A Learning Framework with Disposable Auxiliary Networks for Early Prediction of Product Success
WI/IAT(2021)
摘要
Consider the scenario in which an investor seeks to identify potential products before they are unveiled to the public. For such a scenario, the investor poses questions such as "What characteristic better represents a product?" or "What features make a product popular?" Unlike traditional recommendation problems, in this case, there is no user feedback for the upcoming products, which greatly complicates prediction. To address this challenging yet common scenario, we present an unconventional multi-task learning framework that trains the prediction model on information for mature products that have user feedback, and then uses this model to predict the success of upcoming products for which there is no user feedback. To achieve this goal, we train a main task network to extract product features from their descriptions while at the same time training a disposable auxiliary network to learn domain-specific words and popular trends from user reviews.This disposable auxiliary network is beneficial during the training of the main task network but is unused at the inference stage. Empirical results on two real-world datasets in different languages demonstrate that the proposed framework not only improves the overall rating prediction for products but also identifies the top successful products without any user reviews.
更多查看译文
关键词
disposable auxiliary networks, textual information, blockbuster prediction
AI 理解论文
溯源树
样例
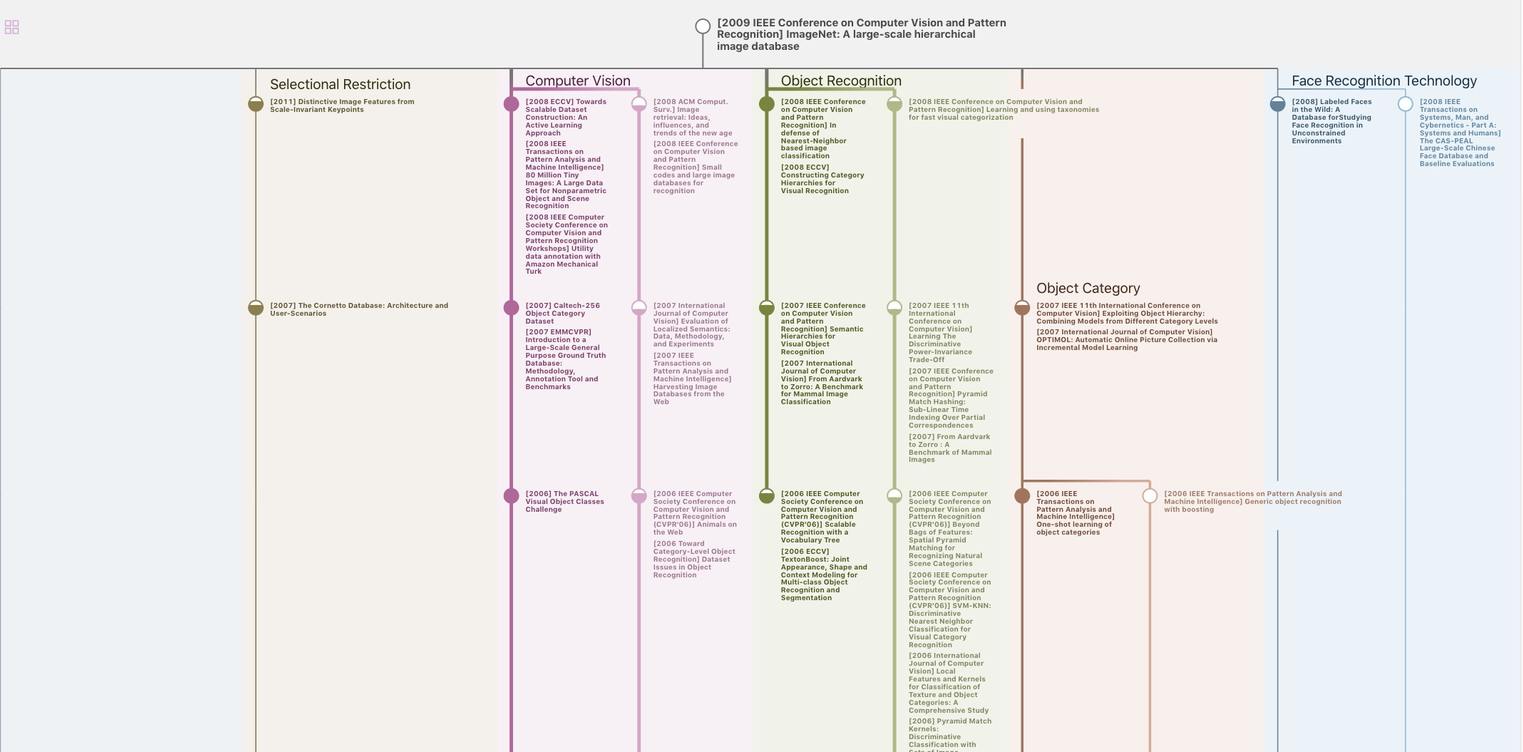
生成溯源树,研究论文发展脉络
Chat Paper
正在生成论文摘要