Fusing multi-season UAS images with convolutional neural networks to map tree species in Amazonian forests
Ecological Informatics(2022)
摘要
Remote sensing images obtained by unoccupied aircraft systems (UAS) across different seasons enabled capturing of species-specific phenological patterns of tropical trees. The application of UAS multi-season images to classify tropical tree species is still poorly understood. In this study, we used RGB images from different seasons obtained by a low-cost UAS and convolutional neural networks (CNNs) to map tree species in an Amazonian forest. Individual tree crowns (ITC) were outlined in the UAS images and identified to the species level using forest inventory data. The CNN model was trained with images obtained in February, May, August, and November. The classification accuracy in the rainy season (November and February) was higher than in the dry season (May and August). Fusing images from multiple seasons improved the average accuracy of tree species classification by up to 21.1 percentage points, reaching 90.5%. The CNN model can learn species-specific phenological characteristics that impact the classification accuracy, such as leaf fall in the dry season, which highlights its potential to discriminate species in various conditions. We produced high-quality individual tree crown maps of the species using a post-processing procedure. The combination of multi-season UAS images and CNNs has the potential to map tree species in the Amazon, providing valuable insights for forest management and conservation initiatives.
更多查看译文
关键词
Individual tree crowns,Deep learning,Multi-temporal images,Phenology,Tropical forest
AI 理解论文
溯源树
样例
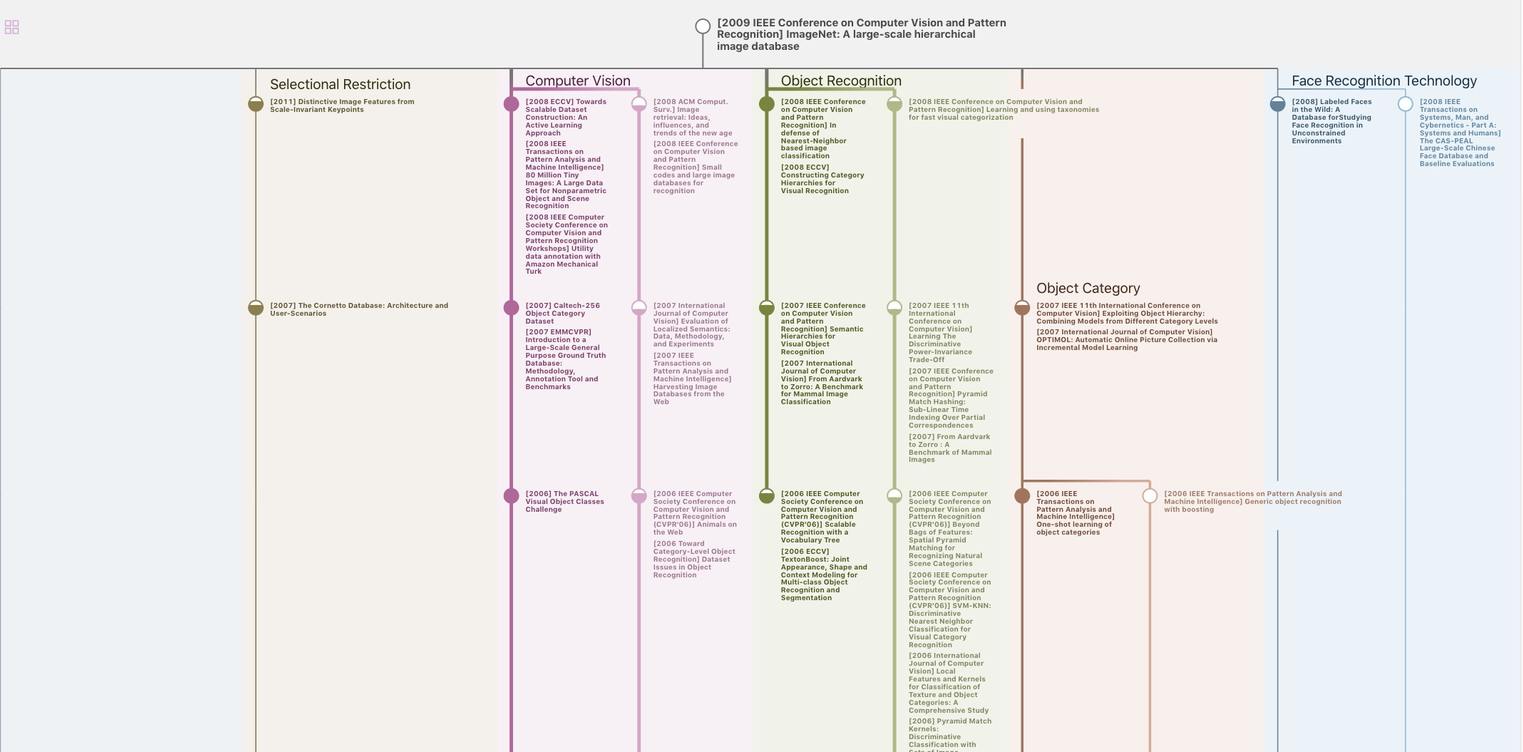
生成溯源树,研究论文发展脉络
Chat Paper
正在生成论文摘要