Improving the detection of noisy labels in image datasets using modified Confidence Learning
2022 26th International Conference on Methods and Models in Automation and Robotics (MMAR)(2022)
摘要
The effectiveness of machine learning algorithms, including deep neural networks (DNN) for classifying image data, depends on proper preparation of the training dataset. Erroneously labeled images in the training data will degrade algorithmic efficiency and cause unpredictable model behavior, thus reduce its safety. Verifying labels in the numerous available databases remains a complicated and laborious task. In this article, we present a MultiNET approach that allows for efficient verification of labeled image datasets. We adapt a state-of-the-art technique, namely Confidence Learning, extending its flexibility and improving the effectiveness by combining outcomes from various DNN architectures. Thanks to the proposed modification, it is possible to automatically detect incorrect labels while minimizing the number of false positives, thus making the verification process much less burdensome. The technique may be of use for researchers and software engineers dealing with externally supplied image datasets.
更多查看译文
关键词
label noise,database verification,confident learning
AI 理解论文
溯源树
样例
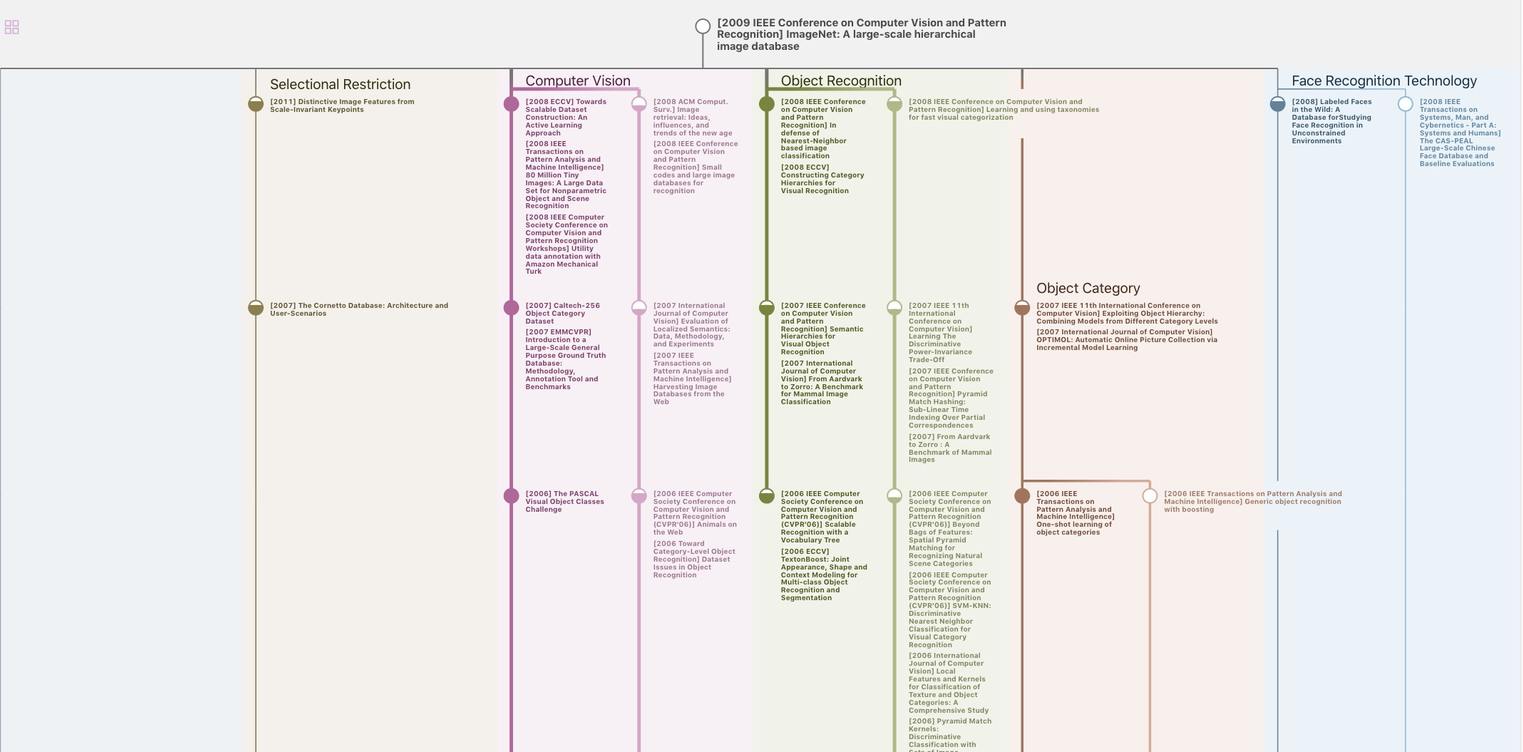
生成溯源树,研究论文发展脉络
Chat Paper
正在生成论文摘要