Graph Representation Learning for Gate Arrival Time Prediction
2022 ACM/IEEE 4th Workshop on Machine Learning for CAD (MLCAD)(2022)
摘要
An accurate estimate of the timing profile at different stages of the physical design flow allows for pre-emptive changes to the circuit, significantly reducing the design time and effort. In this work, a graph based deep regression model is utilized to predict the gate level arrival time of the timing paths of a circuit. Three scenarios for post routing prediction are considered: prediction after completing floorplanning, prediction after completing placement, and prediction after completing clock tree synthesis (CTS). A commercial static timing analysis (STA) tool is utilized to determine the mean absolute percentage error (MAPE) and the mean absolute error (MAE) for each scenario. Results obtained across all models trained on the complete dataset indicate that the proposed methodology outperforms the baseline errors produced by the commercial physical design tools with an average improvement of 61.58% in the MAPE score when predicting the post-routing arrival time after completing floorplanning and 13.53% improvement when predicting the post-routing arrival time after completing placement. Additional prediction scenarios are analyzed, where the complete dataset is further sub-divided based on the size of the circuits, which leads to an average improvement of 34.83% in the MAPE score as compared to the commercial tool for post-floorplanning prediction of the post-routing arrival time and 22.71% improvement for post-placement prediction of the post-routing arrival time.
更多查看译文
关键词
timing prediction,physical design,machine learning,graph convolutional networks
AI 理解论文
溯源树
样例
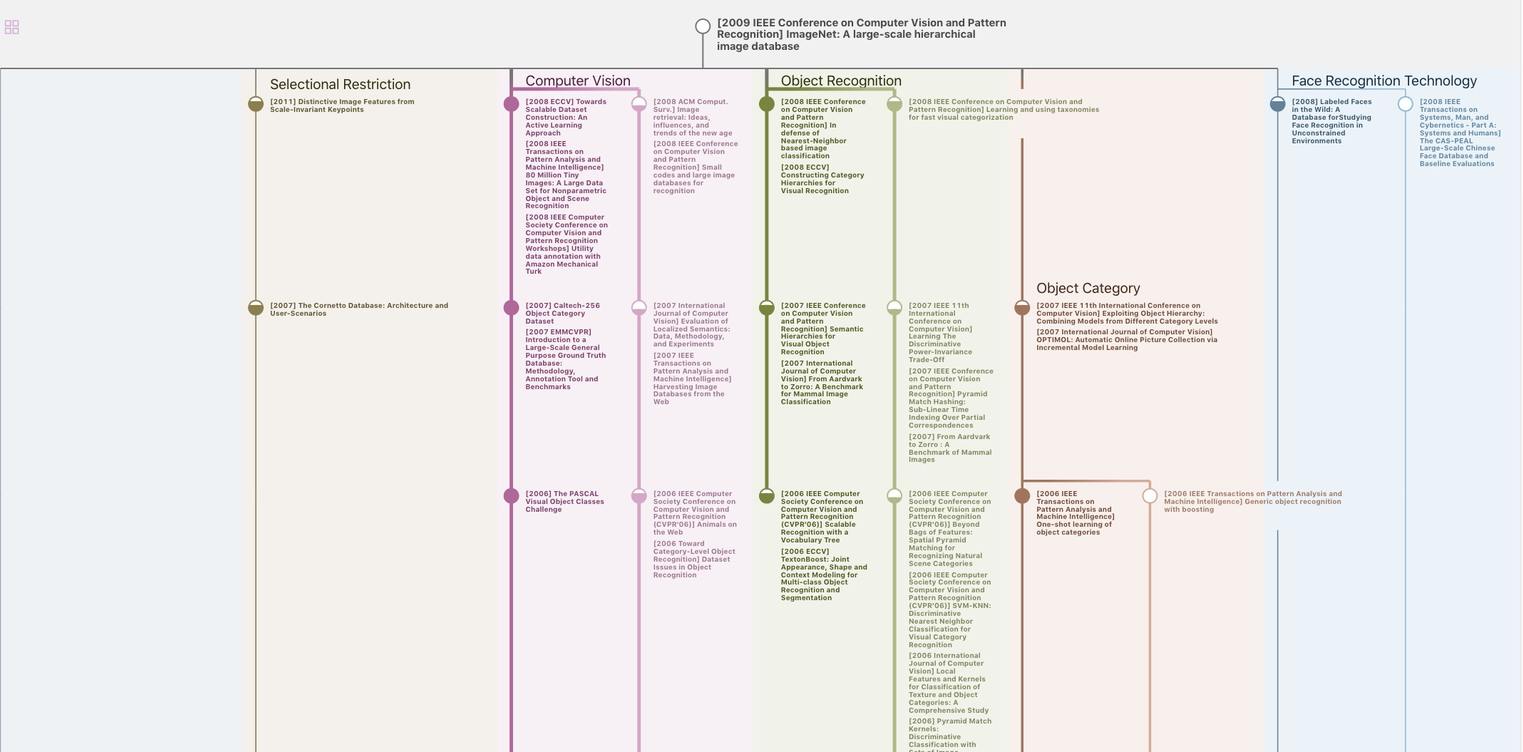
生成溯源树,研究论文发展脉络
Chat Paper
正在生成论文摘要