Learning Traffic Encoding Matrices for Delay-Aware Traffic Engineering in SD-WANs
NOMS 2022-2022 IEEE/IFIP Network Operations and Management Symposium(2022)
摘要
This paper introduces Traffic Encoding Matrices (TEMs) as an alternative to traditional Traffic Matrices (TMs) for representing network traffic demands. While TMs only capture average demand information, TEMs are designed to capture distributional demand information by learning representations whose variations capture most of the structure of the distribution of demands. We present a practical approach based on off-the-shelf neural autoencoders to efficiently construct TEMs at the edge of the network. We then present the design and evaluation of NeuroTE, a DRL-based framework for delay-aware traffic engineering in SD-WANs using TEMs. Using real traffic traces, we present experimental results to demonstrate the advantages of using TEMs instead of TMs in traffic engineering. Our results show that, when traffic demands are dynamic, TEM-based control leads to: 1) improved network performance, and 2) faster convergence to the optimal solution compared to TM-based control using exactly the same DRL control algorithm.
更多查看译文
关键词
TM,TEM-based control,delay-aware traffic engineering,SD-WAN,network traffic demands,distributional demand information,learning traffic encoding matrices,NeuroTE,DRL control algorithm
AI 理解论文
溯源树
样例
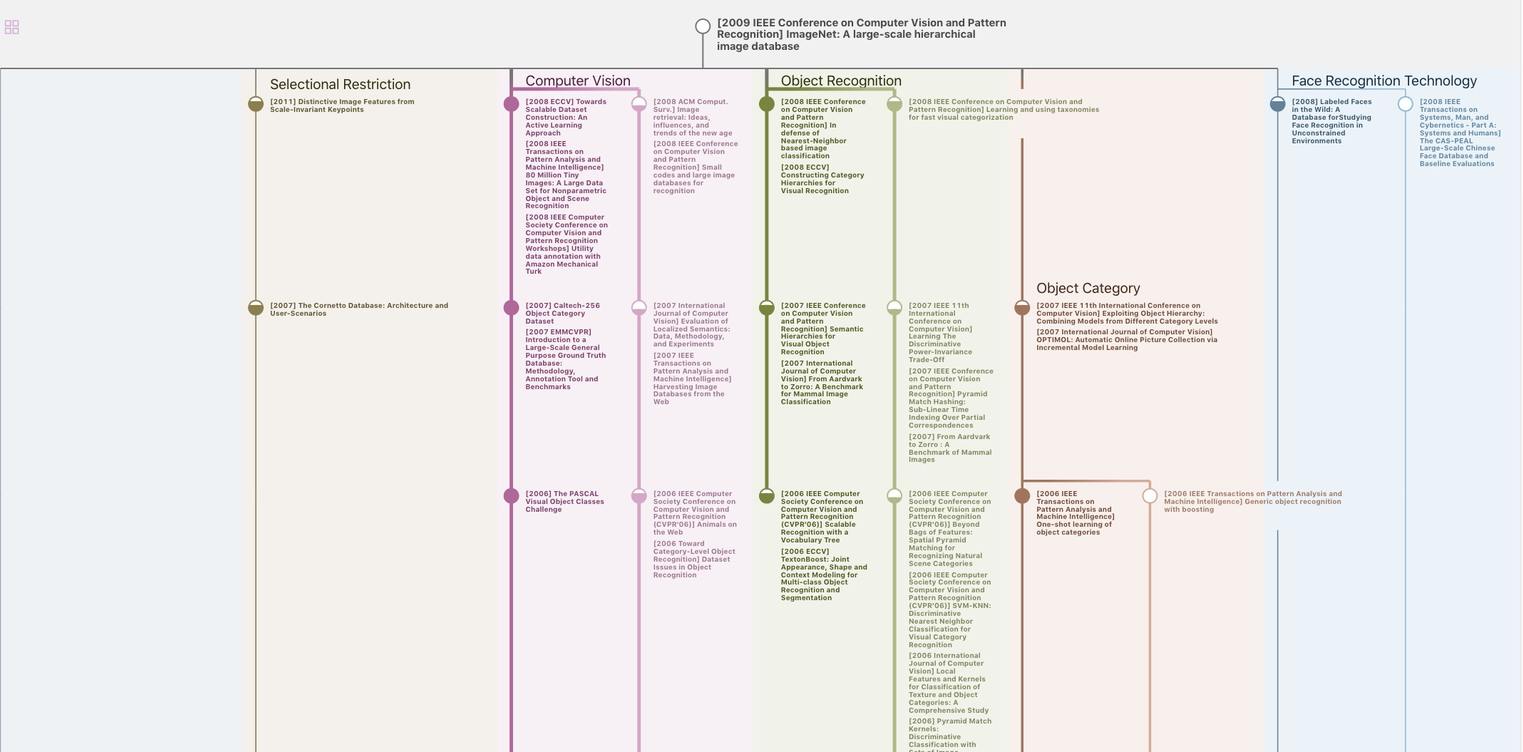
生成溯源树,研究论文发展脉络
Chat Paper
正在生成论文摘要