Interactively Assessing Disentanglement in GANs
COMPUTER GRAPHICS FORUM(2022)
摘要
Generative adversarial networks (GAN) have witnessed tremendous growth in recent years, demonstrating wide applicability in many domains. However, GANs remain notoriously difficult for people to interpret, particularly for modern GANs capable of generating photo-realistic imagery. In this work we contribute a visual analytics approach for GAN interpretability, where we focus on the analysis and visualization of GAN disentanglement. Disentanglement is concerned with the ability to control content produced by a GAN along a small number of distinct, yet semantic, factors of variation. The goal of our approach is to shed insight on GAN disentanglement, above and beyond coarse summaries, instead permitting a deeper analysis of the data distribution modeled by a GAN. Our visualization allows one to assess a single factor of variation in terms of groupings and trends in the data distribution, where our analysis seeks to relate the learned representation space of GANs with attribute-based semantic scoring of images produced by GANs. Through use-cases, we show that our visualization is effective in assessing disentanglement, allowing one to quickly recognize a factor of variation and its overall quality. In addition, we show how our approach can highlight potential dataset biases learned by GANs.
更多查看译文
关键词
disentanglement
AI 理解论文
溯源树
样例
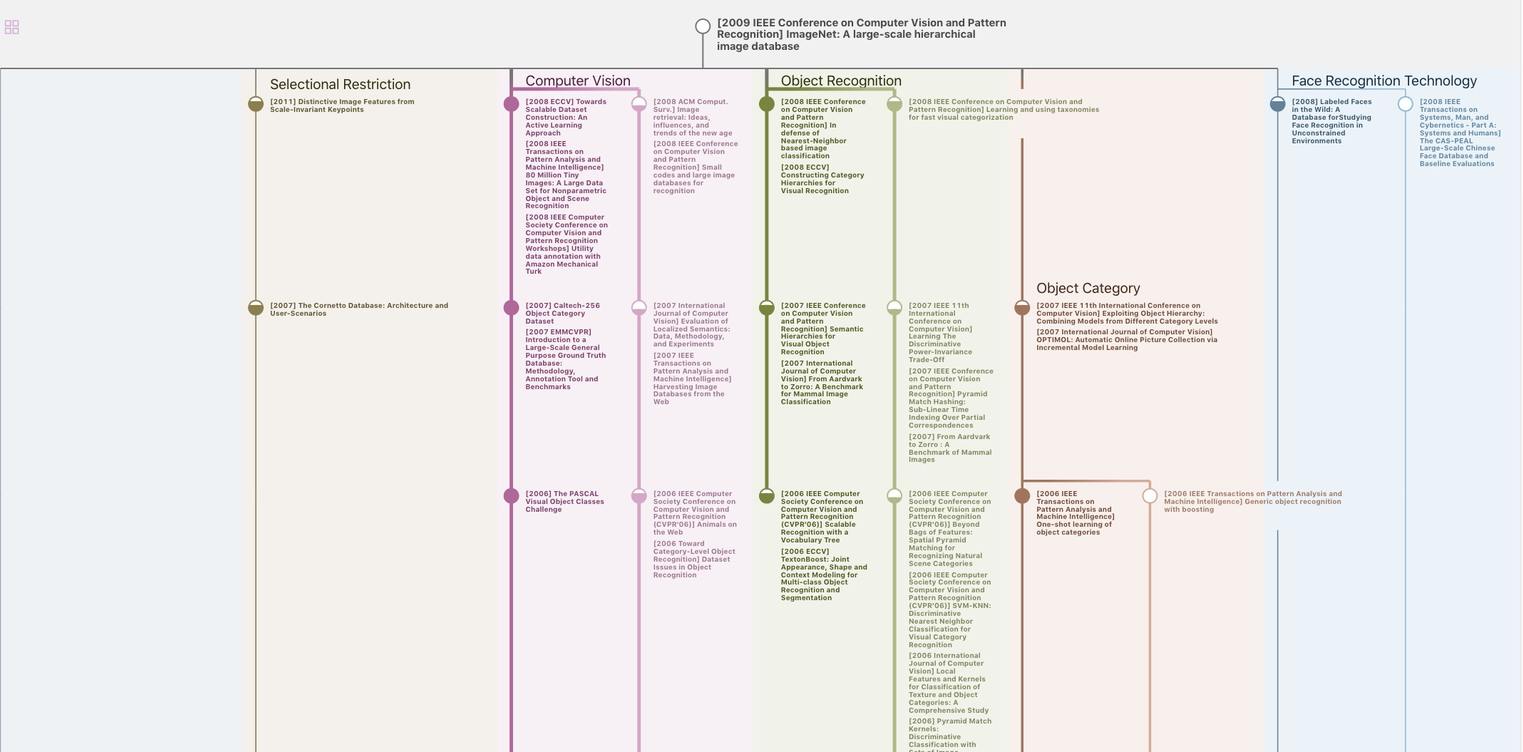
生成溯源树,研究论文发展脉络
Chat Paper
正在生成论文摘要