Non-Contact Human Activity Classification using DCNN based on UWB Radar
2019 IEEE MTT-S International Microwave Biomedical Conference (IMBioC)(2019)
摘要
Deep convolutional neural networks (DCNN) is applied in non-contact human activity classification based on ultra-wideband (UWB) radar system. A weighted time-range-frequency transform (WRTFT) method was used to get the spectrograms combining time, range and frequency information from human activity signals. Then DCNN is utilized to extract features and classification boundaries from spectrograms. Extensive experiments were conducted to compare the classification performance between the physical empirical feature method and DCNN method. DCNN method can achieve a 92.8% classification accuracy for classifying six typical human activities and show a good robustness facing individual diversity.
更多查看译文
关键词
noncontact human activity classification,UWB radar,weighted time-range-frequency transform method,frequency information,human activity signals,physical empirical feature method,DCNN method,deep convolutional neural networks,ultrawideband radar system,features extraction,classification boundaries extraction,spectrograms
AI 理解论文
溯源树
样例
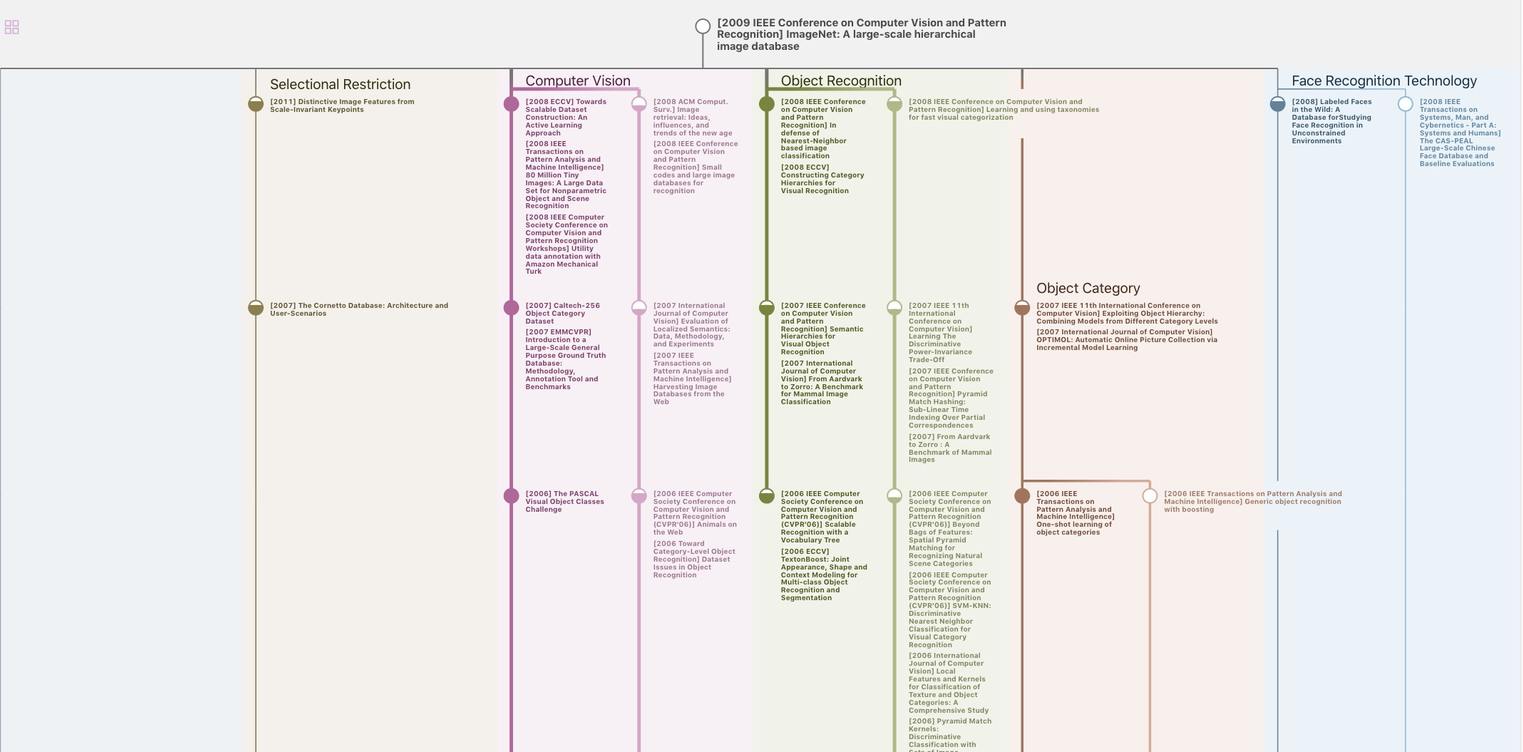
生成溯源树,研究论文发展脉络
Chat Paper
正在生成论文摘要