Deep Learning-Based Layer Identification of 2D Nanomaterials
Coatings(2022)
摘要
Two-dimensional (2D) nanomaterials exhibit unique properties due to their low dimensionality, which has led to great potential for applications in biopharmaceuticals, aerospace, energy storage, mobile communications and other fields. Today, 2D nanomaterials are often prepared and exfoliated by a combination of mechanical and manual methods, which makes the production of 2D nanomaterials inefficient and prevents standardized and industrialized manufacturing. Recent breakthroughs in semantic segmentation techniques based on deep learning have enabled the accurate identification and segmentation of atomic layers of 2D nanomaterials using optical microscopy. In this study, we analyzed in detail sixteen semantic segmentation models that perform well on public datasets and apply them to the layer identification and segmentation of graphene and molybdenum disulfide. Furthermore, we improved the U2-Net† model to obtain the best overall performance, namely 2DU2-Net†. The accuracy of the 2DU2-Net† model was 99.03%, the kappa coefficient was 95.72%, the dice coefficient was 96.97%, and the average cross–merge ratio was 94.18%. Meanwhile, it also had good performance in terms of computation, number of parameters, inference speed and generalization ability. The results show that deep learning-based semantic segmentation methods can greatly improve efficiency and replace most manual operations, and different types of semantic segmentation methods can be adapted to different properties of 2D nanomaterials, thus promoting the research and application of 2D nanomaterials.
更多查看译文
关键词
2D nanomaterials, deep learning, convolutional neural networks, image segmentation, 2DU(2)-Net(dagger), semantic segmentation
AI 理解论文
溯源树
样例
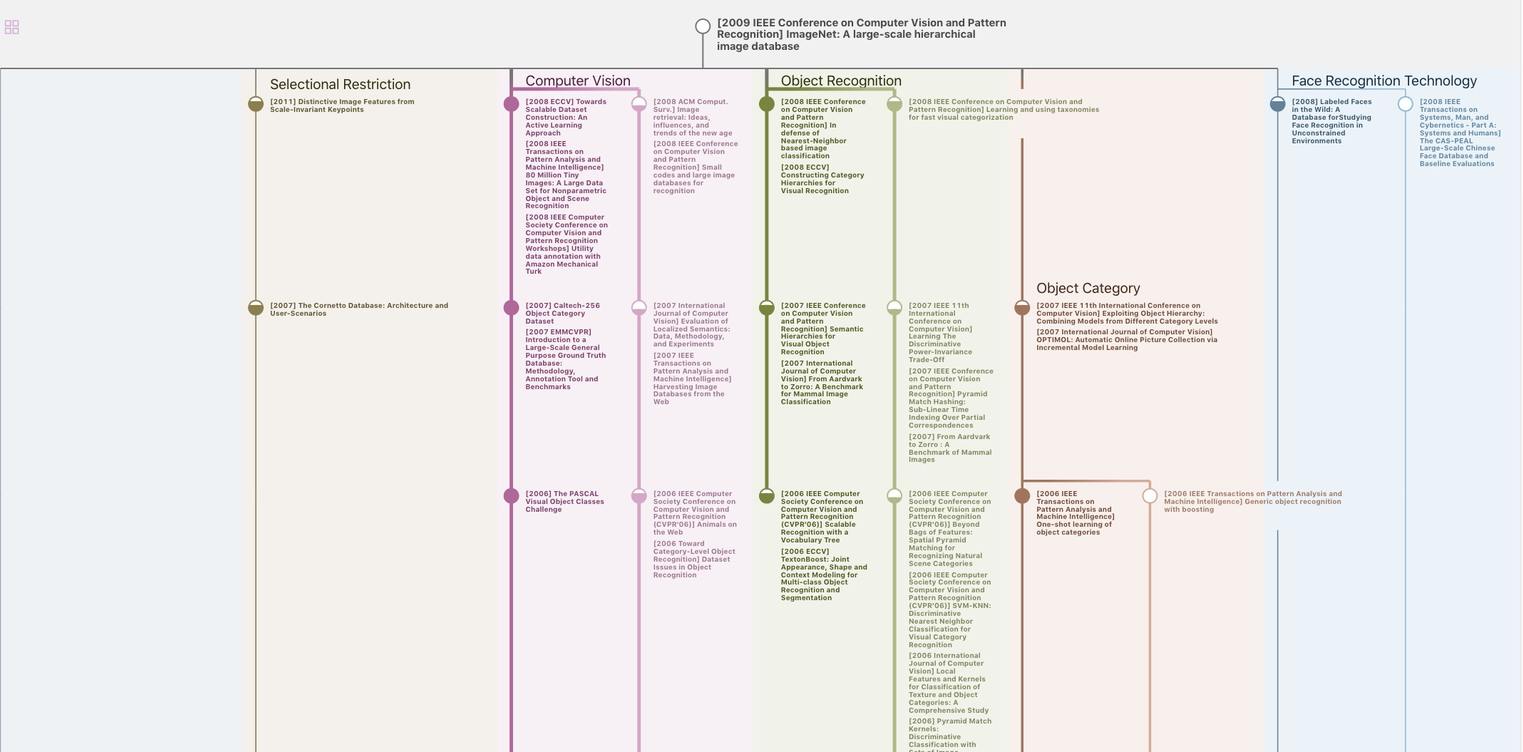
生成溯源树,研究论文发展脉络
Chat Paper
正在生成论文摘要