Video Object Segmentation with Online Mask Refinement
2022 IEEE International Conference on Multimedia and Expo Workshops (ICMEW)(2022)
摘要
This paper proposes a simple and effective video object instance segmentation method without fine-tuning named Mask Refinement Module(MRM). Many papers settle a labeling problem aiming to separate foreground objects, but most of them require training their networks again on target data. In a real scenario, it is not easy to collect dataset on the target environment and to label them as well due to security policies or a cost problem, especially for industry. We solve the problem by reshaping object masks with a video based online-learning method that enables us to adapt various changes frame by frame. In extensive experiments, results show that our approach is highly effective compared to modern methods by up to 13.9% improving of F-measure on large video surveillance dataset such as CDNet (118K images).
更多查看译文
关键词
CDNet,security policies,MRM,mask refinement module,video surveillance dataset,online-learning method,object masks,cost problem,target environment,foreground objects,labeling problem,effective video object instance segmentation method,simple video object instance segmentation method,online mask refinement,video object segmentation
AI 理解论文
溯源树
样例
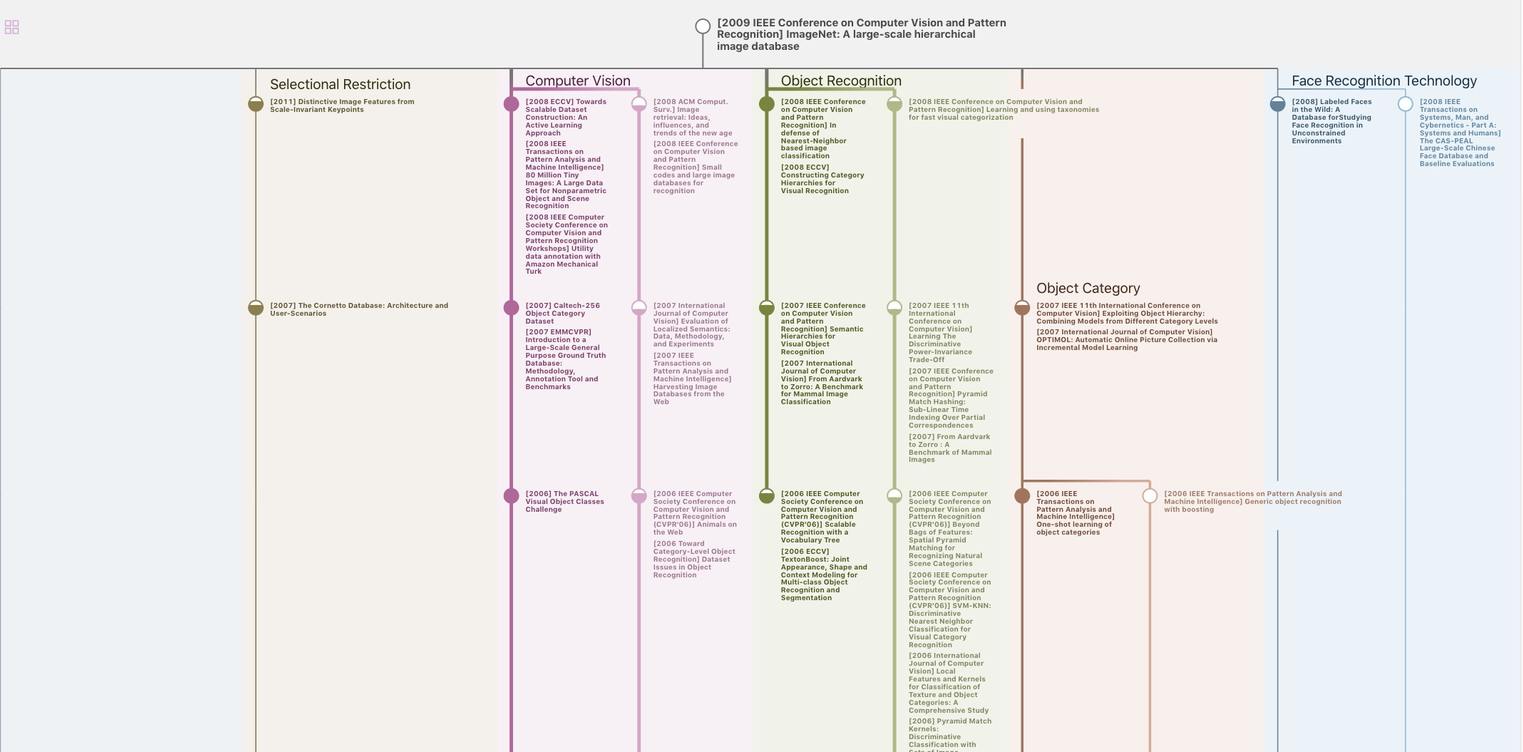
生成溯源树,研究论文发展脉络
Chat Paper
正在生成论文摘要