Fuzzy C-Means Clustering Algorithms with Weighted Membership and Distance
INTERNATIONAL JOURNAL OF UNCERTAINTY FUZZINESS AND KNOWLEDGE-BASED SYSTEMS(2022)
摘要
Fuzzy C-means (FCM) clustering algorithm is an important and popular clustering algorithm which is utilized in various application domains such as pattern recognition, machine learning, and data mining. Although this algorithm has shown acceptable performance in diverse problems, the current literature does not have studies about how they can improve the clustering quality of partitions with overlapping classes. The better the clustering quality of a partition, the better is the interpretation of the data, which is essential to understand real problems. This work proposes two robust FCM algorithms to prevent ambiguous membership into clusters. For this, we compute two types of weights: an weight to avoid the problem of overlapping clusters; and other weight to enable the algorithm to identify clusters of different shapes. We perform a study with synthetic datasets, where each one contains classes of different shapes and different degrees of overlapping. Moreover, the study considered real application datasets. Our results indicate such weights are effective to reduce the ambiguity of membership assignments thus generating a better data interpretation.
更多查看译文
关键词
Clustering algorithms, ambiguous membership, fuzzy C-means, weighting
AI 理解论文
溯源树
样例
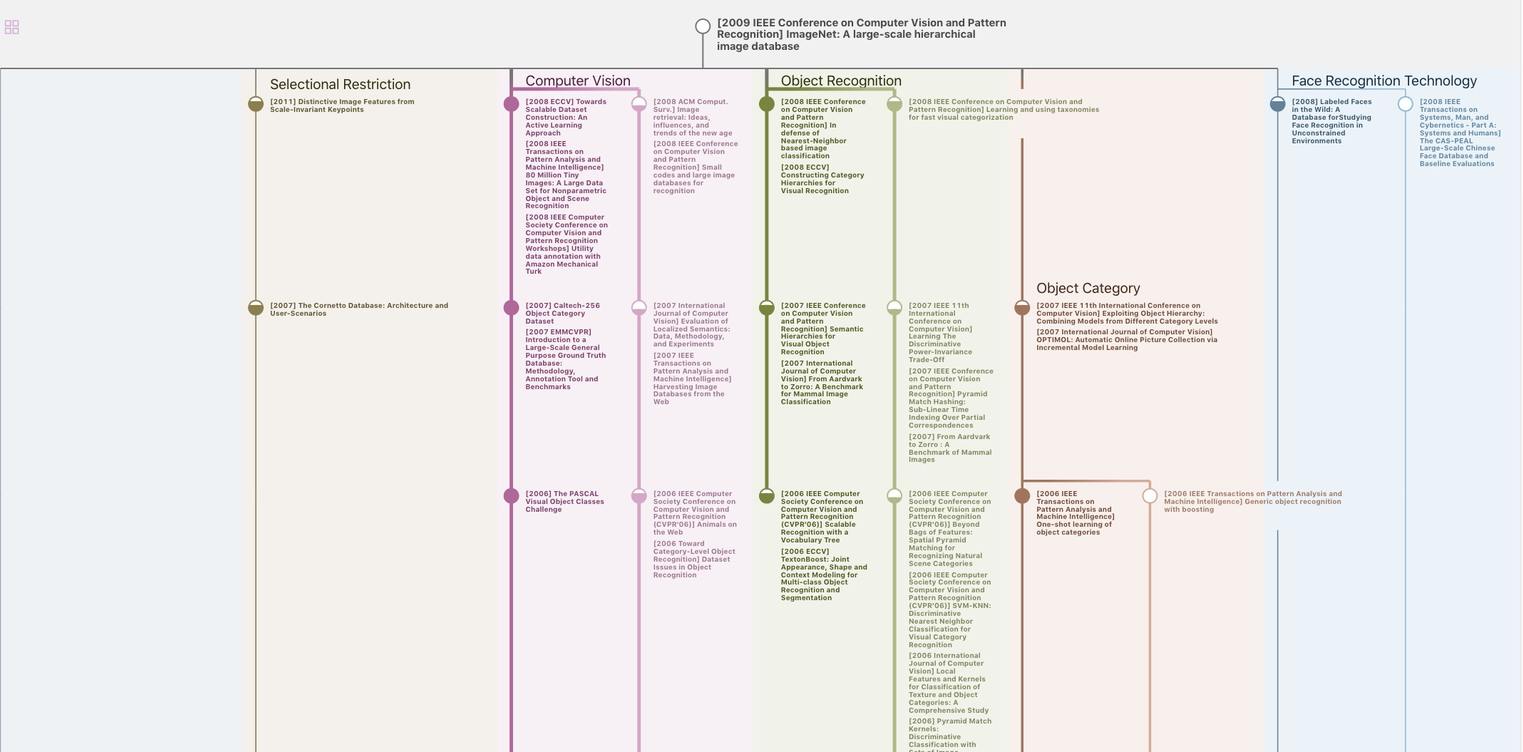
生成溯源树,研究论文发展脉络
Chat Paper
正在生成论文摘要