Wavelet-Based CNN for Robust and High-Capacity Image Watermarking
2022 IEEE International Conference on Multimedia and Expo (ICME)(2022)
摘要
“Physical” watermarking, i.e., print-scanning, print-camera, and screen-shooting resilient watermarking, has drawn great attention these years. Recent studies show that watermarking with deep networks, e.g., StegaStamp, is particularly suitable for these tasks with strong robustness against the attacks from “physical transmissions” in the wild, although it usually has a relatively small amount of embedding capacity. Recognizing the conventional CNNs are vulnerable to input noise inter-ruptions, the wavelet-based CNNs are adopted in our work by replacing their down-sampling (pooling) and up-sampling layers with Discrete Wavelet Transform (DWT) and Inverse Discrete Wavelet Transform (IDWT), respectively, to learn the stable feature representation from noise-corrupted sam-ples. A new residual regularization loss function incorporating the texture complexity is also proposed to significantly improve the visual quality of watermarked images. Exper-imental results show that the proposed wavelet-based CNN model significantly outperforms the state-of-the-art StegaS-tamp in terms of embedding capacity, imperceptibility, and robustness.
更多查看译文
关键词
Data hiding,Watermarking,Neural net-work,Discrete Wavelet Transform
AI 理解论文
溯源树
样例
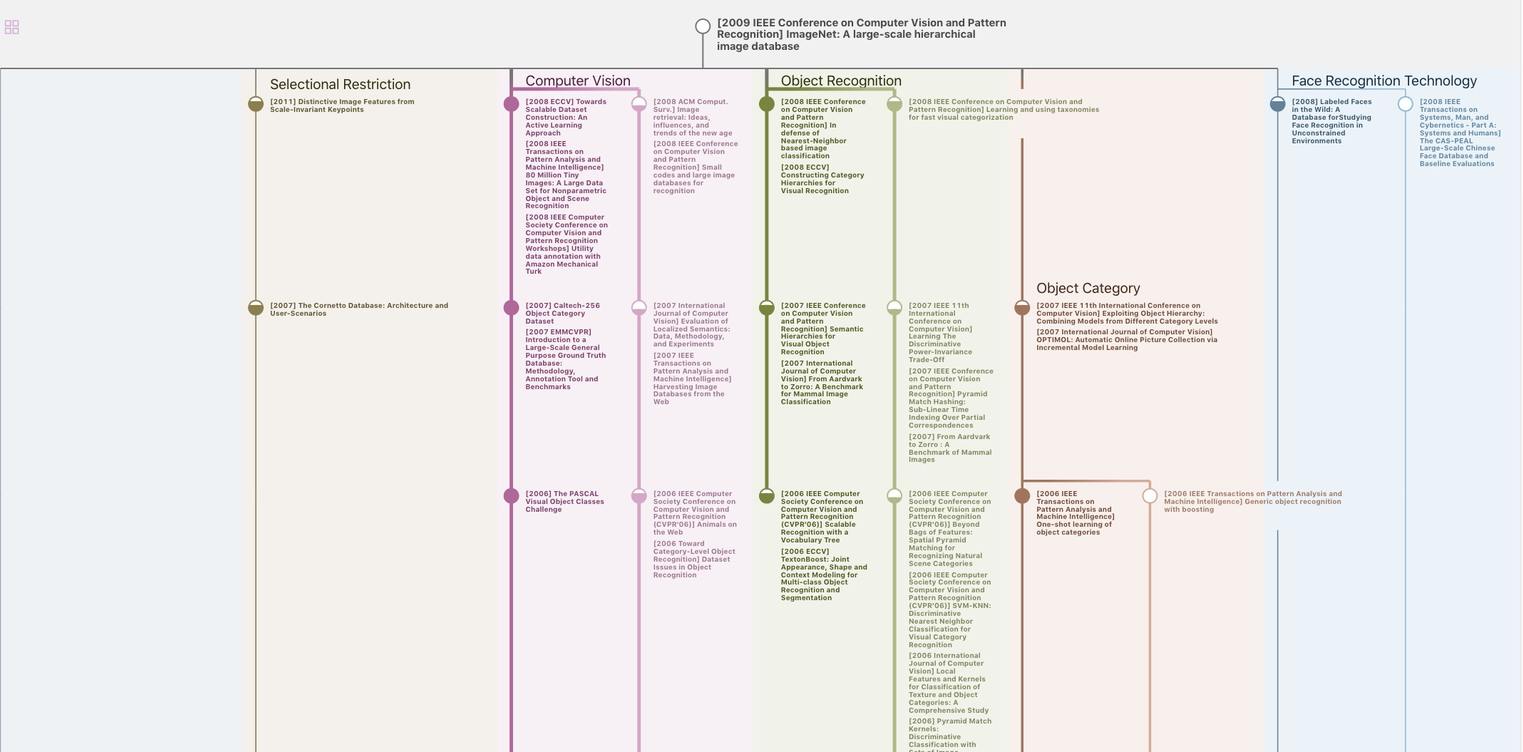
生成溯源树,研究论文发展脉络
Chat Paper
正在生成论文摘要