Multi-objective evolutionary spatio-temporal forecasting of air pollution
Future Generation Computer Systems(2022)
摘要
Nowadays, air pollution forecasting modeling is vital to achieve an increase in air quality, allowing an improvement of ecosystems and human health. It is important to consider the spatial characteristics of the data, as they allow us to infer predictions in those areas for which no information is available. In the current literature, there are a large number of proposals for spatio-temporal air pollution forecasting. In this paper we propose a novel spatio-temporal approach based on multi-objective evolutionary algorithms for the identification of multiple non-dominated linear regression models and their combination in an ensemble learning model for air pollution forecasting. The ability of multi-objective evolutionary algorithms to find a Pareto front of solutions is used to build multiple forecast models geographically distributed in the area of interest. The proposed method has been applied for one-week NO2 prediction in southeastern Spain and has obtained promising results in statistical comparison with other approaches such as the union of datasets or the interpolation of the predictions for each monitoring station. The validity of the proposed spatio-temporal approach is thus demonstrated, opening up a new field in air pollution engineering.
更多查看译文
关键词
Spatio-temporal forecasting,Air pollution,Multi-objective optimization,Evolutionary algorithms,Machine learning,Ensemble learning
AI 理解论文
溯源树
样例
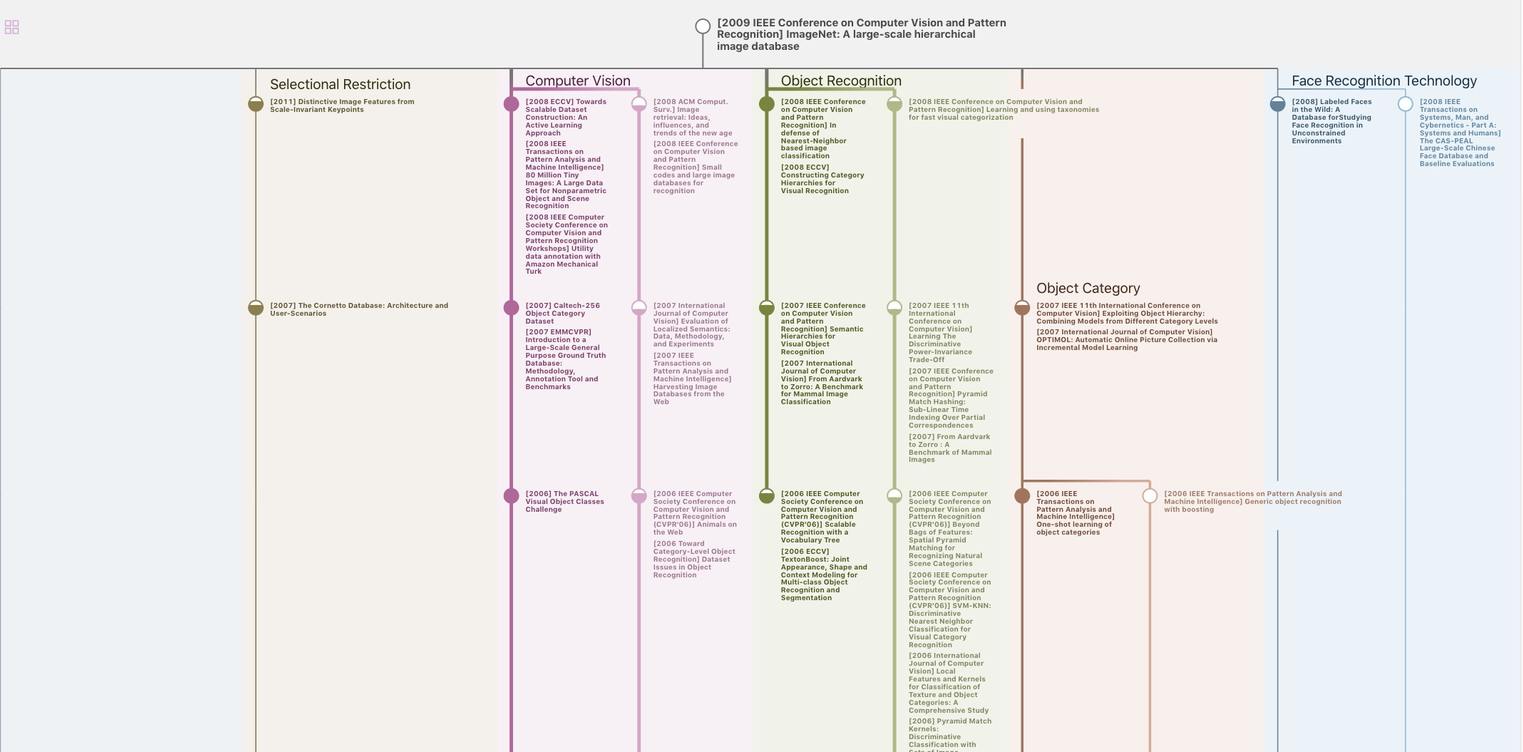
生成溯源树,研究论文发展脉络
Chat Paper
正在生成论文摘要