Fast Cuffless Blood Pressure Classification with ECG and PPG signals using CNN-LSTM Models in Emergency Medicine
2022 45th Jubilee International Convention on Information, Communication and Electronic Technology (MIPRO)(2022)
摘要
Cuffless blood pressure (BP) measurement is gaining a lot of attention as a promising new technology that can be embedded in a patch-like biosensor device. Electrocardiogram (ECG) and photoplethysmogram (PPG) waveforms are non-invasive by their nature - they can be recorded without sending any electrical impulses to the human body. These signals present different aspects of the cardiovascular system, thus using both of the signals for blood pressure classification seems like a viable strategy. Quick estimation of the blood pressure during the triage process in cases of natural disasters with many injured subjects, is an essential measure for following the hemostability of the injured. The main goal of this study is to develop a two-class classification model (Hypotension and Nothypotension) for fast prediction of the blood pressure category by utilizing ECG and PPG signals, in order to detect a BP sudden drop. The developed deep learning models are based on the LSTM architecture and its variants, CNN-LSTM. We also conducted three class classification model. The models were trained and tested using the data from the UCI Machine Learning Repository Cuff-Less Blood Pressure Estimation dataset with 12000 instances. The best result in the two-class model is AUROC = 0.74.
更多查看译文
关键词
blood pressure (BP) estimation,triage,electro-cardiogram (ECG),photoplethysmogram (PPG),long short term memory (LSTM),CNN-LSTM,artificial neural network,deep learning
AI 理解论文
溯源树
样例
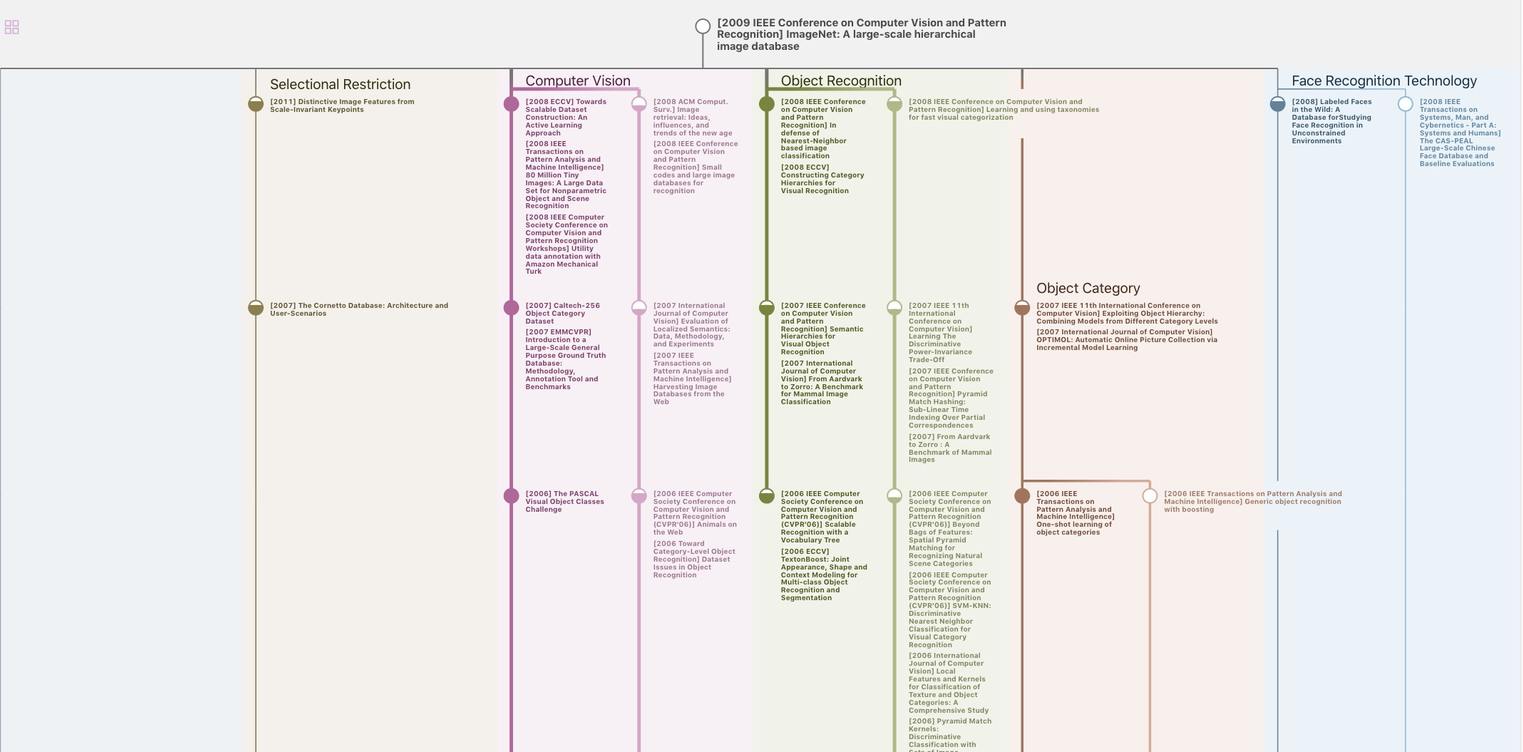
生成溯源树,研究论文发展脉络
Chat Paper
正在生成论文摘要