源树,研究论文发展脉络
Chat Paper
正在生成论文摘要