XT-PRAGGMA: Crosstalk Pessimism Reduction Achieved with GPU Gate-level Simulations and Machine Learning
2022 ACM/IEEE 4th Workshop on Machine Learning for CAD (MLCAD)(2022)
摘要
Accurate crosstalk-aware timing analysis is critical in nanometerscale process nodes. While today’s VLSI flows rely on static timing analysis (STA) techniques to perform crosstalk-aware timing signoff, these techniques are limited due to their static nature as they use imprecise heuristics such as arbitrary aggressor filtering and simplified delay calculations. This paper proposes XT-PRAGGMA, a tool that uses GPU-accelerated dynamic gate-level simulations and machine learning to eliminate false aggressors and accurately predict crosstalk-induced delta delays. XT-PRAGGMA reduces STA pessimism and provides crucial information to identify crosstalk-critical nets, which can be considered for accurate SPICE simulation before signoff. The proposed technique is fast (less than two hours to simulate 30,000 vectors on million-gate designs) and reduces falsely-reported total negative slack in timing signoff by 70%.
更多查看译文
关键词
• Hardware→Physicaldesign(EDA),MethodologiesforEDA,Static timing analysis,Timing analysis and sign-off,Simulation and emulation,Transition-based timing analysis
AI 理解论文
溯源树
样例
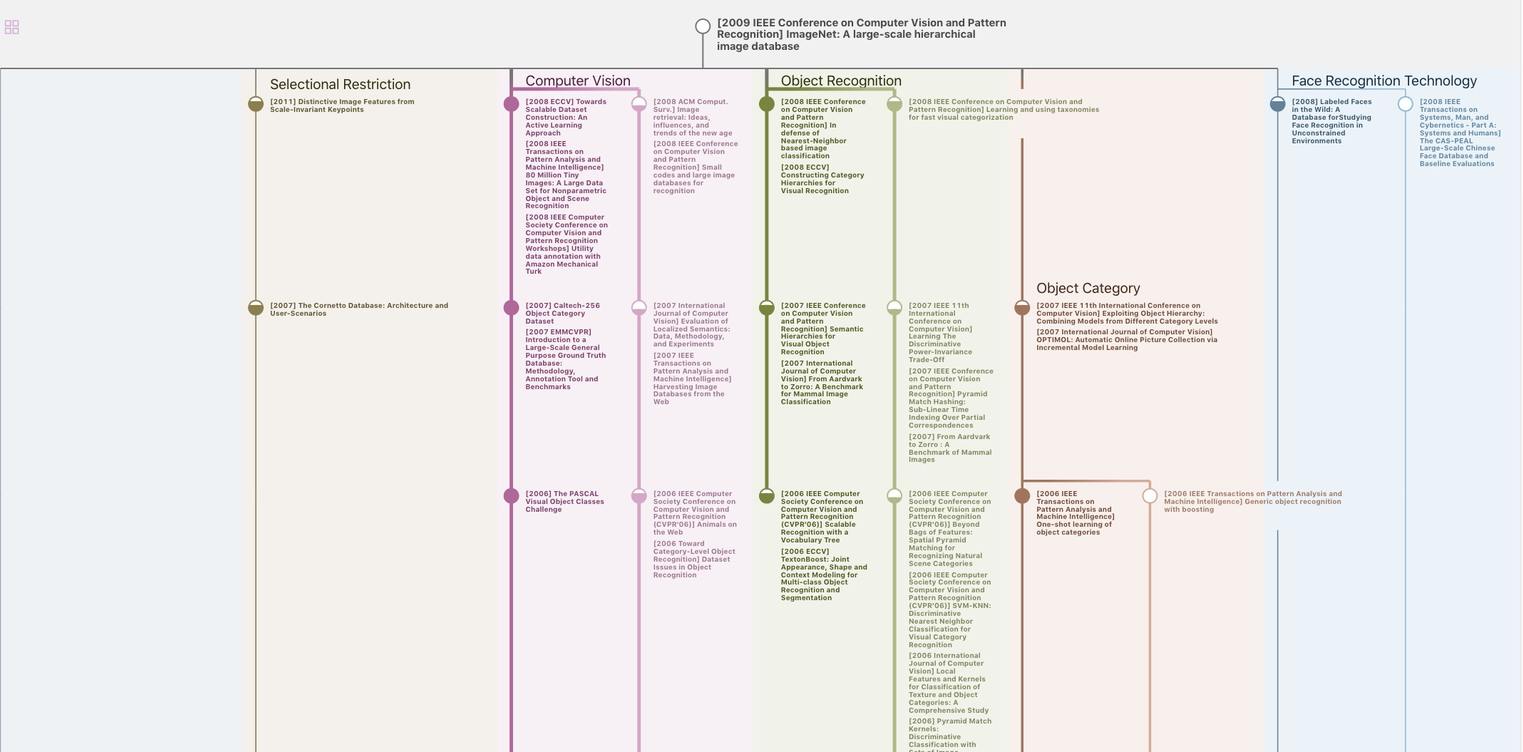
生成溯源树,研究论文发展脉络
Chat Paper
正在生成论文摘要