A Vertex-Directed Evolutionary Algorithm for Multiobjective Endmember Estimation
IEEE TRANSACTIONS ON GEOSCIENCE AND REMOTE SENSING(2022)
摘要
Hyperspectral unmixing including endmember extraction and abundance estimation has been investigated successively in recent years due to increasingly hyperspectral processing requirements. As one type of decision after solution paradigm, the multiobjective endmember estimation (MoEE) method is able to obtain a set of Pareto optimal solutions, thus providing a wealth of information to determine the most representative endmembers. In addition, multiobjective optimization methods also have the characteristics of flexible modeling, excellent global convergence, commendable adaptability, and so on. However, the evolutionary algorithms designed for this kind of method generally use little spatial-spectral information of the hyperspectral image. In this article, we delve into the memetic strategy by exploiting the topological structure of hyperspectral data in the high-dimensional space to establish a vertex-directed MoEE method, termed VD-MoEE. According to the frequently used linear mixture model, endmembers of hyperspectral images are generally distributed at the vertices of the hyperspectral data manifold. Therefore, we design a vertex-directed local search operator to guide the search direction of the individuals in evolutionary algorithms. Experimental results on synthetic and real datasets demonstrated that the proposed VD-MOEE is able to achieve appealing performance in terms of solution selection and accuracy in comparison with several classic and state-of-the-art endmember estimation methods.
更多查看译文
关键词
Hyperspectral imaging, Estimation, Libraries, Pareto optimization, Manifolds, Evolutionary computation, Mixture models, Linear mixture model, multiobjective endmember estimation (MoEE), spatial-spectral information, topological structure, vertex-directed local search
AI 理解论文
溯源树
样例
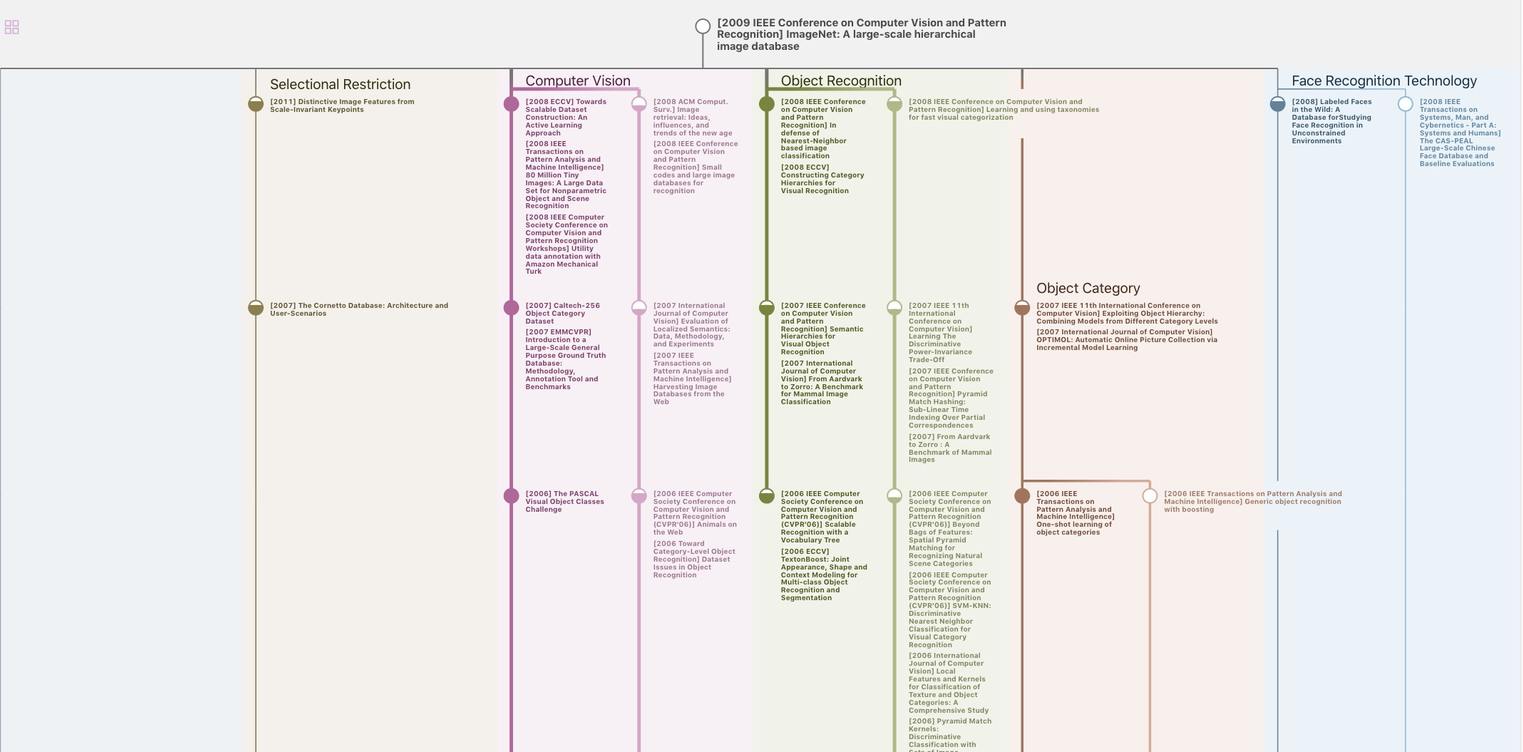
生成溯源树,研究论文发展脉络
Chat Paper
正在生成论文摘要