Autoregressive Uncertainty Modeling for 3D Bounding Box Prediction.
European Conference on Computer Vision(2022)
摘要
3D bounding boxes are a widespread intermediate representation in many computer vision applications. However, predicting them is a challenging task, largely due to partial observability, which motivates the need for a strong sense of uncertainty. While many recent methods have explored better architectures for consuming sparse and unstructured point cloud data, we hypothesize that there is room for improvement in the modeling of the output distribution and explore how this can be achieved using an autoregressive prediction head. Additionally, we release a simulated dataset, COB-3D, which highlights new types of ambiguity that arise in real-world robotics applications, where 3D bounding box prediction has largely been underexplored. We propose methods for leveraging our autoregressive model to make high confidence predictions and meaningful uncertainty measures, achieving strong results on SUN-RGBD, Scannet, KITTI, and our new dataset (Code and dataset are available at bbox.yuxuanliu.com.).
更多查看译文
关键词
3D bounding boxes,3D bounding box estimation,3D object detection,Autoregressive models,Uncertainty modeling
AI 理解论文
溯源树
样例
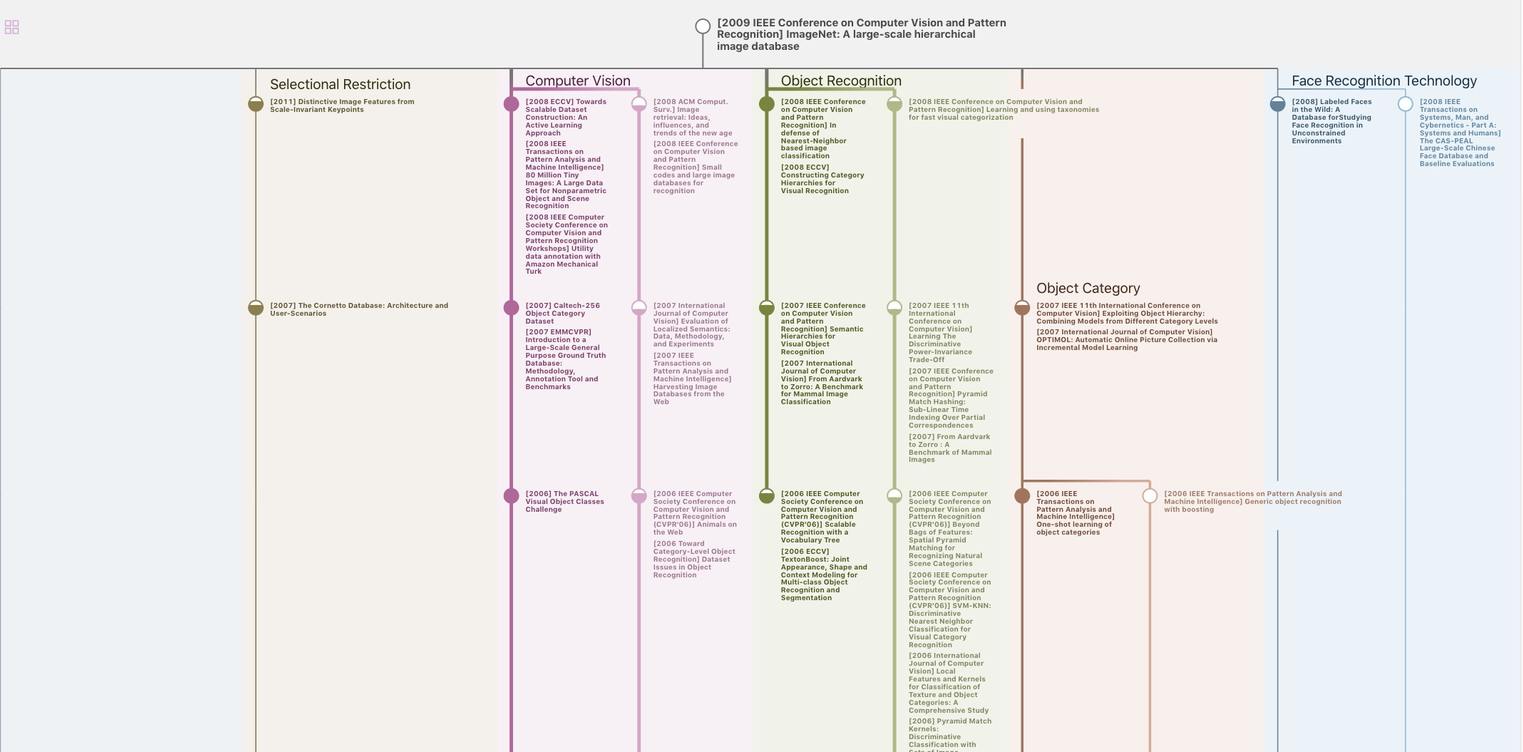
生成溯源树,研究论文发展脉络
Chat Paper
正在生成论文摘要