Ventricular Fibrillation Characterization for Sudden Cardiac Death Risk Prediction based on Wavelet Analysis
2022 IEEE International Symposium on Medical Measurements and Applications (MeMeA)(2022)
摘要
Sudden cardiac death (SCD) is one of the leading causes of death in the world, causing more than 50% of deaths related to cardiovascular diseases. Ventricular fibrillation is the most common feature associated with SCD. This work proposes a non-linear prediction method for Ventricular Fibrillation in ECG signals based on features consistent with this heart disease. Signals from two different groups were used a group of healthy subjects and a group of patients that developed Ventricular Fibrillation minutes before dying. This method is elaborated by extracting ECG features in the QT intervals and then calculating the entropy in the Wavelet Packet Decomposition terminal nodes. Features such as heart rate and the probability that the heart beats with a time less than 50 milliseconds, known as pNN50 are also extracted and they are statistically analyzed to determine their relevance when predicting Ventricular Fibrillation. A machine learning model was trained by Random Forest using these features, and it is particularly valid in predicting heart disease. The experimental results show that the proposed method reveals some frequency ranges that may be more relevant for features extraction and is promising for Ventricular Fibrillation prediction in ECG signals allowing prediction with a greater time frame, accuracy, and precision than those obtained in the state of the art literature.
更多查看译文
关键词
Ventricular Fibrillation,ECG,QT intervals,entropy,Wavelet Packet Decomposition
AI 理解论文
溯源树
样例
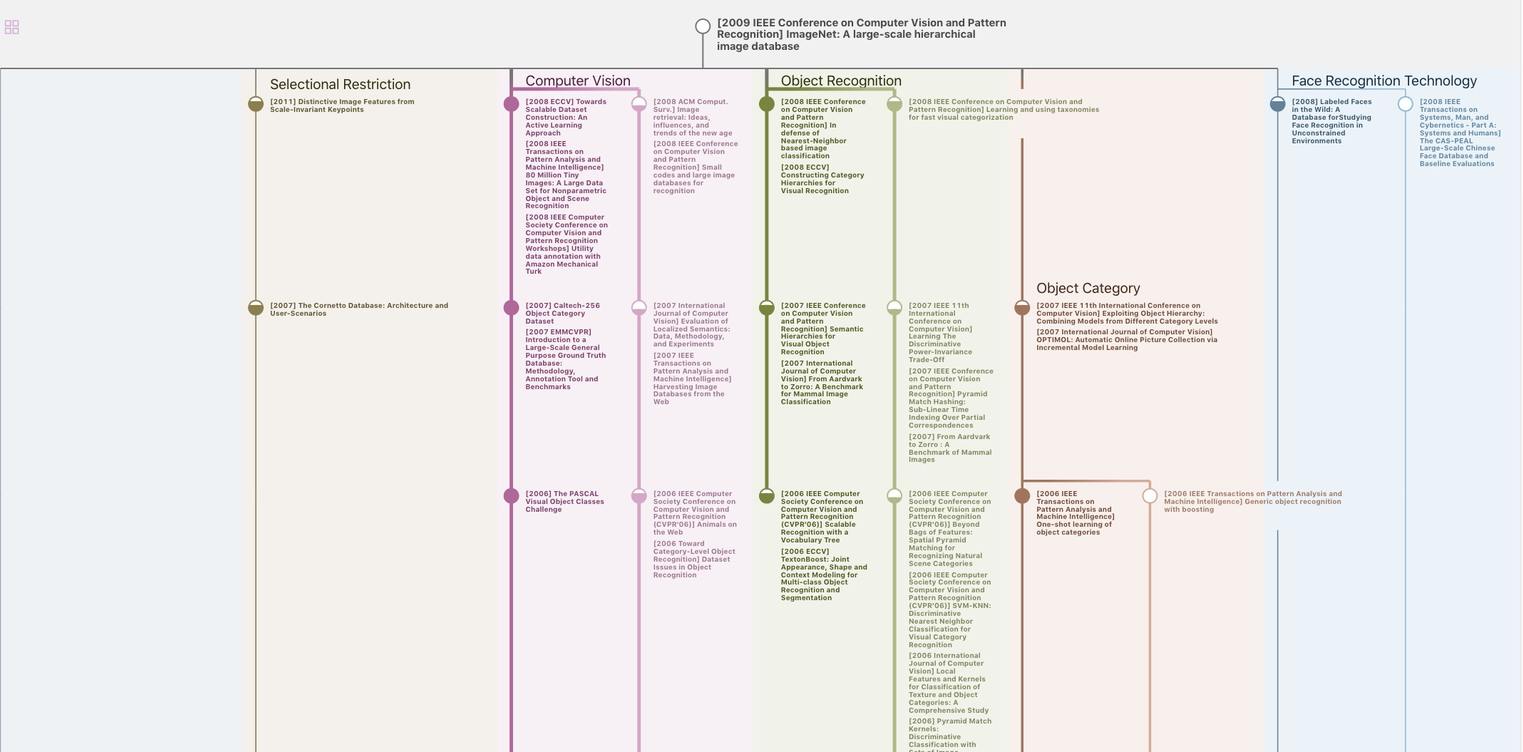
生成溯源树,研究论文发展脉络
Chat Paper
正在生成论文摘要