Towards Interpretable Anomaly Detection: Unsupervised Deep Neural Network Approach using Feedback Loop
NOMS 2022-2022 IEEE/IFIP Network Operations and Management Symposium(2022)
摘要
As telecom networks generate high-dimensional data, it becomes important to support large numbers of co-existing network attributes and to provide an interpretable and eXplainable Artificial Intelligence (XAI) anomaly detection system. Most state-of-the-art techniques tackle the problem of detecting network anomalies with high precision but the models don’t provide an interpretable solution. This makes it hard for operators to adopt the given solutions. The proposed Cluster Characterized Autoencoder (CCA) architecture improves model interpretability by designing an end-to-end data driven AI-based framework. Candidate anomalies identified using the feature optimised Autoencoder and entropy based feature ranking are clustered in reconstruction error space using subspace clustering. This clustering is seen to separate true positives and false positives and how well this is done is evaluated using entropy and information gain. A two dimensional t-SNE representation of anomaly clusters is used as a graphical interface to the analysis and explanation of individual anomalies using SHAP values. The solution provided by this unsupervised approach helps the analyst in the categorisation, identification and feature explanation of anomalies providing faster root cause analysis. Therefore, our solution provides better support for the network domain analysts with an interpretable and explainable Artificial Intelligence (AI) anomaly detection system. Experiments on a real-world telecom network dataset demonstrate the efficacy of our proposed algorithm.
更多查看译文
关键词
Neural Network,eXplainable AI,Group Anomaly Detection
AI 理解论文
溯源树
样例
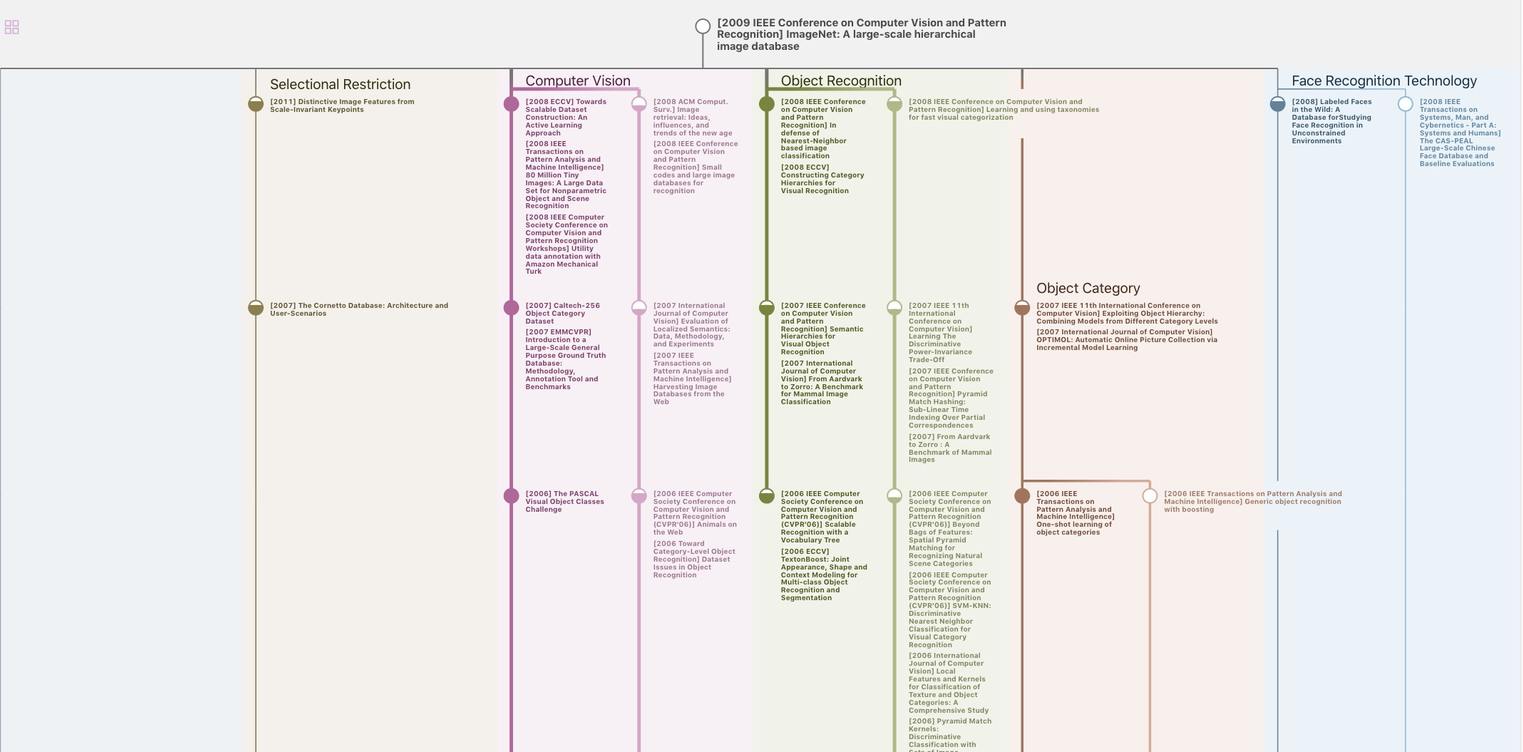
生成溯源树,研究论文发展脉络
Chat Paper
正在生成论文摘要