No-Reference Light Field Image Quality Assessment Method Based on a Long-Short Term Memory Neural Network
2022 IEEE International Conference on Multimedia and Expo Workshops (ICMEW)(2022)
摘要
Light Field (LF) cameras capture angular and spatial information and, consequently, require a large amount of resources in memory and bandwidth. To reduce these requirements, LF contents generally need to undergo compression and transmission protocols. Since these techniques may introduce distortions, the design of Light-Field Image Quality Assessment (LFI-IQA) methods are important to monitor the quality of the LFI content at the user side. In this work, we present a No-Reference (NR) LFIIQA method that is based on a Long Short-Term Memory based Deep Neural Network (LSTM-DNN). The method is composed of two streams. The first stream extracts long-term dependent distortion related features from horizontal epipolar plane images, while the second stream processes bottleneck features of micro-lens images. The outputs of both streams are fused, and supplied to a regression operation that generates a scalar value as a predicted quality score. Results show that the proposed method is robust and accurate, outperforming several state-of-the-art LF-IQA methods.
更多查看译文
关键词
Light Field Image Quality Assessment,Epipolar Plane Images,Convolution Neural Network,Transfer Learning,Long Short-TermMemory Network
AI 理解论文
溯源树
样例
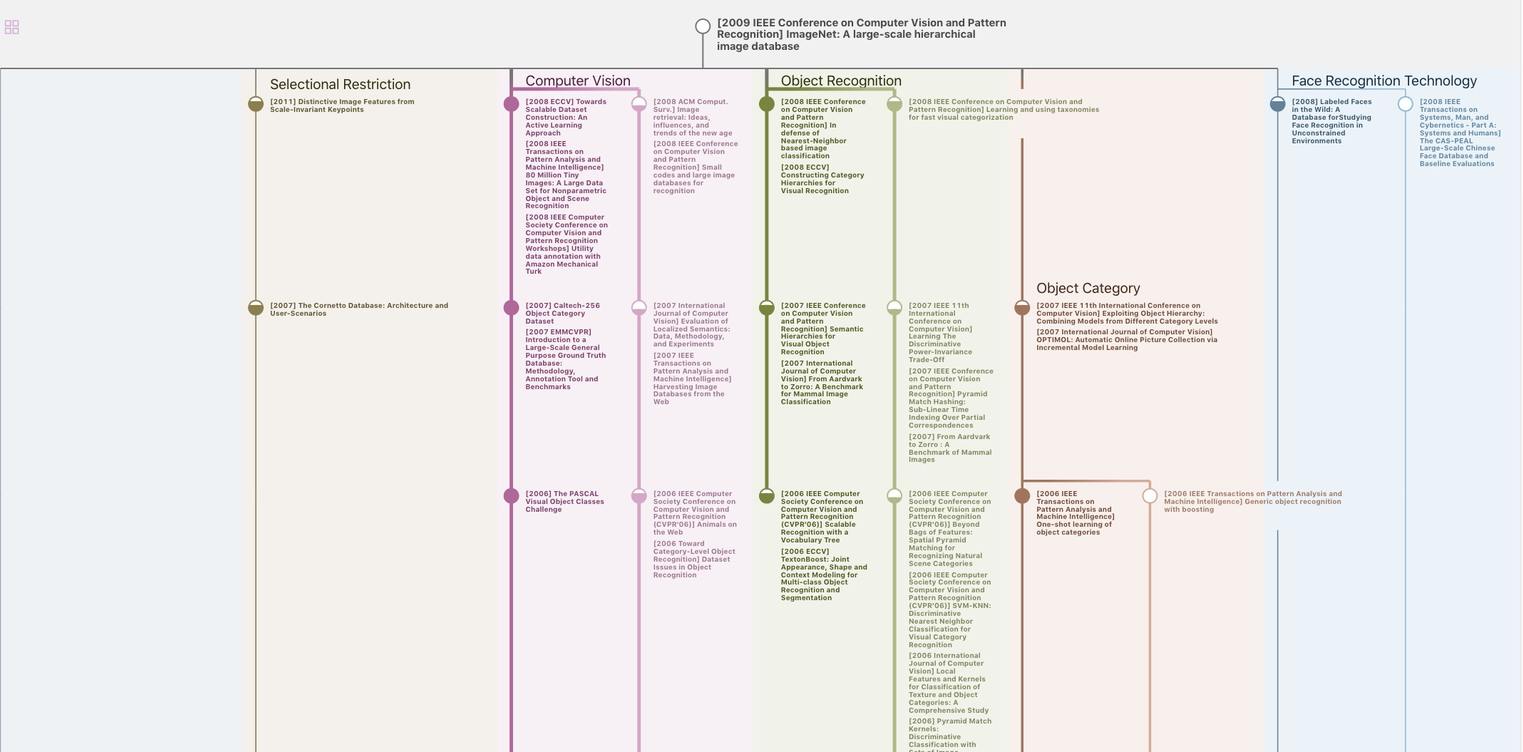
生成溯源树,研究论文发展脉络
Chat Paper
正在生成论文摘要